Towards seamless rainfall and flood forecasting in the Netherlands: improvements to and validation of blending in pysteps
crossref(2024)
摘要
Flood early warning in fast responding catchments challenges our forecasting systems. It requires frequently updated, accurate and high-resolution rainfall forecasts to provide timely warning of rainfall amounts that will reach a catchment in the coming hours. The Netherlands is a typical example, with polder systems below sea level, a high level of urbanization and catchments with short response times. The need for better short-term rainfall forecasts is clearly present, but this is generally not feasible with numerical weather prediction (NWP) models alone. Hence, an alternative rainfall forecasting method is desirable for the first few hours into the future.Rainfall nowcasting can provide this alternative but quickly loses skill after the first few hours. A promising way forward is a seamless forecasting system, which tries to optimally combine rainfall products from nowcasting and NWP. In this study, we applied the STEPS blending method to combine rainfall forecasts from ensemble radar nowcasts with those from the Harmonie-AROME configuration of the ACCORD NWP model in the Netherlands. This blending method is part of the open-source nowcasting initiative pysteps. To make blending possible in an operational setup, including the needs of involved water authorities, we made several adjustments to the blending implementation in pysteps, for instance:We reduced the computational time by using a faster preprocessing and advection scheme. We improved the noise initialization (needed for generating ensemble members) to allow for stable forecasts, also when one or both product(s) contain(s) no rain. We enabled a dynamic disaggregation of the 1-hour resolution NWP forecasts to match the temporal resolution of the radar nowcast. We operationalized the updated blending framework in the flood forecasting platforms of the involved water authorities. Given a forecast duration of 12 hours for the blended forecast and a 10-minute time step, average computation times are 3.4 minutes for a deterministic run and 12.3 minutes for an ensemble forecast with 10 members on a 4-core machine. Preprocessing takes approximately 10 minutes and only needs to occur when a new NWP forecast is issued. We tested the implementation for an entire, rainy summer month (July 15 to August 15, 2023) and analyzed the results over the entire domain. The results demonstrate that the blending method effectively combines radar nowcasts with NWP forecasts. Depending on the statistical score considered (such as RMSE and critical success index), the blending method performs either better or on par with the best-performing individual product (radar nowcast or NWP). A consistent finding is that the blending closely tracks the nowcast quality during the initial 1 to 2 hours of the forecast (in this study, the nowcast had lower errors than NWP during the first 2 – 2.5 hours), after which it gradually transitions into the NWP forecast. At longer lead times, the seamless product retains local precipitation structures and extremes better than the NWP product. It does this by leveraging information from the radar nowcast and the stochastic perturbations. Based on these results, a seamless forecasting approach can be regarded as an improvement for the involved water authorities.
更多查看译文
AI 理解论文
溯源树
样例
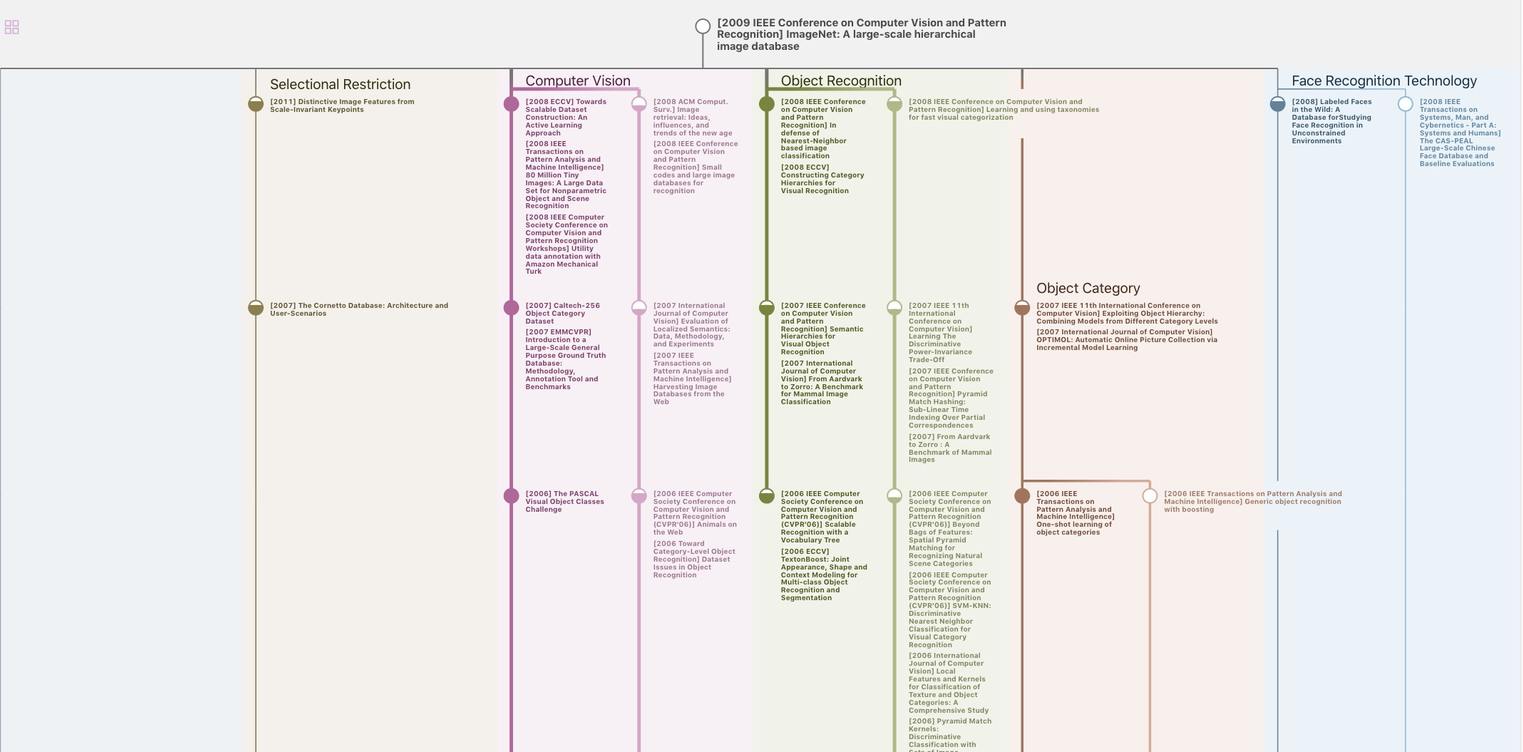
生成溯源树,研究论文发展脉络
Chat Paper
正在生成论文摘要