Accurate Localization in LOS/NLOS Channel Coexistence Scenarios Based on Heterogeneous Knowledge Graph Inference
ACM transactions on sensor networks(2024)
摘要
Accurate localization is one of the basic requirements for smart cities and smart factories. In wireless cellular network localization, the straight-line propagation of electromagnetic waves between base stations and users is called line-of-sight (LOS) wireless propagation. In some cases, electromagnetic wave signals cannot propagate in a straight line due to obstruction by buildings or trees, and these scenarios are usually called non-LOS (NLOS) wireless propagation. Traditional localization algorithms such as TDOA, AOA, etc. , are based on LOS channels, which are no longer applicable in environments where NLOS propagation is dominant, and in most scenarios, the number of base stations with LOS channels containing users is often small, resulting in traditional localization algorithms being unable to satisfy the accuracy demand of high-precision localization. In addition, some nonideal factors may be included in the actual system, all of which can lead to localization accuracy degradation. Therefore, the approach developed in this paper uses knowledge graph and graph neural network (GNN) technology to model communication data as knowledge graphs, and it adopts the knowledge graph inference technique based on a heterogeneous graph attention mechanism to infer unknown data representations in complex scenarios based on the known data and the relationships between the data to achieve high-precision localization in scenarios with LOS/NLOS channel coexistence. We experimentally demonstrate a spatial 2D localization accuracy level of approximately 10 meters on multiple datasets and find that our proposed algorithm has higher accuracy and stronger robustness than the state-of-the-art algorithms.
更多查看译文
关键词
Wireless cellular network localization,Knowledge graph,Graph neural networks,Heterogeneous graph attention mechanism
AI 理解论文
溯源树
样例
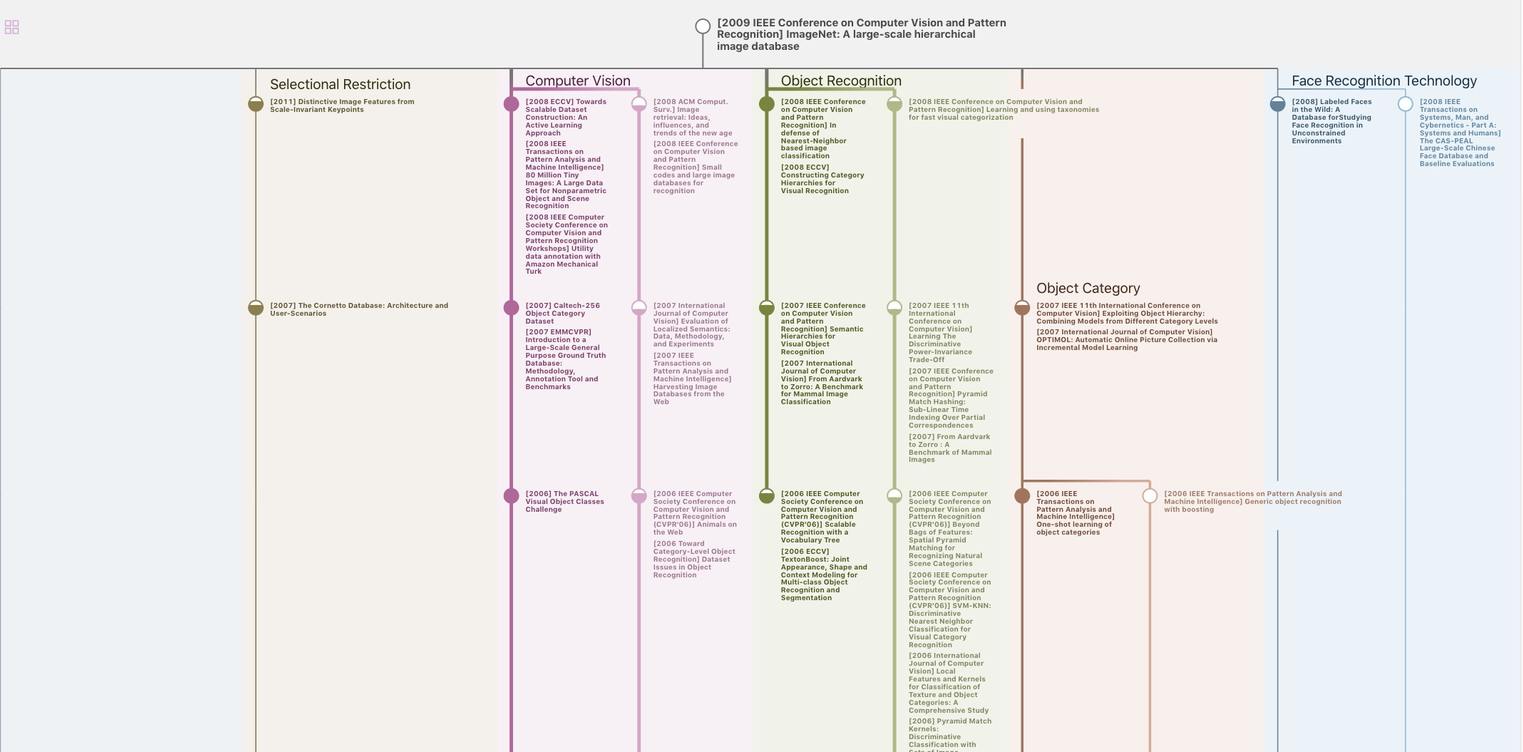
生成溯源树,研究论文发展脉络
Chat Paper
正在生成论文摘要