Mitigating Severe Robustness Degradation on Graphs
ICLR 2024(2024)
摘要
Although graph neural networks have exhibited remarkable performance in various graph tasks, a significant concern is their vulnerability to adversarial attacks. Consequently, many defense methods have been proposed to alleviate the deleterious effects of adversarial attacks and learn robust graph representations. However, most of them are difficult to *simultaneously* avoid two major limitations: (i) an emergent and severe degradation in robustness when exposed to very intense attacks, and (ii) heavy computation complexity hinders them from scaling to large graphs. In response to these challenges, we introduce an innovative graph defense method for unpredictable real-world scenarios by *designing a graph robust learning framework that is resistant to robustness degradation* and *refraining from unscalable designs with heavy computation*: specifically, our method employs a denoising module, which eliminates edges that are associated with attacked nodes to reconstruct a cleaner graph; Then, it applies Mixture-of-Experts to select differentially private noises with varying magnitudes to counteract the hidden features attacked at different intensities toward robust predictions; Moreover, our overall design avoids the reliance on heavy adjacency matrix computations, such as SVD, thus facilitating its applicability even on large graphs. Comprehensive experiments have been conducted to demonstrate the anti-degraded robustness and scalability of our method, as compared to popular graph adversarial learning methods, under diverse attack intensities and various datasets of different sizes.
更多查看译文
关键词
Graph neural network
AI 理解论文
溯源树
样例
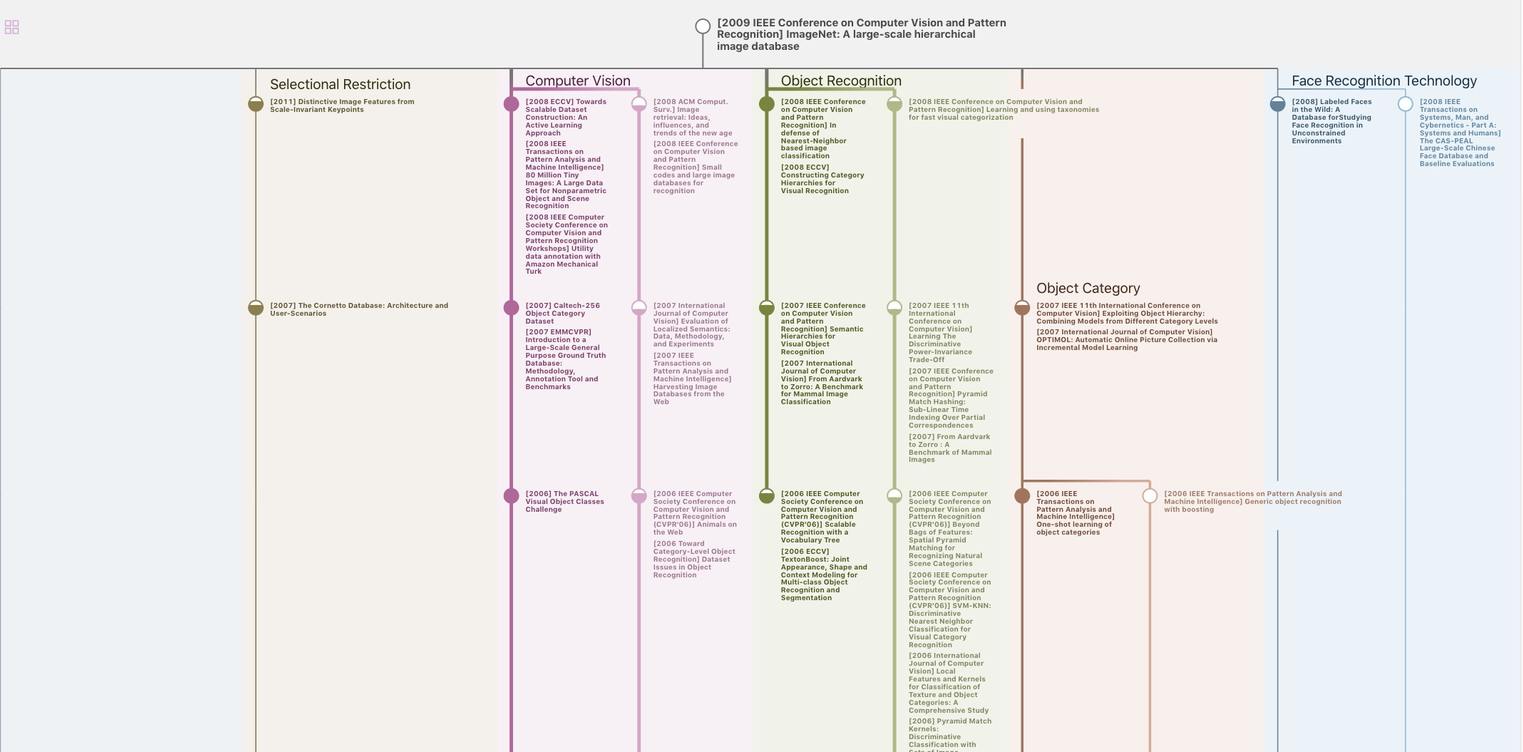
生成溯源树,研究论文发展脉络
Chat Paper
正在生成论文摘要