JointNet: A multimodal deep-learning-based approach for joint inversion of Rayleigh wave dispersion and ellipticity
crossref(2024)
摘要
Joint inversion of multiple datasets is an effective approach for high-precision imaging of the crustal and upper mantle velocity structures. In this study, we propose a novel deep learning-based method called JointNet for jointly inverting Rayleigh wave phase velocity and ellipticity data to obtain high-precision shear wave velocity models. JointNet, a multimodal deep neural network, is designed to analyze these independent physical parameters and generate outputs that include a velocity model and layer thicknesses. The network is trained using a large dataset of randomly generated 1D models along with their corresponding calculated phase velocities and ellipticities. Our tests using synthetic and observed data demonstrate that JointNet produces inversion results that are highly comparable to those obtained through a Markov Chain Monte Carlo-based method. This indicates that the network effectively captures the nonlinear relationship between phase velocity, ellipticity data, and the 1D Vs model. In addition, JointNet eliminates the need for prior information input and significantly reduces the computational time for inversion compared to traditional nonlinear methods. Training using synthetic data based on a global model ensures its wide applicability in various regions with different velocity structures. Furthermore, JointNet can be readily adapted to incorporate additional datasets, such as receiver functions, to further enhance imaging resolution. Essentially, JointNet can also function as a novel inversion framework for more extensive model inversion studies.
更多查看译文
AI 理解论文
溯源树
样例
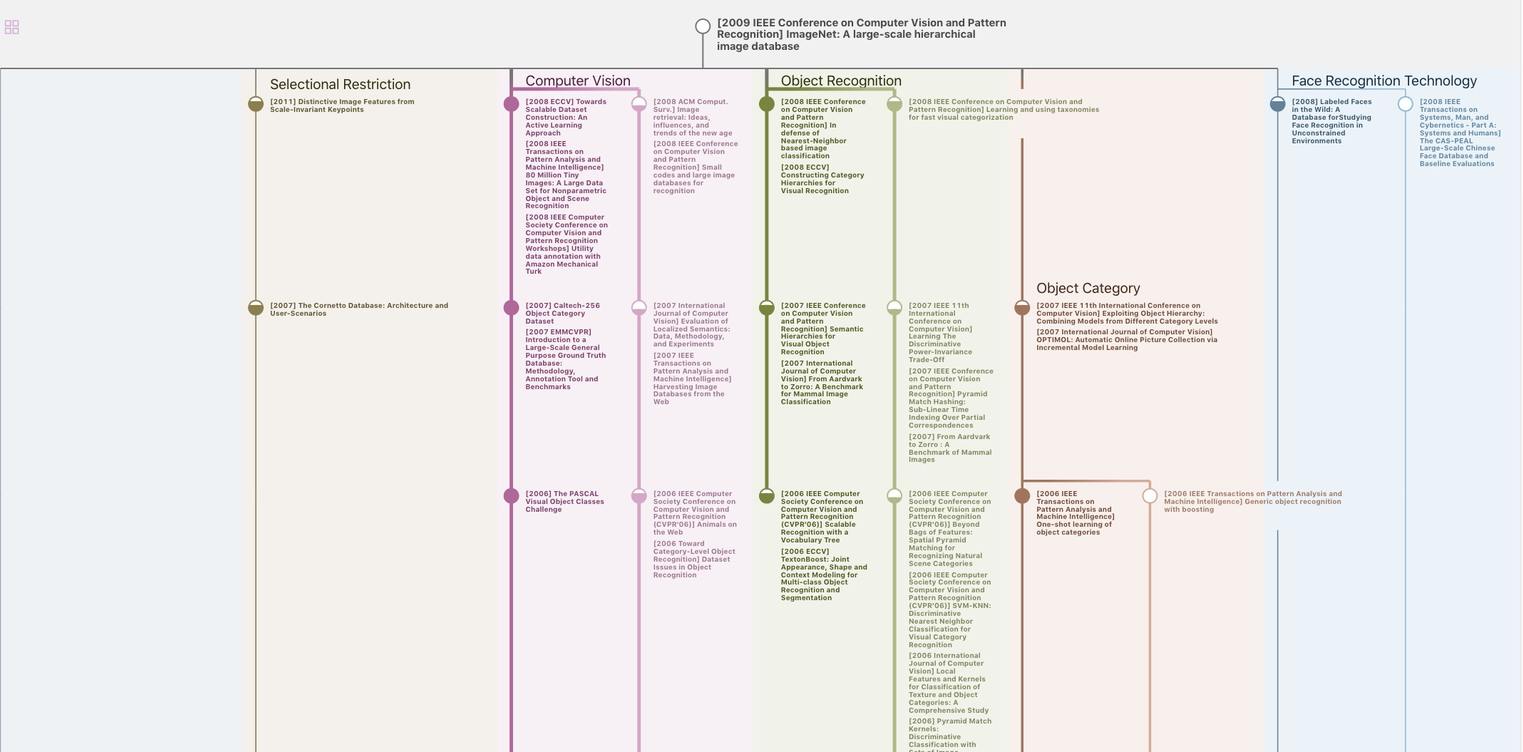
生成溯源树,研究论文发展脉络
Chat Paper
正在生成论文摘要