Boosting Graph Anomaly Detection with Adaptive Message Passing
ICLR 2024(2024)
摘要
Unsupervised graph anomaly detection has been widely used in real-world applications. Existing methods primarily focus on local inconsistency mining (LIM), based on the intuition that establishing high similarities between abnormal nodes and their neighbors is difficult. However, the message passing employed by graph neural networks (GNNs) results in local anomaly signal loss, as GNNs tend to make connected nodes similar, which conflicts with the LIM intuition. In this paper, we propose GADAM, a novel framework that not only resolves the conflict between LIM and message passing but also leverages message passing to augment anomaly detection through a transformative approach to anomaly mining beyond LIM. Specifically, we first propose an efficient MLP-based LIM approach to obtain local anomaly scores in a conflict-free way. Next, we introduce a novel approach to capture anomaly signals from a global perspective. This involves a hybrid attention based adaptive message passing, enabling nodes to selectively absorb abnormal or normal signals from their surroundings. Extensive experiments conducted on nine benchmark datasets, including two large-scale OGB datasets, demonstrate that GADAM surpassinges existing state-of-the-art methods in terms of both effectiveness and efficiency.
更多查看译文
关键词
Graph neural network,unsupervised anomaly detection
AI 理解论文
溯源树
样例
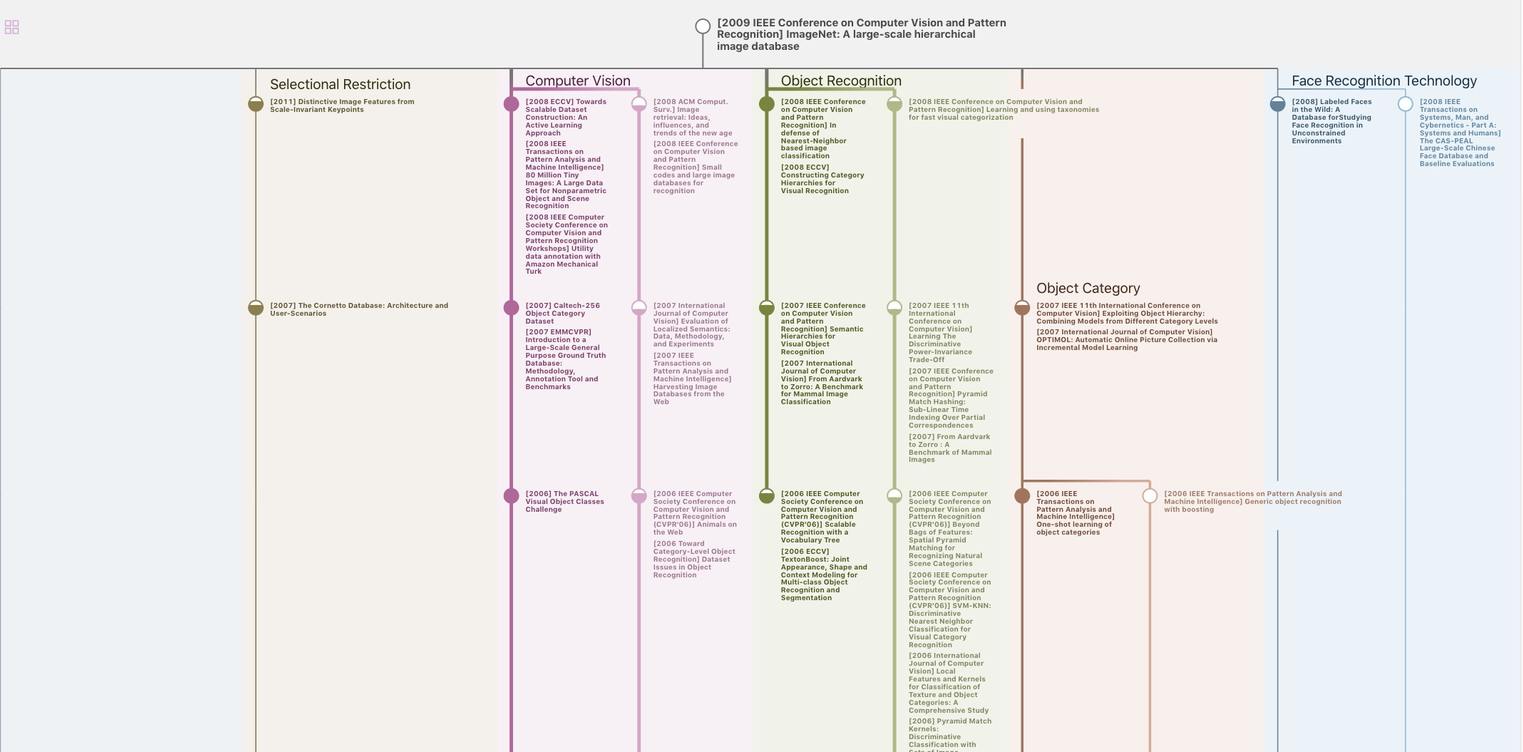
生成溯源树,研究论文发展脉络
Chat Paper
正在生成论文摘要