LabelDP-Pro: Learning with Label Differential Privacy via Projections
ICLR 2024(2024)
摘要
Label differentially private (label DP) algorithms seek to preserve the privacy of the labels in a training dataset in settings where the features are known to the adversary. In this work, we study a new family of label DP training algorithms. Unlike most prior label DP algorithms that have been based on label randomization, our algorithm naturally leverages the power of the central model of DP. It interleaves gradient projection operations with private stochastic gradient descent steps in order to improve the utility of the trained model while guaranteeing the privacy of the labels. We show that such projection-based algorithms can be made practical and that they improve on the state-of-the art for label DP training in the high-privacy regime. We complement our empirical evaluation with theoretical results shedding light on the efficacy of our method through the lens of bias-variance trade-offs.
更多查看译文
关键词
Differential Privacy,Label Differential Privacy,Projections
AI 理解论文
溯源树
样例
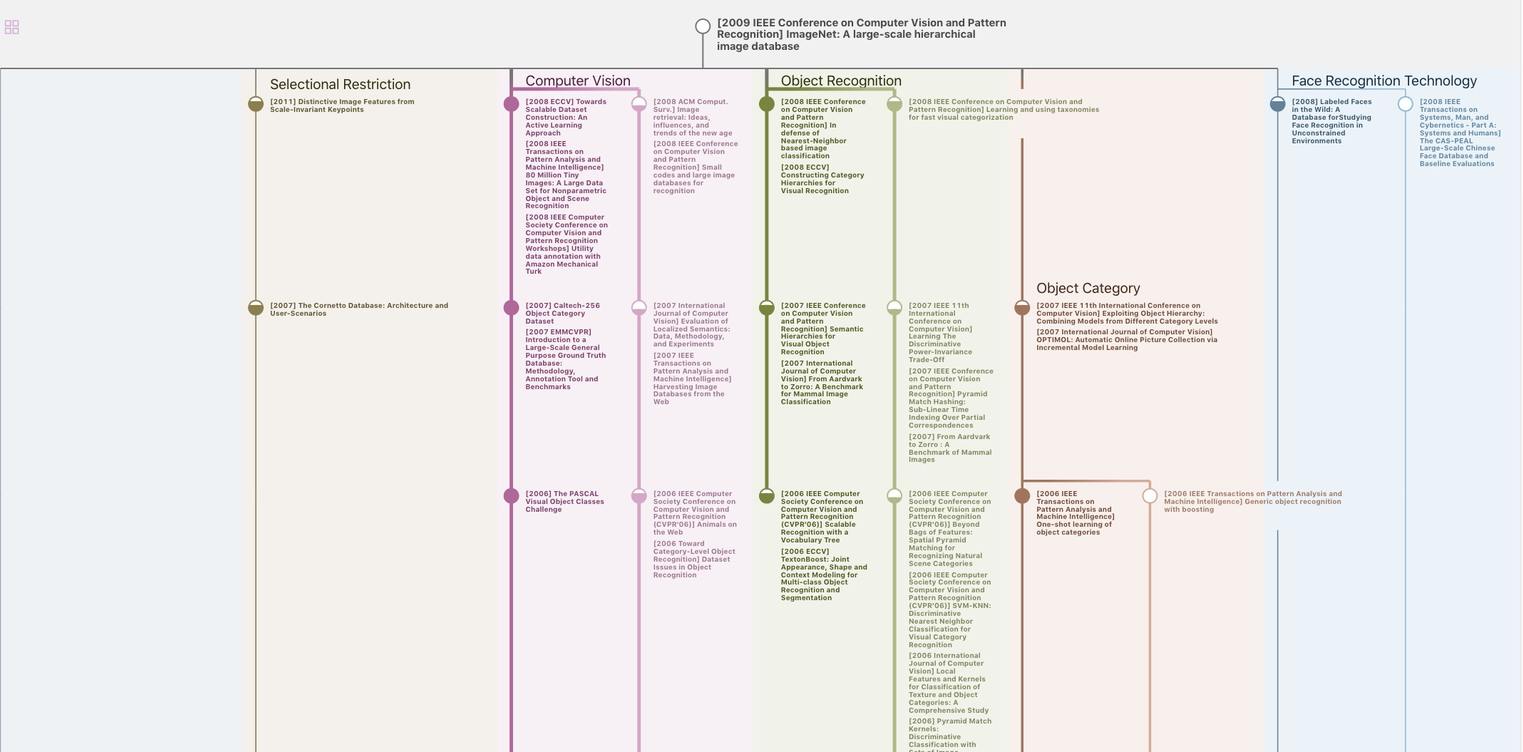
生成溯源树,研究论文发展脉络
Chat Paper
正在生成论文摘要