AuG-KD: Anchor-Based Mixup Generation for Out-of-Domain Knowledge Distillation
ICLR 2024(2024)
Abstract
Due to privacy or patent concerns, a growing number of large models are released without granting access to their training data, making transferring their knowledge inefficient and problematic. In response, Data-Free Knowledge Distillation (DFKD) methods have emerged as direct solutions. However, simply adopting models derived from DFKD for real-world applications suffers significant performance degradation, due to the discrepancy between teachers' training data and real-world scenarios (student domain). The degradation stems from the portions of teachers' knowledge that are not applicable to the student domain. They are specific to the teacher domain and would undermine students' performance. Hence, selectively transferring teachers' appropriate knowledge becomes the primary challenge in DFKD. In this work, we propose a simple but effective method AuG-KD. It utilizes an uncertainty-guided and sample-specific anchor to align student-domain data with the teacher domain and leverages a generative method to progressively trade off the learning process between OOD knowledge distillation and domain-specific information learning via mixup learning. Extensive experiments in 3 datasets and 8 settings demonstrate the stability and superiority of our approach.
MoreTranslated text
Key words
out-of-domain knowledge distillation,mixup learning,domain shift,uncertainty
AI Read Science
Must-Reading Tree
Example
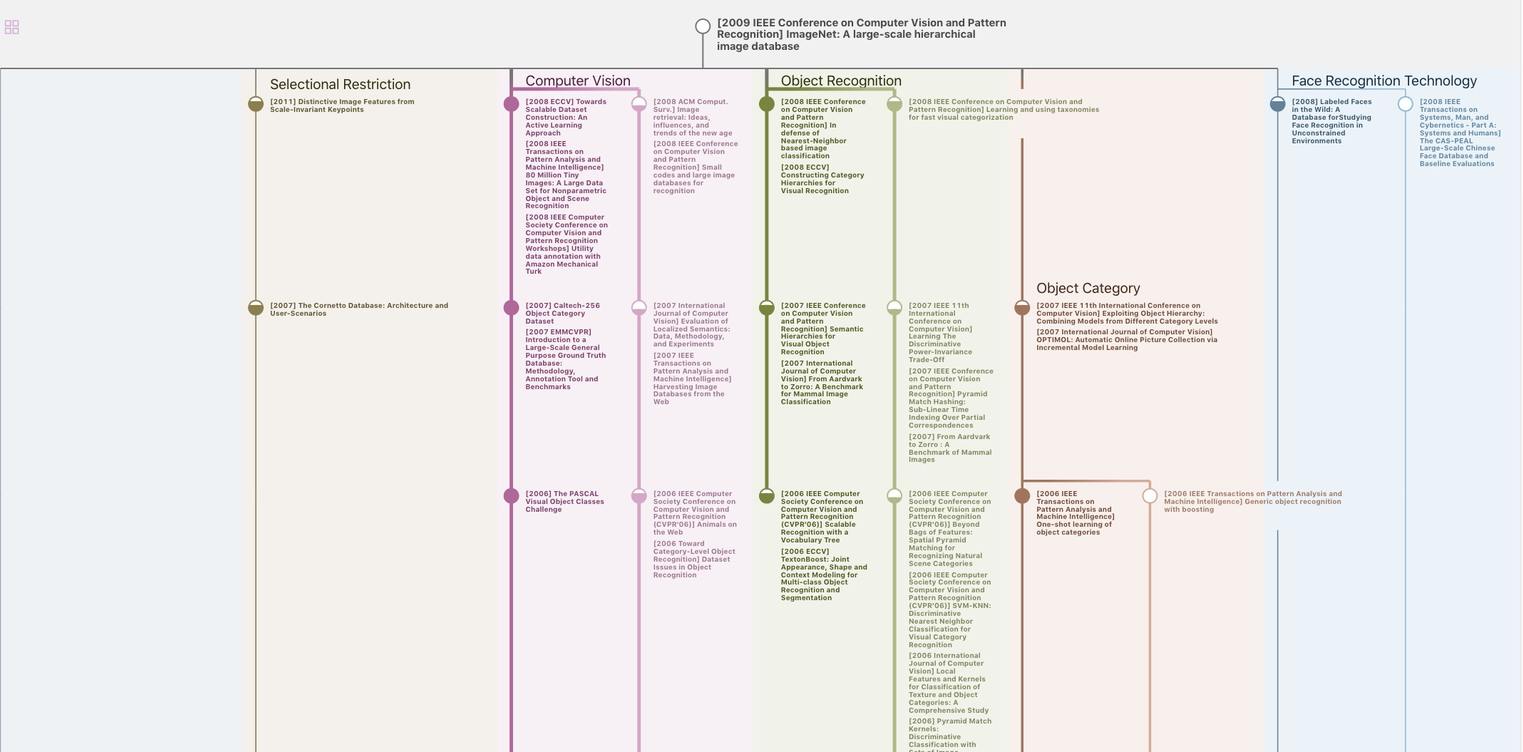
Generate MRT to find the research sequence of this paper
Chat Paper
Summary is being generated by the instructions you defined