A Study of Generalization in Offline Reinforcement Learning
ICLR 2024(2024)
摘要
Despite the recent progress in offline reinforcement learning (RL) algorithms, agents are usually trained and tested on the same environment. In this paper, we perform an in-depth study of the generalization abilities of offline RL algorithms, showing that they struggle to generalize to new environments. We also introduce the first benchmark for evaluating generalization in offline learning, collecting datasets with varying sizes and skill-levels from Procgen (2D video games) and WebShop (e-commerce websites). The datasets contain trajectories for a limited number of game levels or natural language instructions and at test time, the agent has to generalize to new levels or instructions. Our experiments reveal that existing offline learning algorithms perform significantly worse than online RL on both train and test environments. Behavioral cloning is a strong baseline, typically outperforming offline RL and sequence modeling approaches when trained on data from multiple environments and tested on new ones. Finally, we find that increasing the diversity of the data, rather than its size, improves generalization for all algorithms. Our study demonstrates the limited generalization of current offline learning algorithms highlighting the need for more research in this area.
更多查看译文
关键词
Offline RL,Dataset,Generalization,Procgen,Webshop
AI 理解论文
溯源树
样例
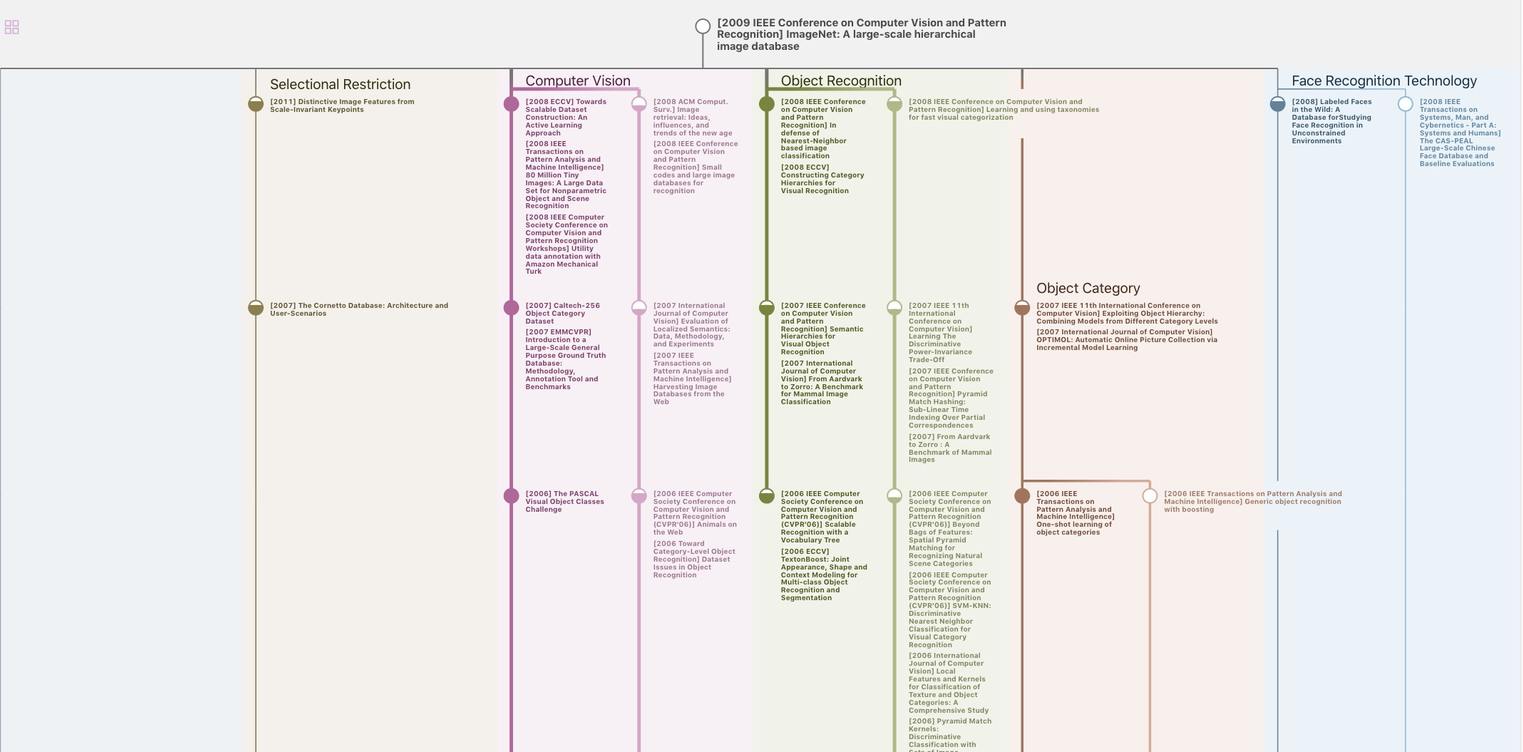
生成溯源树,研究论文发展脉络
Chat Paper
正在生成论文摘要