Unknown Domain Inconsistency Minimization for Domain Generalization
ICLR 2024(2024)
摘要
The objective of domain generalization (DG) is to enhance the transferability
of the model learned from a source domain to unobserved domains. To prevent
overfitting to a specific domain, Sharpness-Aware Minimization (SAM) reduces
source domain's loss sharpness. Although SAM variants have delivered
significant improvements in DG, we highlight that there's still potential for
improvement in generalizing to unknown domains through the exploration on data
space. This paper introduces an objective rooted in both parameter and data
perturbed regions for domain generalization, coined Unknown Domain
Inconsistency Minimization (UDIM). UDIM reduces the loss landscape
inconsistency between source domain and unknown domains. As unknown domains are
inaccessible, these domains are empirically crafted by perturbing instances
from the source domain dataset. In particular, by aligning the loss landscape
acquired in the source domain to the loss landscape of perturbed domains, we
expect to achieve generalization grounded on these flat minima for the unknown
domains. Theoretically, we validate that merging SAM optimization with the UDIM
objective establishes an upper bound for the true objective of the DG task. In
an empirical aspect, UDIM consistently outperforms SAM variants across multiple
DG benchmark datasets. Notably, UDIM shows statistically significant
improvements in scenarios with more restrictive domain information,
underscoring UDIM's generalization capability in unseen domains. Our code is
available at .
更多查看译文
关键词
Robustness,Domain generalization,Sharpness-Aware Minimization,Loss Sharpness,Inconsistency
AI 理解论文
溯源树
样例
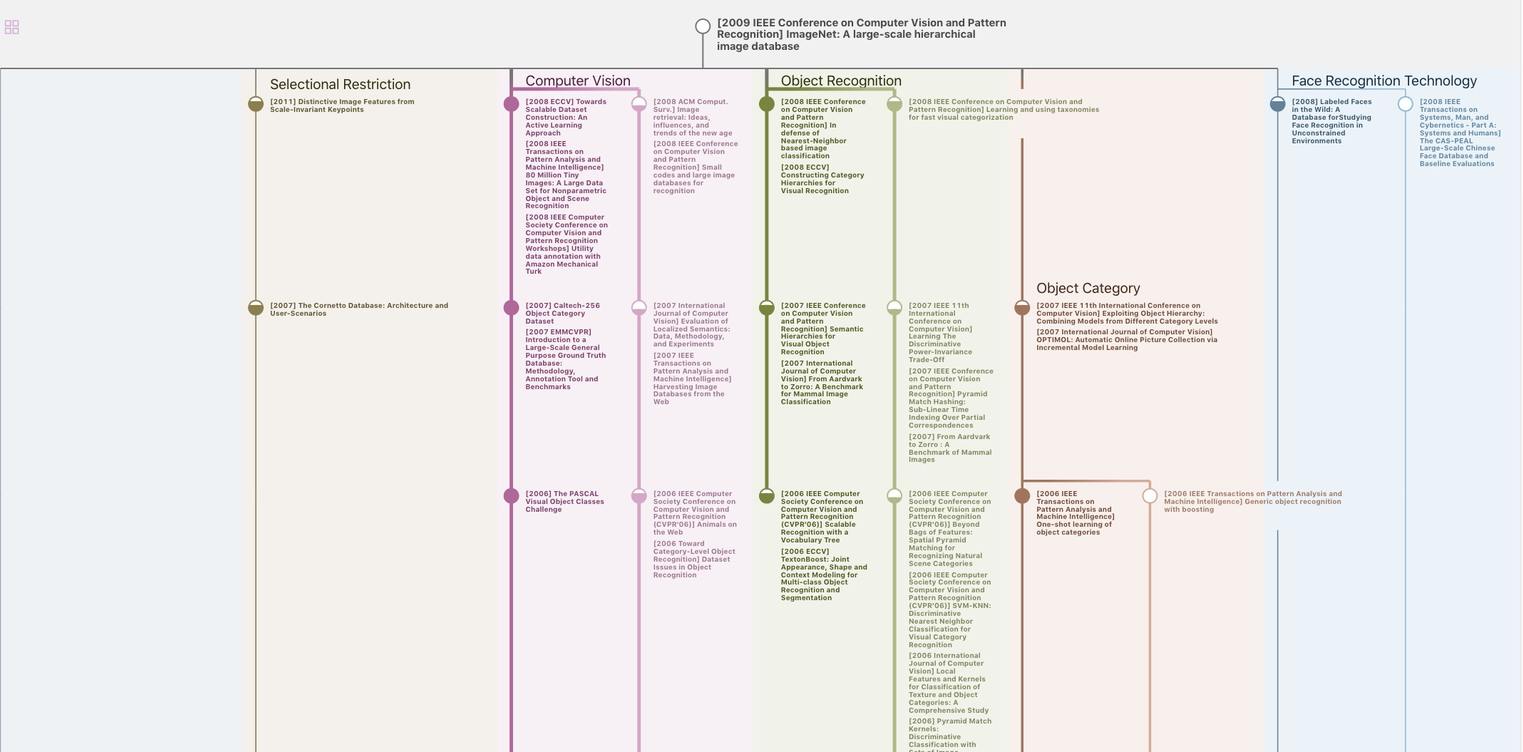
生成溯源树,研究论文发展脉络
Chat Paper
正在生成论文摘要