Faster Approximation of Probabilistic and Distributional Values Via Least Squares
ICLR 2024(2024)
Key words
data valuation,probabilistic values,approximation,distributional Shapley value
AI Read Science
Must-Reading Tree
Example
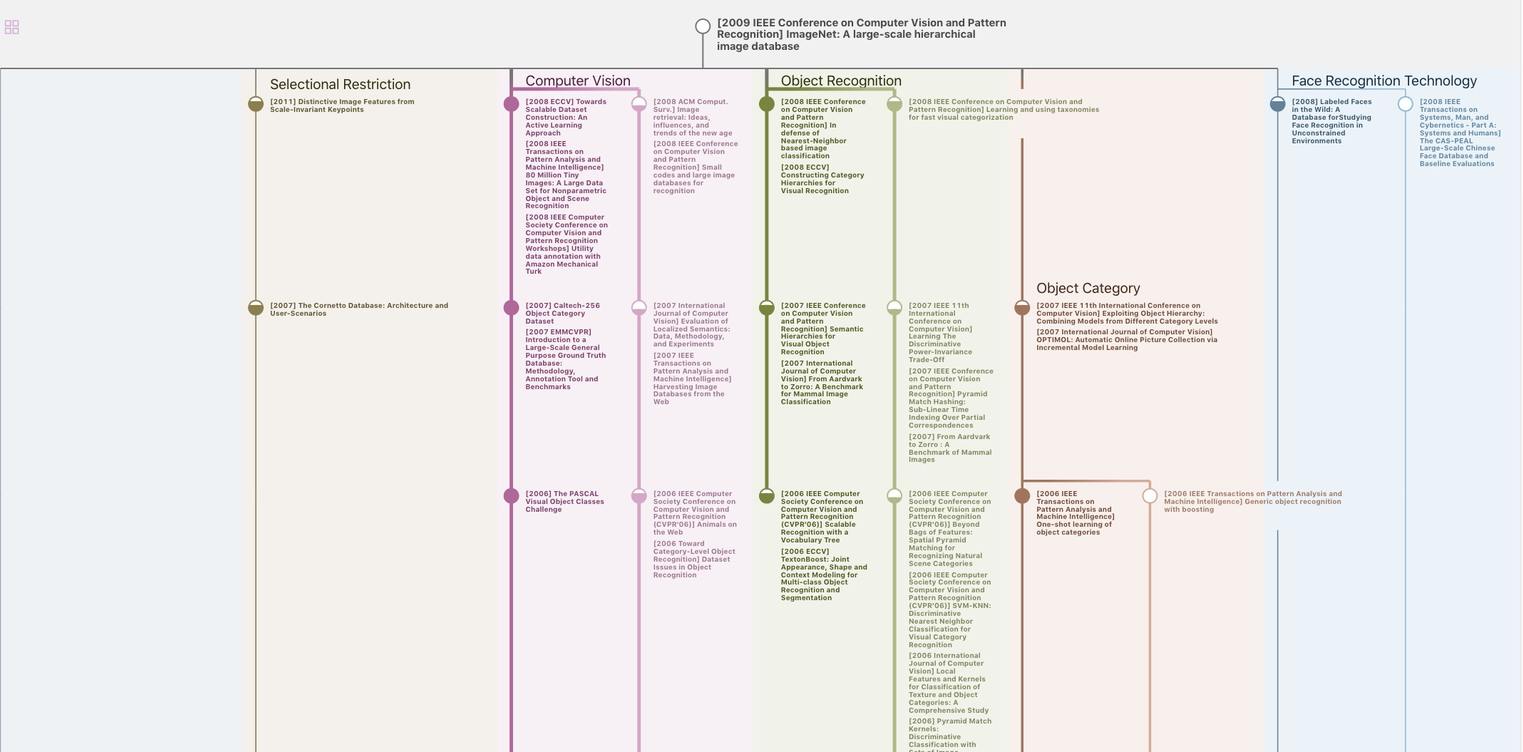
Generate MRT to find the research sequence of this paper
Chat Paper
Summary is being generated by the instructions you defined