More is Better: when Infinite Overparameterization is Optimal and Overfitting is Obligatory
ICLR 2024(2024)
摘要
In our era of enormous neural networks, empirical progress has been driven by the philosophy that *more is better.*
Recent deep learning practice has found repeatedly that larger model size, more data, and more computation (resulting in lower training loss) optimizing to near-interpolation improves performance. In this paper, we give theoretical backing to these empirical observations by showing that these three properties hold in random feature (RF) regression, a class of models equivalent to shallow networks with only the last layer trained.
Concretely, we first show that the test risk of RF regression decreases monotonically with both the number of features and samples, provided the ridge penalty is tuned optimally. In particular, this implies that infinite width RF architectures are preferable to those of any finite width. We then proceed to demonstrate that, for a large class of tasks characterized by powerlaw eigenstructure, training to near-zero training loss is *obligatory:* near-optimal performance can *only* be achieved when the training error is much smaller than the test error. Grounding our theory in real-world data, we find empirically that standard computer vision tasks with convolutional neural kernels clearly fall into this class. Taken together, our results tell a simple, testable story of the benefits of overparameterization and overfitting in random feature models.
更多查看译文
关键词
overparameterization,interpolation,random feature regression,kernel regression,generalization,overfitting
AI 理解论文
溯源树
样例
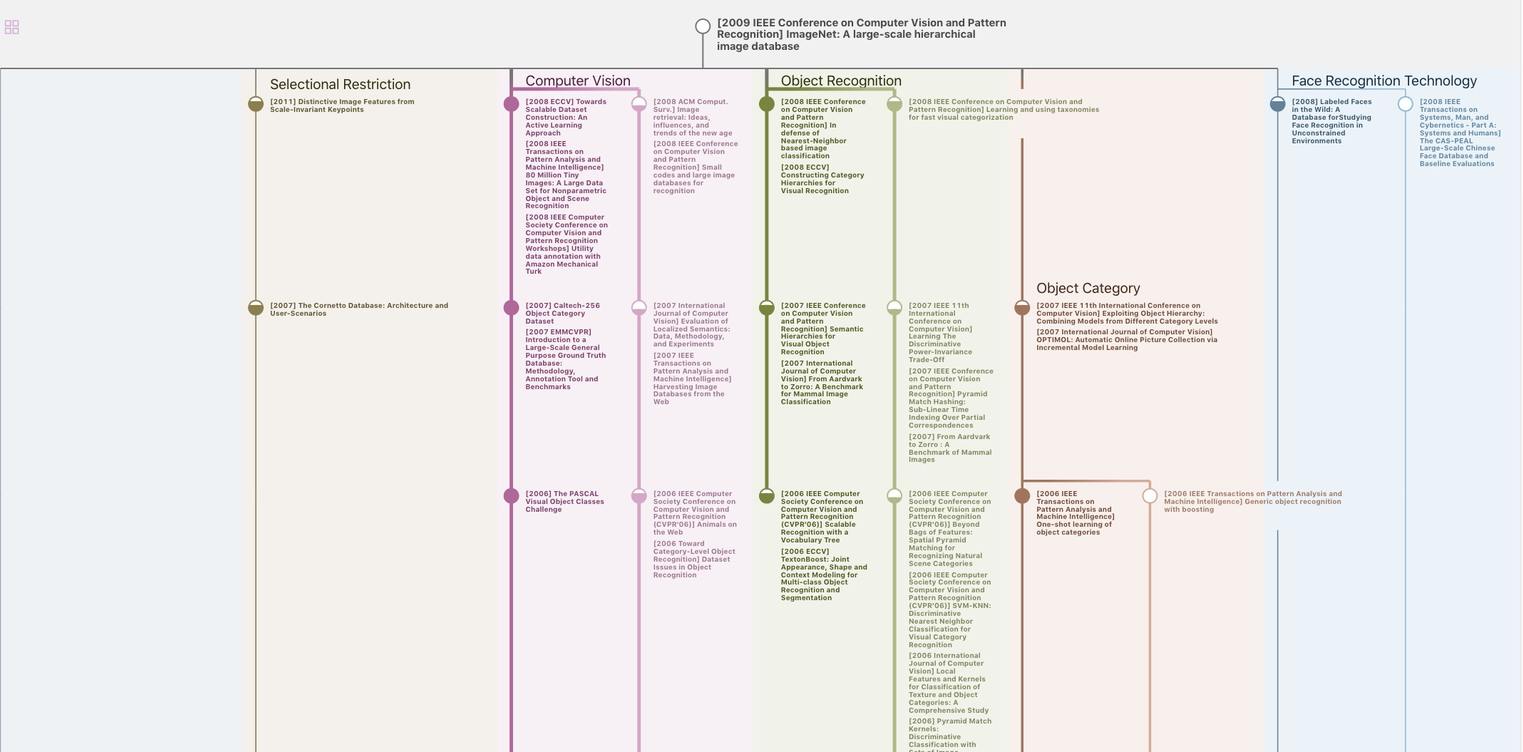
生成溯源树,研究论文发展脉络
Chat Paper
正在生成论文摘要