Enhancement of coastal winds and surface ocean currents with deep learning – The Copernicus Marine Service Evolution KAILANI project
crossref(2024)
摘要
Coastal stakeholders are adding ocean forecasts in their daily operations. The Copernicus Marine Service - Monitoring and Forecasting Centres (CMS - MFCs) are evolving to meet these user new demands. For instance, the wave forecast service at the Iberian-Biscay-Ireland area (IBI-WAV) is being upgraded with increases in model resolution (from 1/20º to 1/40º) and the delivery of new variables (e.g. maximum wave height). Enhancing coastal forcings (winds and surface currents) reinforces these forthcoming upgrades. Higher model resolution benefits local-scale phenomena representation (i.e. wind wave growth or wave refraction due to currents), but errors in the forcings may degrade the expected performance. The Copernicus Marine Service Evolution project KAILANI (2022-2024) aims to improve the accuracy of these operational forcings by correcting them with Artificial Neural Networks (ANNs). These ANNs are fed with remote sensing data as target datasets, allowing to predict complex spatial patterns by using the same forcings as predictors. Three pilot sites have been selected to develop this methodology: (i) the Galician area (NE-Atlantic, macrotidal), (ii) the Canary Islands (NE-Atlantic, mesotidal) and (iii) the Ebro Delta (NW Mediterranean, microtidal). The ANN for coastal winds has been trained with Satellite Synthetic Aperture Radar (SAR; data from the ESA Sentinel-1 mission). Due to the nature of the data (i.e. good spatial coverage, but with revisit times up to several days), the ANN architecture consists of a Generative Adversarial Network (GAN) that uses Convolutional layers for addressing the spatial dimension. The ANN is able to downscale ECMWF-IFS wind fields from 1/10º to 1/40º resolution, adding spatial features learnt a priori from SAR data. The ANN-predicted winds present lower speed bias (up to 30% in specific areas) and RMSD (up to 10%) than original ECMWF-IFS winds. Surface currents are predicted with ANNs based on Autoencoders (AE), using hourly-averaged HF-Radar data as target dataset (retrieved from Copernicus Marine In-Situ TAC). Spatial and time dimension are addressed with Convolutional and Long Short-term Memory (LSTM) layers, respectively. The input data includes (i) IBI-PHY currents and (ii) atmospheric forcings from ECMWF-IFS. The AE improves error metrics respect IBI-PHY, showing speed and directional biases close to 2 cm/s and 7º (achieving bias decreases of 45% and 60% in some areas, respectively). These ANN-driven wind and currents forcings will be used in pre-operational tests by the IBI-WAV service. If successful, this methodology could be transferred into operations of the IBI NRT wave forecast system, as well as to be extended in other CMS regional MFCs.
更多查看译文
AI 理解论文
溯源树
样例
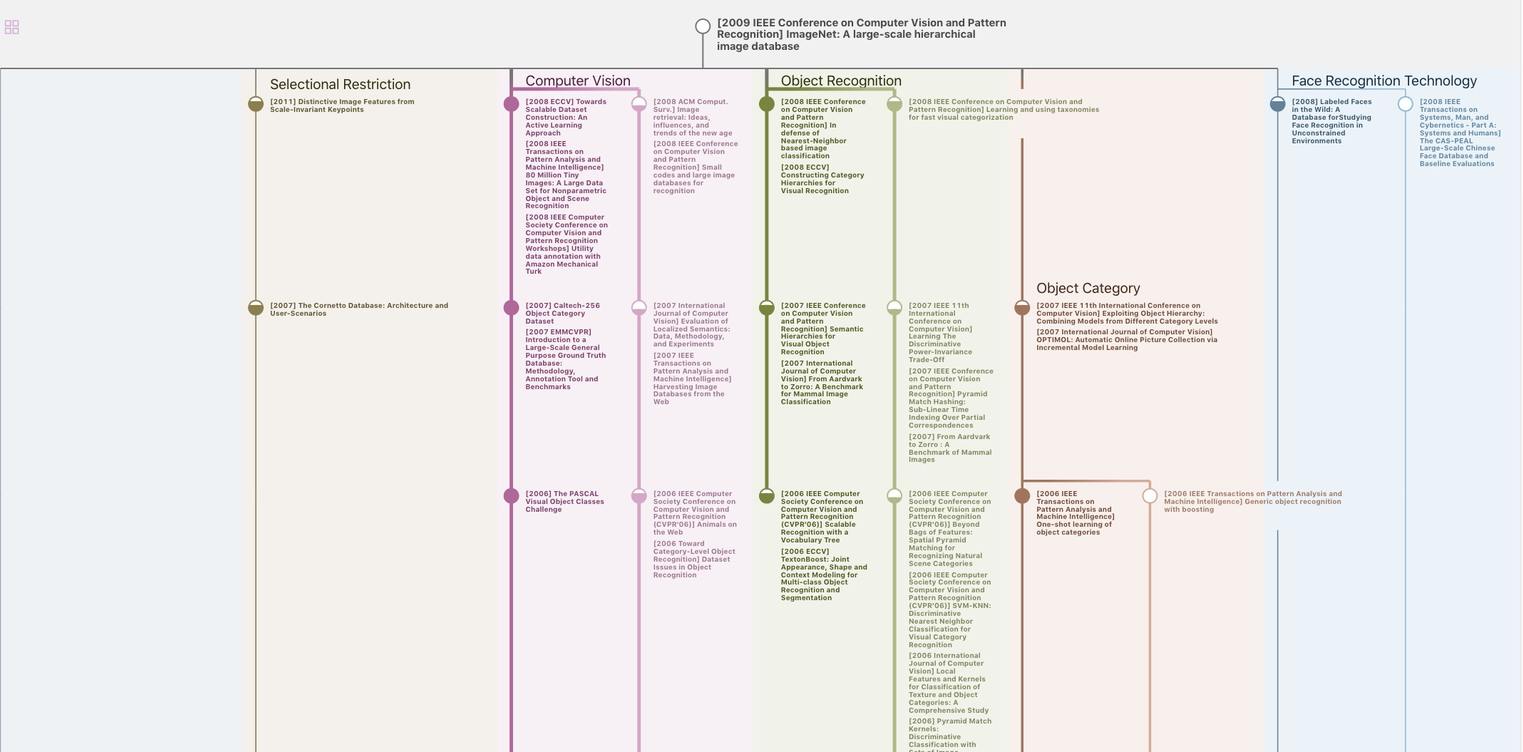
生成溯源树,研究论文发展脉络
Chat Paper
正在生成论文摘要