BioBridge: Bridging Biomedical Foundation Models via Knowledge Graphs
ICLR 2024(2024)
摘要
Foundation models (FMs) learn from large volumes of unlabeled data to demonstrate superior performance across a wide range of tasks. However, FMs developed for biomedical domains have largely remained unimodal, i.e., independently trained and used for tasks on protein sequences alone, small molecule structures alone, or clinical data alone.
To overcome this limitation, we present BioBridge, a parameter-efficient learning framework, to bridge independently trained unimodal FMs to establish multimodal behavior. BioBridge achieves it by utilizing Knowledge Graphs (KG) to learn transformations between one unimodal FM and another without fine-tuning any underlying unimodal FMs.
Our results demonstrate that BioBridge can
beat the best baseline KG embedding methods (on average by ~ 76.3%) in cross-modal retrieval tasks. We also identify BioBridge demonstrates out-of-domain generalization ability by extrapolating to unseen modalities or relations. Additionally, we also show that BioBridge presents itself as a general-purpose retriever that can aid biomedical multimodal question answering as well as enhance the guided generation of novel drugs. Code is at https://github.com/RyanWangZf/BioBridge.
更多查看译文
关键词
drug discovery,foundation model,multi-modal learning,knowledge graph
AI 理解论文
溯源树
样例
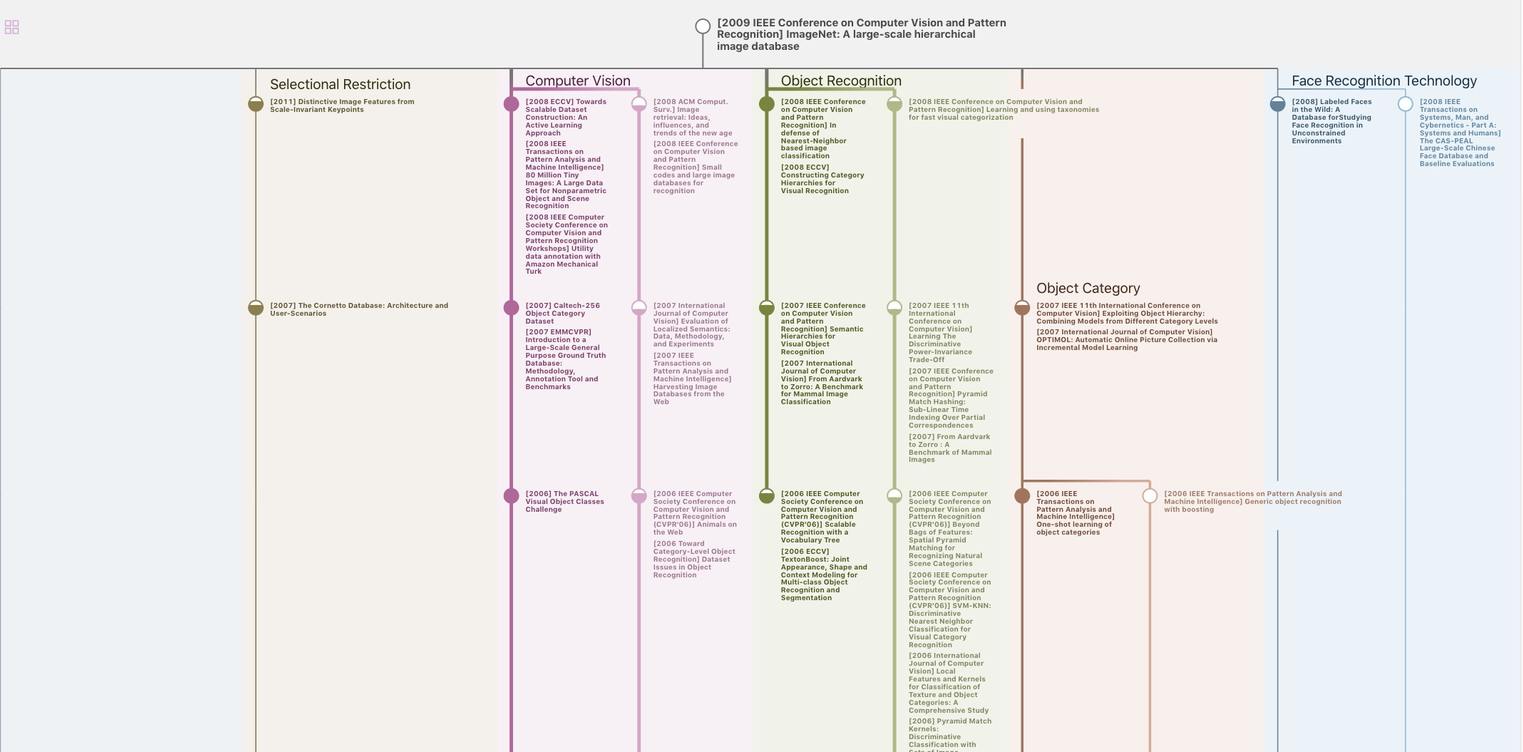
生成溯源树,研究论文发展脉络
Chat Paper
正在生成论文摘要