Electron density-based GPT for optimization and suggestion of host–guest binders
Nature Computational Science(2024)
摘要
Here we present a machine learning model trained on electron density for the production of host–guest binders. These are read out as simplified molecular-input line-entry system (SMILES) format with >98% accuracy, enabling a complete characterization of the molecules in two dimensions. Our model generates three-dimensional representations of the electron density and electrostatic potentials of host–guest systems using a variational autoencoder, and then utilizes these representations to optimize the generation of guests via gradient descent. Finally the guests are converted to SMILES using a transformer. The successful practical application of our model to established molecular host systems, cucurbit[ n ]uril and metal–organic cages, resulted in the discovery of 9 previously validated guests for CB[6] and 7 unreported guests (with association constant K a ranging from 13.5 M −1 to 5,470 M −1 ) and the discovery of 4 unreported guests for [Pd 2 1 4 ] 4+ (with K a ranging from 44 M −1 to 529 M −1 ).
更多查看译文
AI 理解论文
溯源树
样例
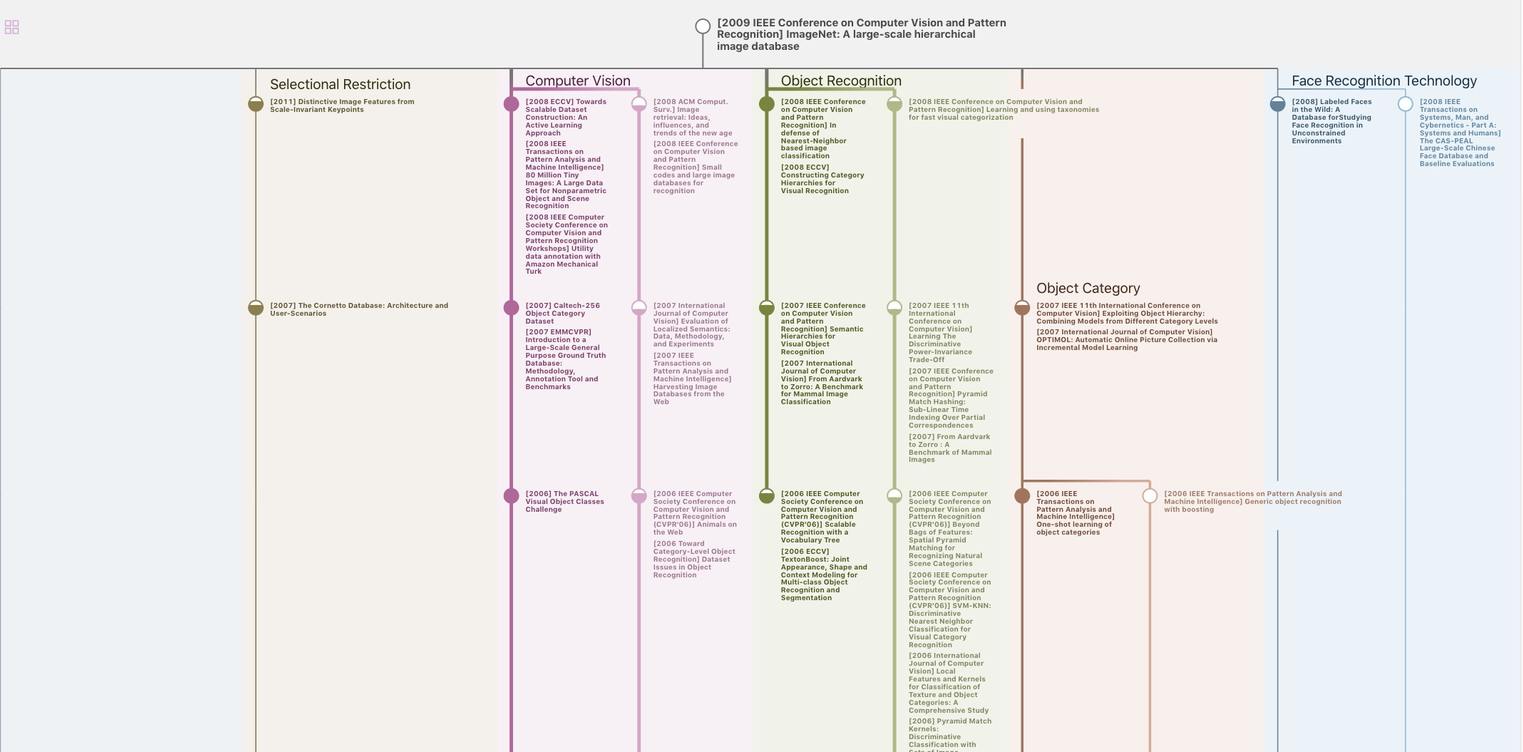
生成溯源树,研究论文发展脉络
Chat Paper
正在生成论文摘要