Compositional Conservatism: A Transductive Approach in Offline Reinforcement Learning
ICLR 2024(2024)
摘要
Offline Reinforcement learning (RL) is a compelling framework for learning optimal policies without additional environmental interaction.
Nevertheless, offline RL inevitably faces the problem of distributional shifts, where the states and actions encountered during policy execution are not in the training dataset.
A common solution involves incorporating conservatism into either the policy or value function, which serves as a safeguard against uncertainties and unknowns.
In this paper, we also focus on achieving the same objectives of conservatism but from a different perspective.
We propose COmpositional COnservatism with Anchor-seeking ($\text{\textit{COCOA}}$) for offline RL, an approach that pursues conservatism in a compositional manner on top of the transductive reparameterization (Netanyahu
et al., 2023).
In this reparameterization, the input variable (the state in our case) is viewed as the combination of an anchor and its difference from the original input.
Independently of and agnostically to the prevalent $\text{\textit{behavioral}}$ conservatism in offline RL, COCOA learns to seek both in-distribution anchors and differences with the learned dynamics model, encouraging conservatism in the $\text{\textit{compositional input space}}$ for the function approximators of the Q-function and policy.
Our experimental results show that our method generally improves the performance of four state-of-the-art offline RL algorithms on the D4RL benchmark.
更多查看译文
关键词
offline reinforcement learning,compositional generalization,conservatism,transduction
AI 理解论文
溯源树
样例
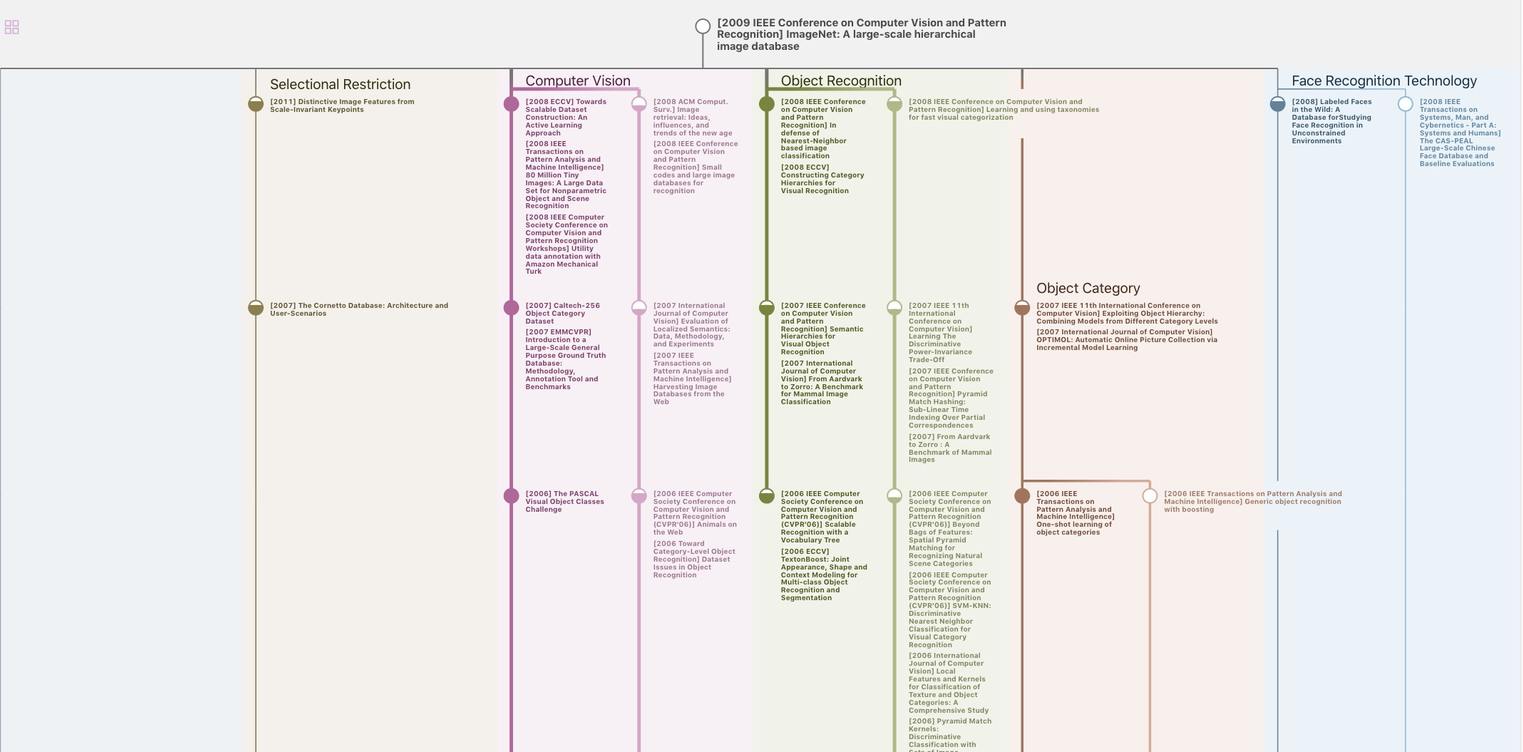
生成溯源树,研究论文发展脉络
Chat Paper
正在生成论文摘要