Urban 3D Change Detection with Deep Learning: Custom Data Augmentation Techniques
crossref(2024)
摘要
The ability to detect changes occurring on the Earth's surface is essential for comprehensively monitoring and understanding evolving landscapes and environments. To achieve a comprehensive understanding, it is imperative to employ methodologies capable of efficiently capturing and analyzing both two-dimensional (2D) and three-dimensional (3D) changes across various periods. Artificial Intelligence (AI) stands out as a primary resource for investigating these alterations, and when combined with Remote sensing (RS) data, it has demonstrated superior performance compared to conventional Change Detection (CD) algorithms. The recent introduction of the MultiTask Bitemporal Images Transformer [1] (MTBIT) network has made it possible to simultaneously solve 2D and 3D CD tasks leveraging bi-temporal optical images. However, this network presents certain limitations that necessitate being considered. These constraints encompass a tendency to overfit the training distribution and challenges in inferring extreme values [1]. To address these shortcomings, this work introduces a series of custom augmentations, including strategies like Random Crop, Crop or Resize, Mix up, Gaussian Noise on the 3D CD maps, and Radiometric Transformation. Applied individually or in specific combinations, these augmentations aim to bolster MTBIT's ability to discern intricate geometries and subtle structures that are otherwise difficult to detect. Furthermore, the evaluation metrics used to assess MTBIT, such as Root Mean Squared Error (RMSE) and the change RMSE (cRMSE), have their limitations. As a response, the introduction of the true positive RMSE (tpRMSE) offers a more comprehensive evaluation, specifically focusing on MTBIT's efficacy in the 3D CD task by considering only the pixels affected by actual elevation changes. The implementation of custom augmentations particularly when applied in synergy, like Crop or Resize with Gaussian Noise on the 3D map, yielded substantial improvements. These interventions led – through the best augmentation configuration – to the reduction of the cRMSE to 5.88 meters and the tpRMSE to 5.34 meters, compared to the baseline (standard MTBIT) values of 6.33 meters and 5.60 meters, respectively. The proposed augmentations significantly bolster the practical usability and reliability of MTBIT in real-world applications, effectively addressing critical challenges within the realm of Remote Sensing CD. REFERENCES: [1] Marsocci, V., Coletta, V., Ravanelli, R., Scardapane, S., Crespi, M., 2023. Inferring 3D change detection from bitemporal optical images. ISPRS Journal of Photogrammetry and Remote Sensing, 196, 325-339
更多查看译文
AI 理解论文
溯源树
样例
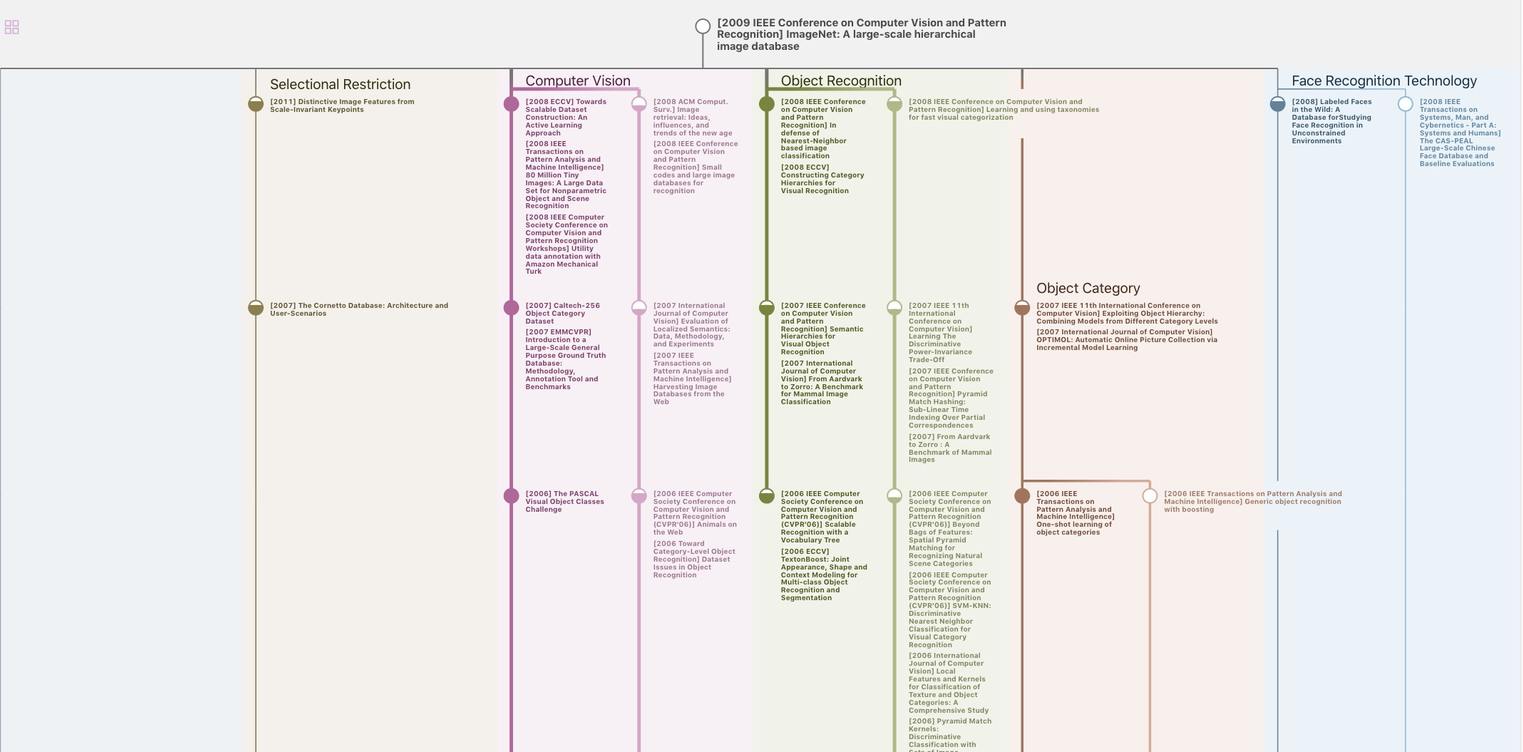
生成溯源树,研究论文发展脉络
Chat Paper
正在生成论文摘要