Skilful probabilistic forecasts of UK floods months ahead using a hybrid approach
crossref(2024)
摘要
Streamflow forecasts months ahead are an important component of flood risk management. Hybrid methods that predict seasonal streamflow quantiles using ML/AI models driven by climate model outputs are currently underexplored, yet have some important advantages over traditional approaches based on hydrological models. For example, they are computational efficient, can incorporate a wide variety of input data, and may avoid the need for spatial downscaling and/or bias correction. Here we develop a hybrid subseasonal to seasonal streamflow forecasting system to predict the monthly maximum daily streamflow up to four months ahead. We train a machine learning model on dynamical precipitation and temperature forecasts from a large ensemble from the Copernicus Climate Change Service (C3S). We show that multi-site ML models trained on pooled catchment data together with static catchment attributes are significantly more skilful compared to single-site ML models that are trained on data from each catchment individually. Overall, we find 99.8% of stations show positive skill relative to climatology in the first month after initialization, 90.7% in the second month, 57.9% in the third month and 35% in the fourth month.
更多查看译文
AI 理解论文
溯源树
样例
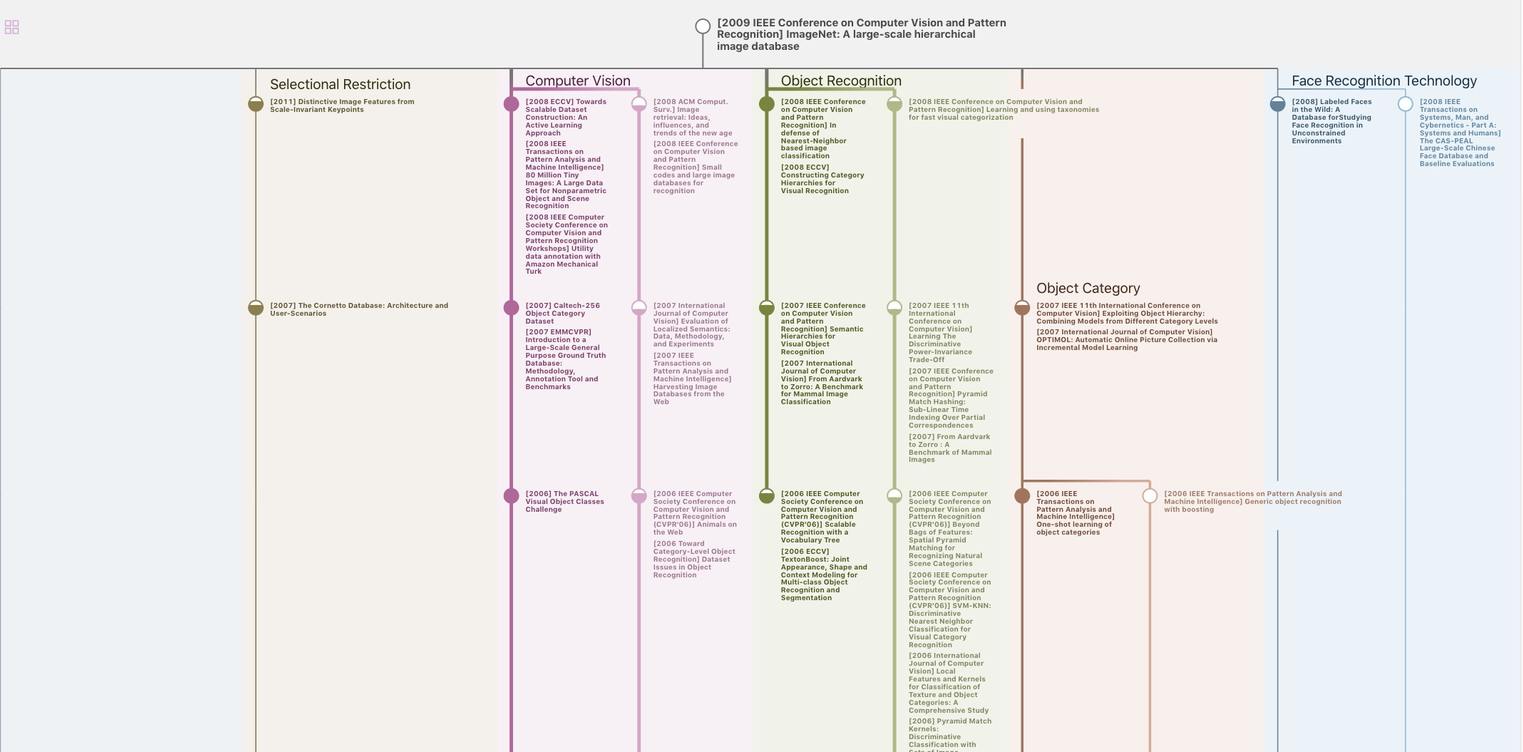
生成溯源树,研究论文发展脉络
Chat Paper
正在生成论文摘要