A MapReduce-Based Distributed SVM for Scalable Data Type Classification.
Lecture Notes of the Institute for Computer Sciences, Social Informatics, and Telecommunications Engineering(2016)
摘要
Data type classification is a significant problem in digital forensics and information security field. Methods based on support vector machine have proven the most successful across varying classification approaches in the previous work. However, the training process of SVM is notably computationally intensive with the number of training vectors increased rapidly. In this study, we proposed parallel distributed SVM (PDSVM) based on Hadoop MapReduce for scalable data type classification. First the map phase determines support vectors (SVs) in the splits of dataset by running the sequential minimal optimization. Then the reduce phase merges SVs and computes the degree of global convergence. Finally, PDSVM utilizes the global convergence SVs to get SVM model. The experimental results demonstrate that PDSVM can not only process large scale training dataset, but also perform well in the term of classification accuracy.
更多查看译文
关键词
Data type classification,Digital forensics,Support vector machine,Distributed,MapReduce
AI 理解论文
溯源树
样例
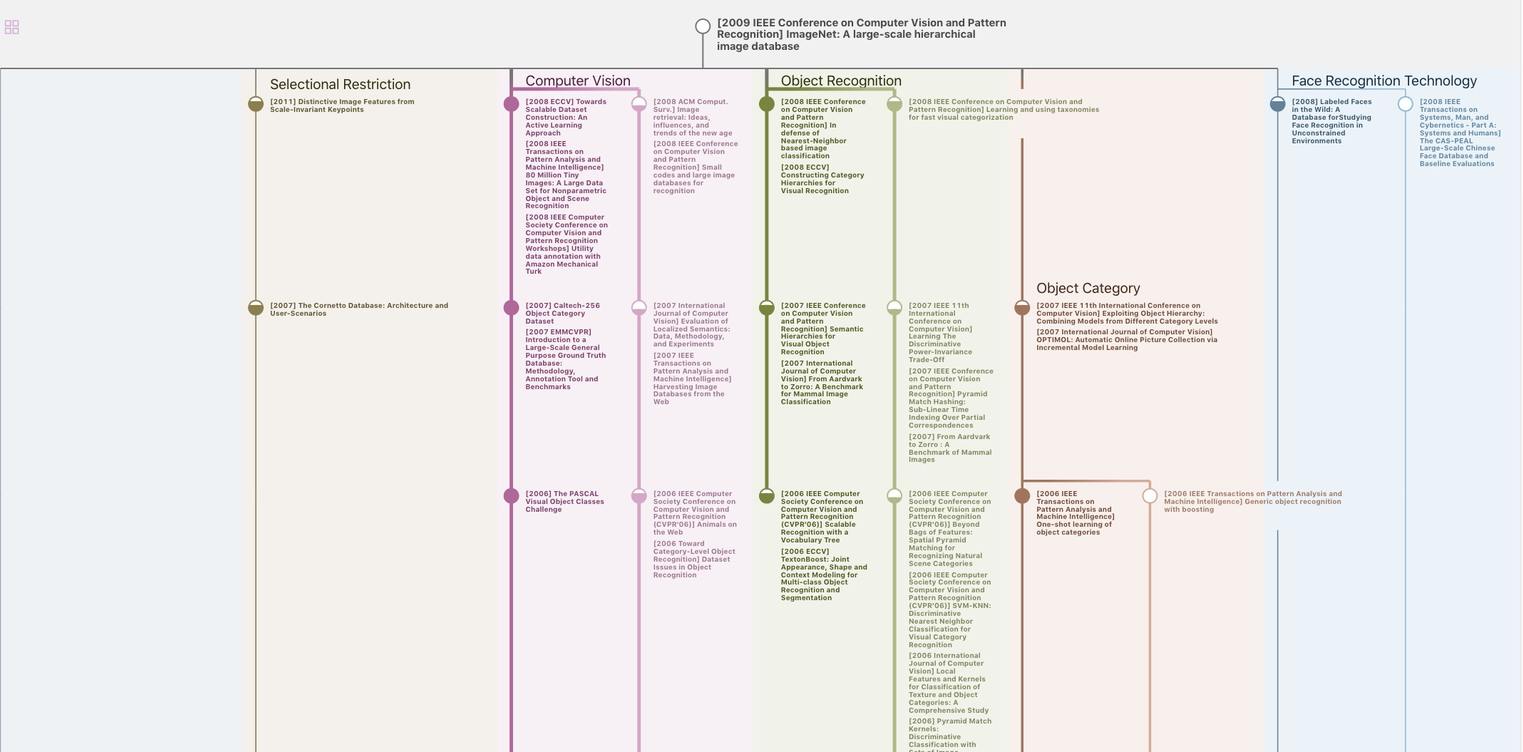
生成溯源树,研究论文发展脉络
Chat Paper
正在生成论文摘要