Reducing snow amount Uncertainty in CMIP6 Pan-Canadian Climate Projections
crossref(2024)
Abstract
Recent studies have demonstrated that the uncertainty in projections can be reduced by weighting the GCMs based on their ability to accurately reproduce historical climate conditions in specific geographical regions. This study aims to reduce the uncertainty in projections of the annual maximum snow amount from obtained from the most recent iteration of GCMs in the Coupled Model Intercomparison Project Phase 6 (CMIP6). To do so, we implement a three-phase approach in order to adapt the Climate model Weighting by Independence and Performance (ClimWIP) algorithm to the main drivers of snow-amount projections.Phase one of our research involves identifying and implementing the most effective metric combinations that yield a weighted field closely aligning with the reference dataset's state. In phase two, these optimal combinations are applied within a perfect model protocol to determine the most appropriate combination for practical application. The final phase uses the selected combination to compute weights specifically for the climate projection of the annual maximum snow amount.Our findings indicate that our approach primarily impacts regions where snow amount is a critical factor. Additionally, we observe a narrowed range of uncertainties in both the annual maximum snow amount and the 2-meter temperature projections. This study's outcomes not only demonstrate the efficacy of our approach but also offers valuable insights for future climate projection and adaptation strategies in Canada.
MoreTranslated text
AI Read Science
Must-Reading Tree
Example
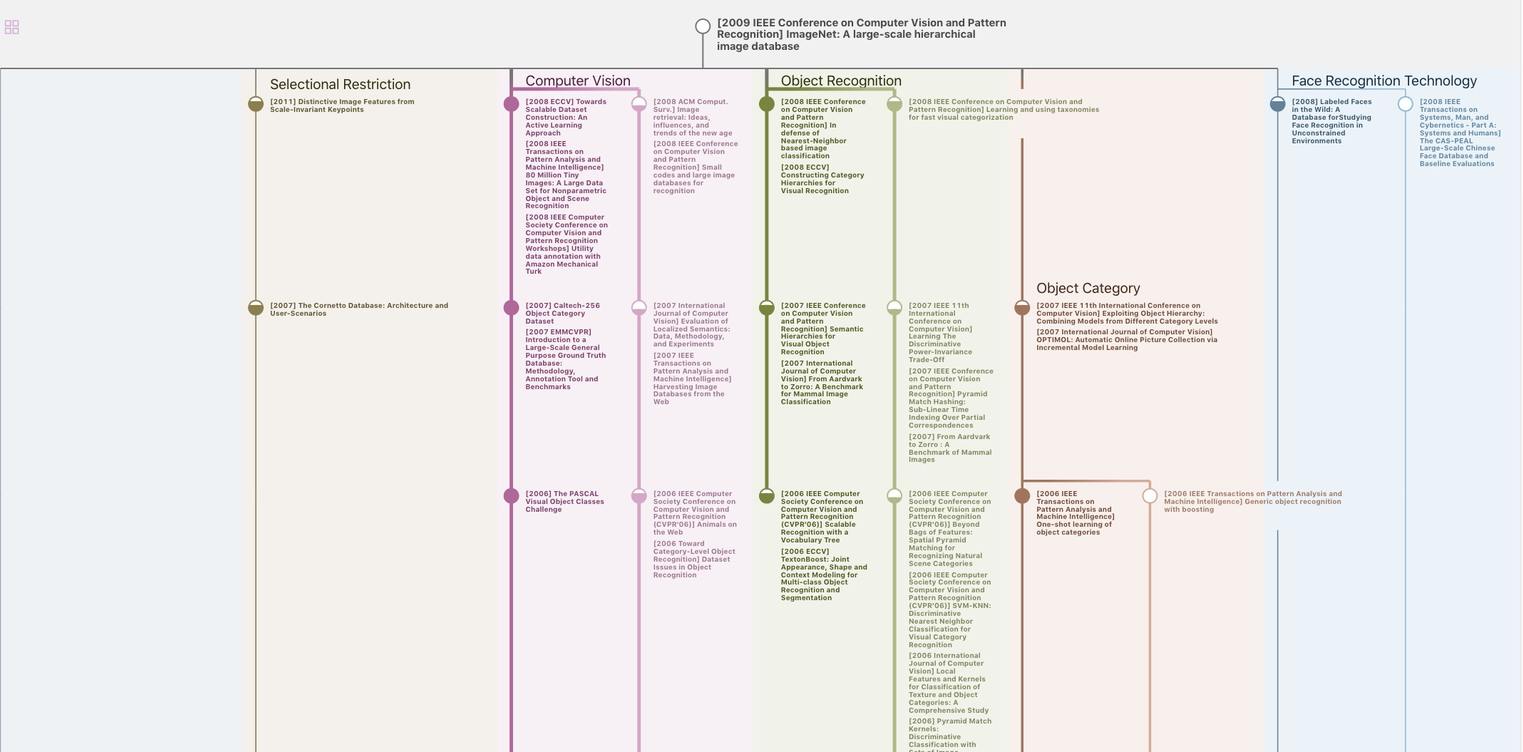
Generate MRT to find the research sequence of this paper
Chat Paper
Summary is being generated by the instructions you defined