Decoupled Marked Temporal Point Process using Neural Ordinary Differential Equations
ICLR 2024(2024)
摘要
A Marked Temporal Point Process (MTPP) is a stochastic process whose realization is a set of event-time data. MTPP is often used to understand complex dynamics of asynchronous temporal events such as money transaction, social media, healthcare, etc. Recent studies have utilized deep neural networks to capture complex temporal dependencies of events and generate embedding that aptly represent the observed events. While most previous studies focus on the inter-event dependencies and their representations, how individual events influence the overall dynamics over time has been under-explored. In this regime, we propose a Decoupled MTPP framework that disentangles characterization of a stochastic process into a set of evolving influences from different events. Our approach employs Neural Ordinary Differential Equations (Neural ODEs) to learn flexible continuous dynamics of these influences while simultaneously addressing multiple inference problems, such as density estimation and survival rate computation. We emphasize the significance of disentangling the influences by comparing our framework with state-of-the-art methods on real-life datasets, and provide analysis on the model behavior for potential applications.
更多查看译文
关键词
Marked Temporal Point Process,Neural ODE
AI 理解论文
溯源树
样例
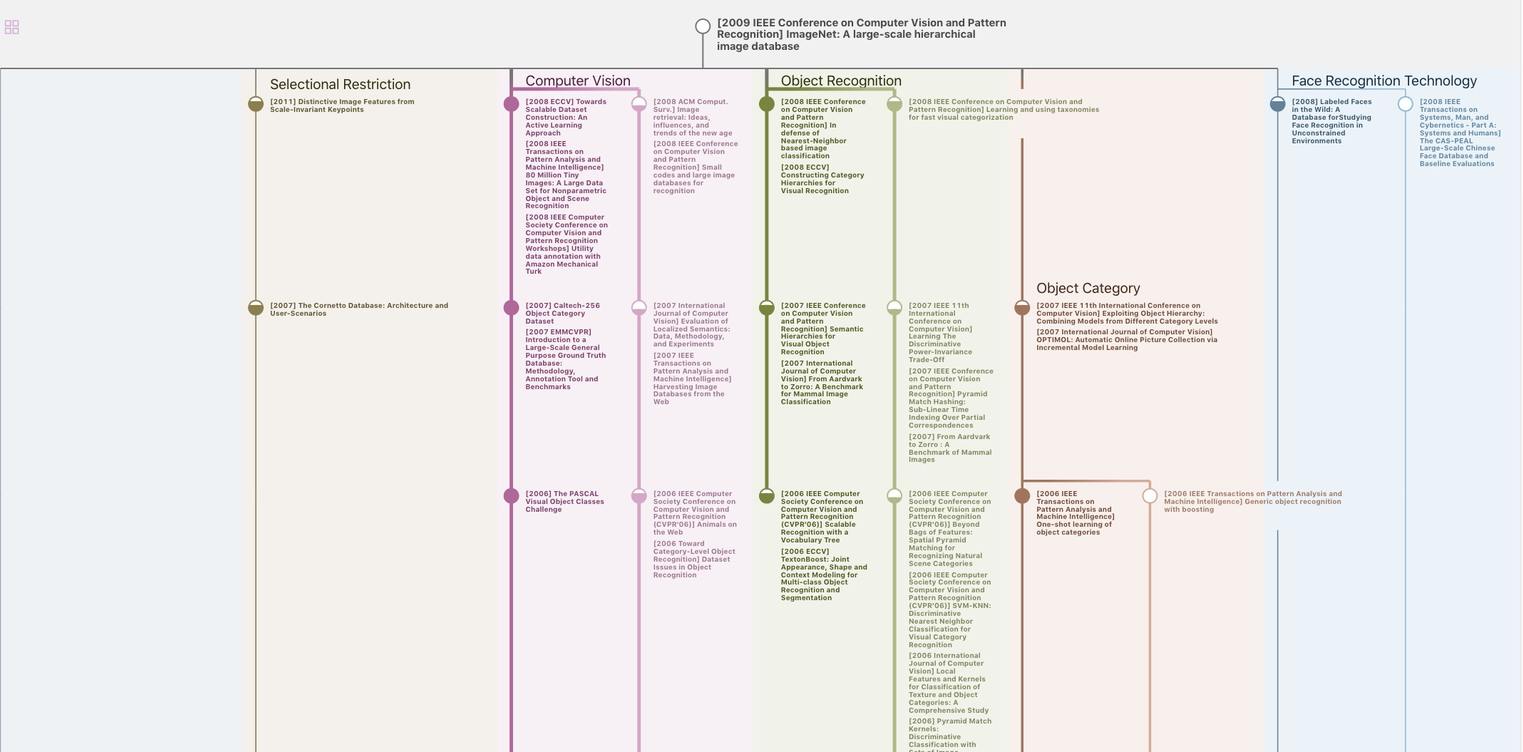
生成溯源树,研究论文发展脉络
Chat Paper
正在生成论文摘要