Scale-Adaptive Diffusion Model for Complex Sketch Synthesis
ICLR 2024(2024)
摘要
While diffusion models have revolutionized generative AI, their application to human sketch generation, especially in the creation of complex yet concise and recognizable sketches, remains largely unexplored. Existing efforts have primarily focused on vector-based sketches, limiting their ability to handle intricate sketch data. This paper introduces an innovative extension of diffusion models to pixellevel sketch generation, addressing the challenge of dynamically optimizing the guidance scale for classifier-guided diffusion. Our approach achieves a delicate balance between recognizability and complexity in generated sketches through scale-adaptive classifier-guided diffusion models, a scaling indicator, and the concept of a residual sketch. We also propose a three-phase sampling strategy to enhance sketch diversity and quality. Experiments on the QuickDraw dataset showcase the potential of diffusion models to push the boundaries of sketch generation, particularly in complex scenarios unattainable by vector-based methods.
更多查看译文
关键词
Generative model,Classifier-based Diffusion Model,Sketch
AI 理解论文
溯源树
样例
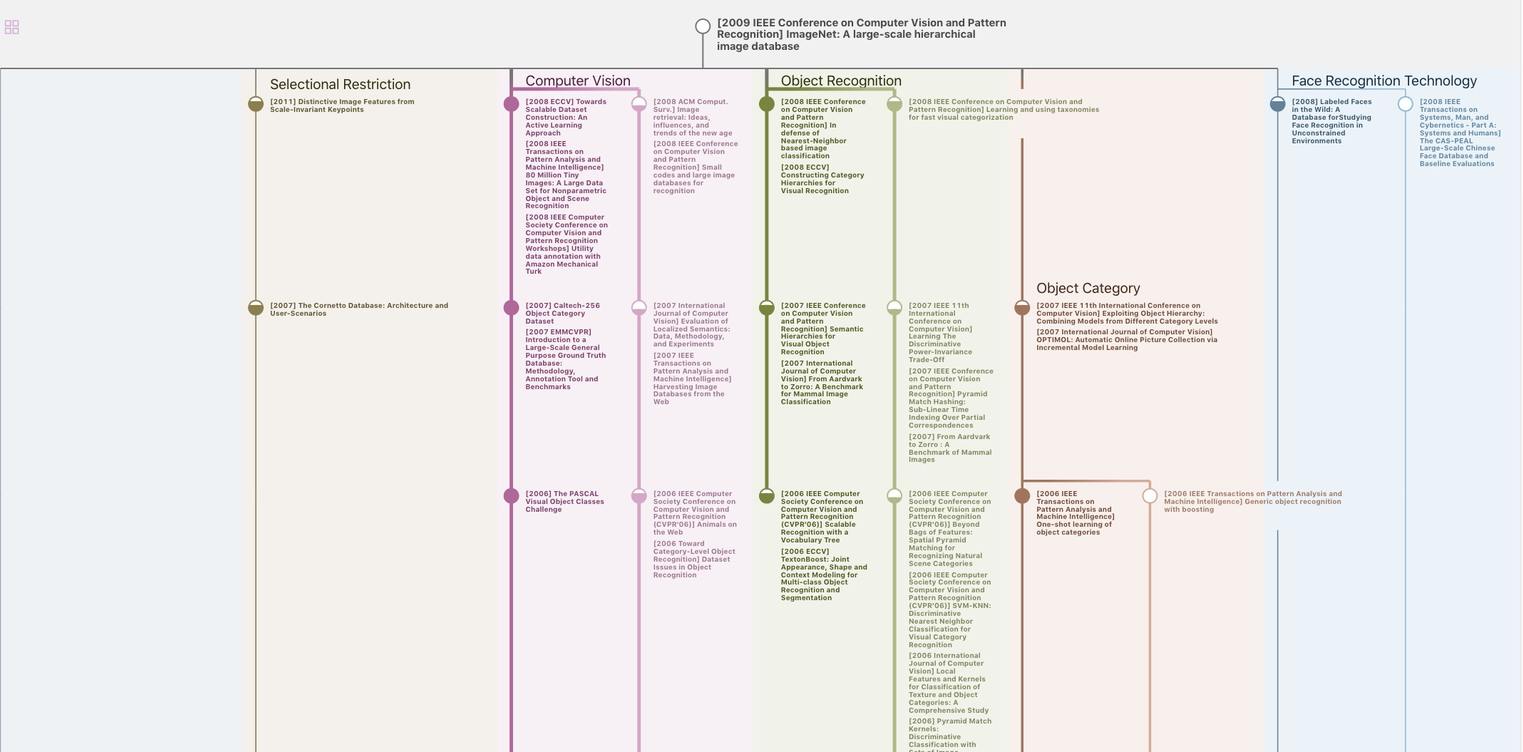
生成溯源树,研究论文发展脉络
Chat Paper
正在生成论文摘要