Towards Generalised Neural Implicit Representations for Image Registration
DEEP GENERATIVE MODELS, DGM4MICCAI 2023(2024)
摘要
Neural implicit representations (NIRs) enable to generate and parametrize the transformation for image registration in a continuous way. By design, these representations are image-pair-specific, meaning that for each signal a new multi-layer perceptron has to be trained. In this work, we investigate for the first time the potential of existent NIR generalisation methods for image registration and propose novel methods for the registration of a group of image pairs using NIRs. To exploit the generalisation potential of NIRs, we encode the fixed and moving image volumes to latent representations, which are then used to condition or modulate the NIR. Using ablation studies on a 3D benchmark dataset, we show that our methods are able to generalise to a set of image pairs with a performance comparable to pairwise registration using NIRs when trained on N = 10 and N = 120 datasets. Our results demonstrate the potential of generalised NIRs for 3D deformable image registration.
更多查看译文
关键词
Image registration,Neural implicit representation,Generalisation,Periodic activation functions
AI 理解论文
溯源树
样例
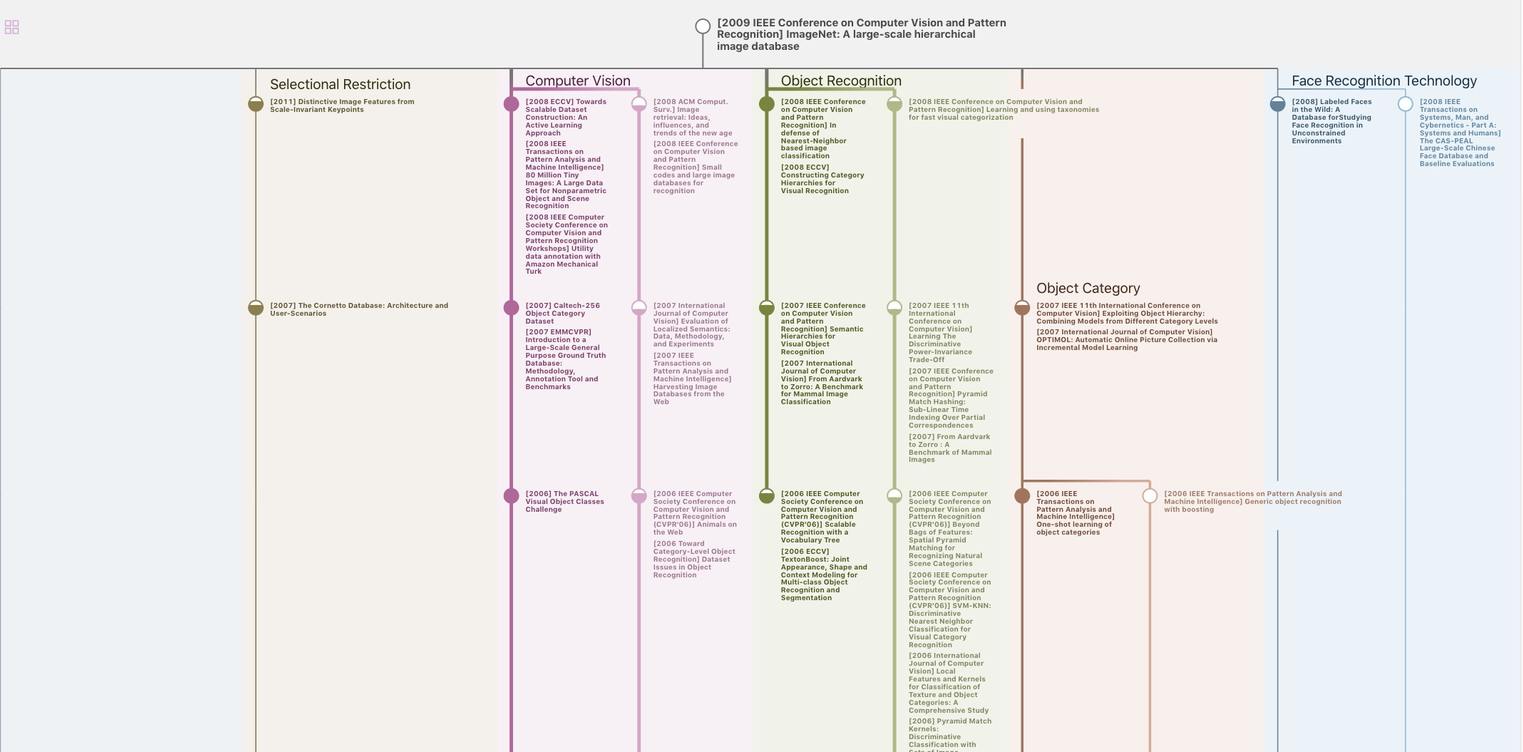
生成溯源树,研究论文发展脉络
Chat Paper
正在生成论文摘要