Towards CO2 emission estimation in urban areas using a dense sensor network and the high-resolution GRAMM/GRAL model
crossref(2024)
摘要
Urban areas are responsible for about 70% of anthropogenic CO2 emissions and are therefore an important system in which to develop mitigation strategies to reduce emissions. To assess these strategies and monitor mitigation efforts, independent knowledge of urban CO2 sources is required. A measurement-based estimation of emissions can be obtained using CO2 measurements, along with prior information on emissions from inventories and a high-resolution transport model. Here we use the forward model system GRAMM/GRAL. This consists of two nested models, a prognostic mesoscale model (GRAMM), and a microscale computational fluid dynamics and Lagrangian dispersion model (GRAL). We run GRAL on a 15 km x 15 km grid over the city of Oakland, California, at a horizontal resolution of 10 m x 10 m. This resolution of 10 meters is sufficient to resolve street canyon effects. We utilize the Berkeley Atmospheric CO2 Observation Network (BEACO2N), a unique high-density network of CO2 monitoring stations consisting of mid-cost sensors. To optimize computational time, GRAMM/GRAL is run in a steady-state mode where we compute hourly steady-state wind and concentration fields, corresponding to different synoptic meteorological situations. To infer the temporal evolution of the simulated CO2 concentration over a whole year we then use a match-to-observation algorithm that for each hour chooses the hourly steady-state wind field which minimizes the difference between the simulated wind and the observed wind time series from an urban network of wind measuring stations (May et al. 2024). In our study, we assess the performance of the GRAMM/GRAL model in Oakland and compare the modelled and measured wind and concentration fields over a year. In general, we find a good agreement between modelled and observed wind fields. Comparing the time series of simulated CO2 concentration to the observed CO2 concentration from the BEACO2N network, we analyze the agreement and difference between the modelled and simulated CO2 concentration and propose possible improvements in the modelling framework. Finally, we propose an inversion set-up to infer emission estimates at high resolution given the observations and discuss remaining challenges and limitations.
更多查看译文
AI 理解论文
溯源树
样例
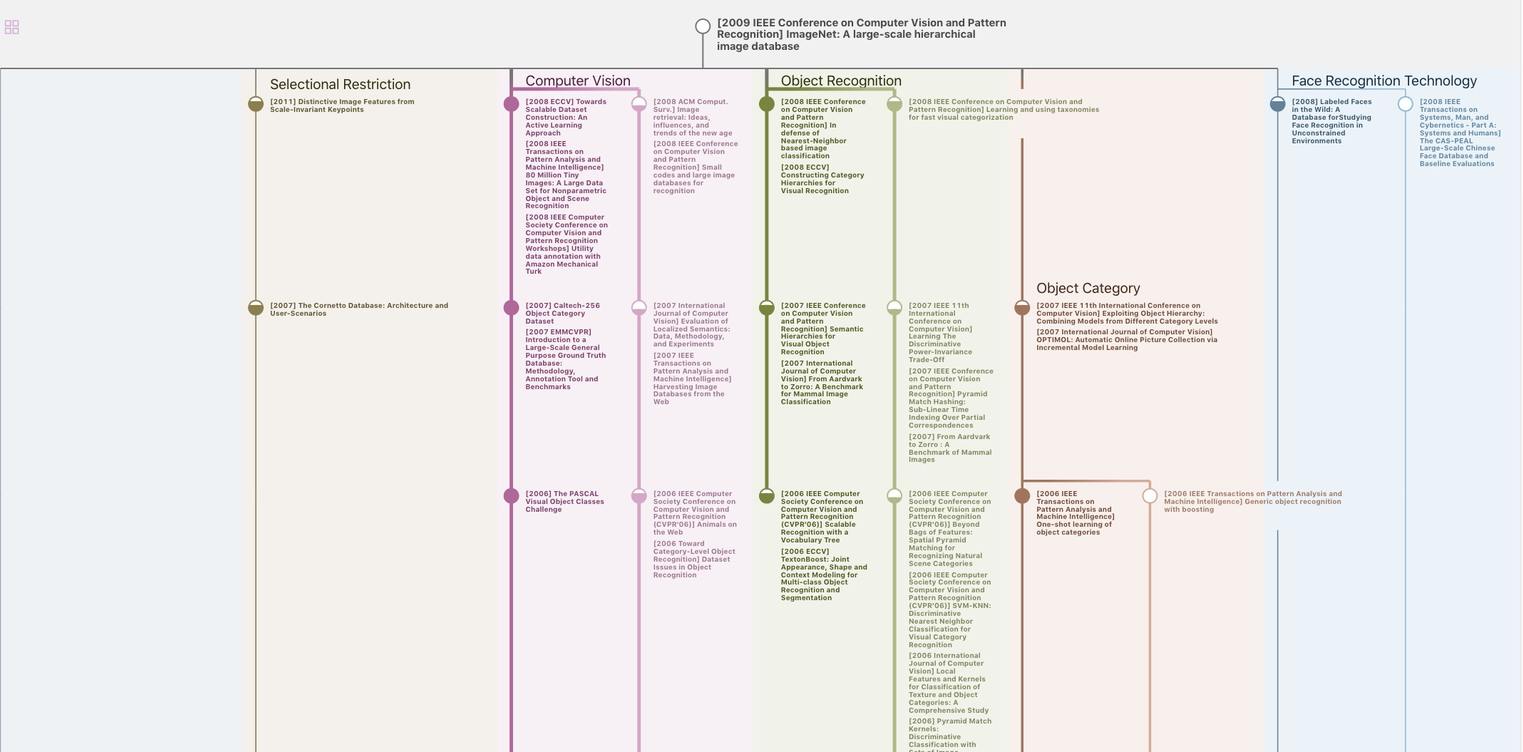
生成溯源树,研究论文发展脉络
Chat Paper
正在生成论文摘要