Machine learning of 27Al NMR quadrupolar tensors for crystalline structures from DFT
crossref(2024)
摘要
NMR crystallography has emerged as promising technique for the determination and refinement of crystal structures. The crystal structure of compounds containing quadrupolar nuclei, such as 27Al, can be improved by directly comparing solid-state NMR measurements to DFT computations of the electric field gradient (EFG). The high computational cost of these first-principles calculations limits the applicability of this method to all but the most well-defined structures. We developed a fast, low-cost machine learning model to predict EFG parameters based on local structural motifs and elemental parameters. We computed 8081 EFG tensors using DFT and benchmarked them against 105 experimental parameters. Surprisingly, simple local geometric features dominate the predictive performance of the resulting random-forest model, yielding an R2 value of 0.98 and an RMSE of 0.61 MHz. This model accuracy should enable pre-refining future structural assignments before finally validating with first-principles calculations. Such a catalogue of 27Al NMR tensors can serve as a tool for researchers assigning complex quadrupolar spectra.
更多查看译文
AI 理解论文
溯源树
样例
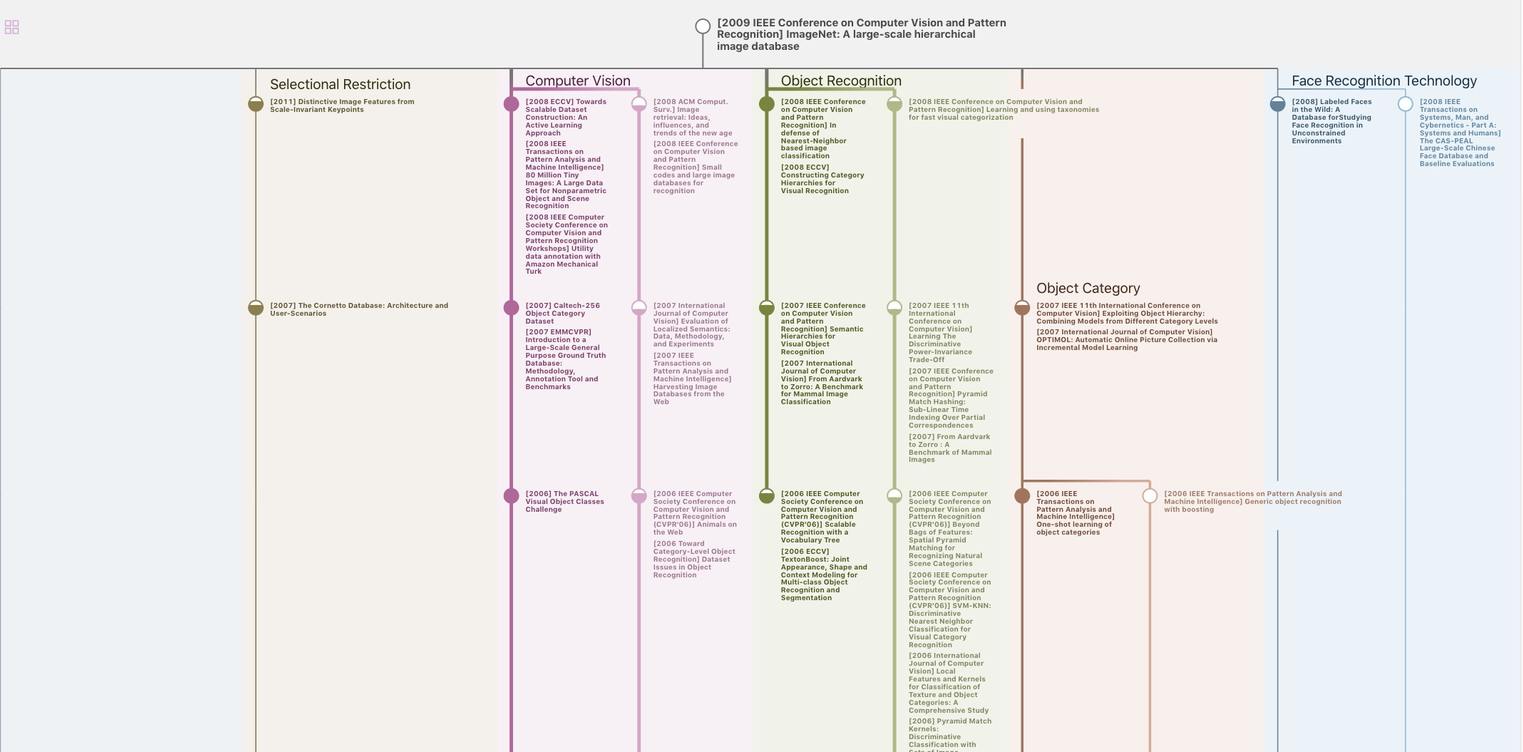
生成溯源树,研究论文发展脉络
Chat Paper
正在生成论文摘要