Detection and classification of Brandt’s vole burrow clusters utilizing GF-2 satellite imagery and faster R-CNN model
Frontiers in Ecology and Evolution(2024)
摘要
Most small rodent populations worldwide exhibit fascinating population dynamics, capturing the attention of numerous scholars due to their multiyear cyclic fluctuations in population size and the astonishing amplitude of these fluctuations. Hulunbuir steppe stands as a crucial global hub for livestock production, yet in recent decades, the area has faced recurring challenges from steppes rodent invasions, with Brandt’s vole (Lasiopodomys brandtii, BV) being particularly rampant among them. They not only exhibit seasonal reproduction but also strong social behavior, and are generally considered pests, especially during population outbreak years. Prior studies suggest that BV population outbreaks tend to occur across a wider geographic area, and a strong indicator for identifying rodent outbreaks is recognizing their burrow clusters (burrow systems). Hence, this paper conducts target object detection of BV burrow clusters in the typical steppes of Hulunbuir using two GF-2 satellite images from 2021 (the year of the BV outbreak). This task is accomplished by incorporating the Faster R-CNN model in combination with three detection approaches: object-based image classification (OBIC), based on vegetation index classification (BVIC), and based on texture classification (BTC). The results indicate that OBIC demonstrated the highest robustness in BV burrow cluster detection, achieving an average AP of 63.80% and an F1 score of 0.722 across the two images. BTC exhibited the second-highest level of accuracy, achieving an average AP of 55.95% and an F1 score of 0.6660. Moreover, this approach displayed a strong performance in BV burrow clusters localization. In contrast, BVIC achieved the lowest level of accuracy among the three methods, with an average AP of only 29.45% and an F1 score of 0.4370. Overall, this study demonstrates the crucial role of utilizing high-resolution satellite imagery combined with DL-based object detection techniques in effectively monitoring and managing the potential outbreaks of steppe rodent pests across larger spatial extents.
更多查看译文
关键词
object detection,faster R-CNN,Brandt’s vole,burrow clusters,GF-2,Hulunbuir
AI 理解论文
溯源树
样例
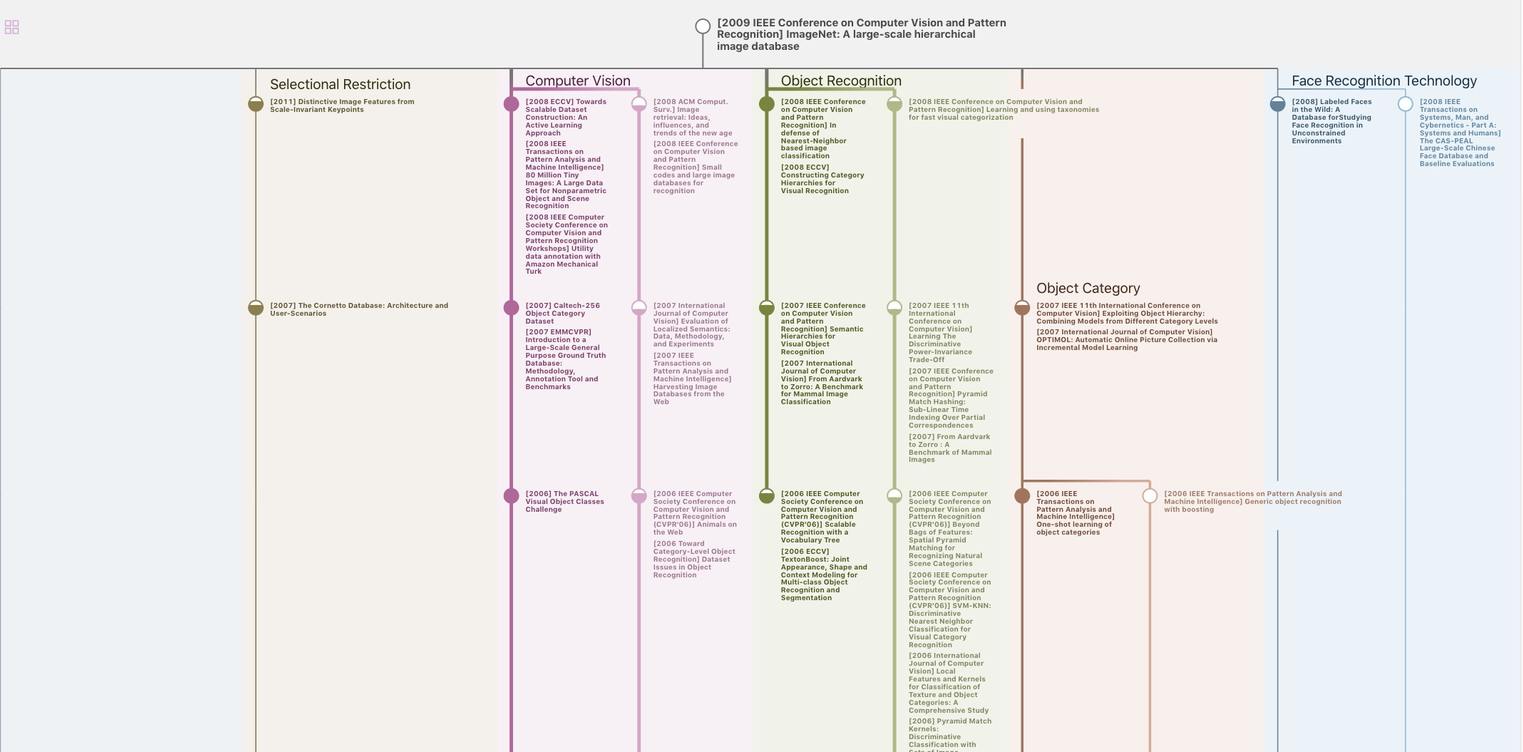
生成溯源树,研究论文发展脉络
Chat Paper
正在生成论文摘要