Rethinking the Benefits of Steerable Features in 3D Equivariant Graph Neural Networks
ICLR 2024(2024)
摘要
Theoretical and empirical comparisons have been made to assess the expressive power and performance of invariant and equivariant GNNs. However, there is currently no theoretical result comparing the expressive power of $k$-hop invariant GNNs and equivariant GNNs. Additionally, little is understood about whether the performance of equivariant GNNs, employing steerable features up to type-$L$, increases as $L$ grows -- especially when the feature dimension is held constant. In this study, we introduce a key lemma that allows us to analyze steerable features by examining their corresponding invariant features. The lemma facilitates us in understanding the limitations of $k$-hop invariant GNNs, which fail to capture the global geometric structure due to the loss of geometric information between local structures. Furthermore, we investigate the invariant features associated with different types of steerable features and demonstrate that the expressiveness of steerable features is primarily determined by their dimension -- independent of their irreducible decomposition. This suggests that when the feature dimension is constant, increasing $L$ does not lead to essentially improved performance in equivariant GNNs employing steerable features up to type-$L$. We substantiate our theoretical insights with numerical evidence.
更多查看译文
关键词
Steerable features,Equivariant graph neural networks,Message passing
AI 理解论文
溯源树
样例
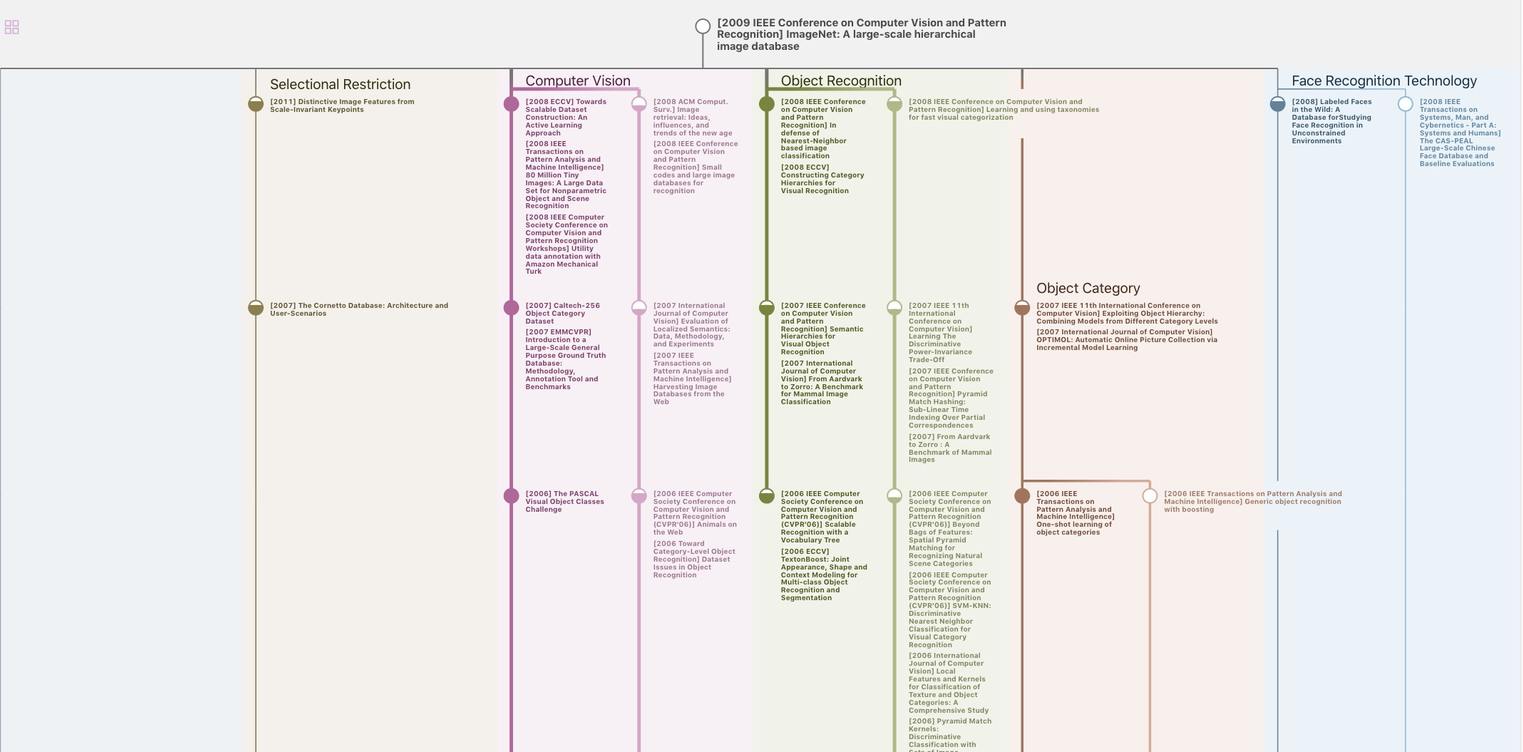
生成溯源树,研究论文发展脉络
Chat Paper
正在生成论文摘要