Learning From Simplicial Data Based on Random Walks and 1D Convolutions
ICLR 2024(2024)
摘要
Triggered by limitations of graph-based deep learning methods in terms of computational expressivity and model flexibility, recent years have seen a surge of interest in computational models that operate on higher-order topological domains such as hypergraphs and simplicial complexes. While the increased expressivity of these models can indeed lead to a better classification performance and a more faithful representation of the underlying system, the computational cost of these higher-order models can increase dramatically. To this end, we here explore a simplicial complex neural network learning architecture based on random walks and fast 1D convolutions (SCRaWl), in which we can adjust the increase in computational cost by varying the length and number of random walks considered while accounting for higher-order relationships. Importantly, due to the random walk-based design, the expressivity of the proposed architecture is provably incomparable to that of existing message-passing simplicial neural networks. We empirically evaluate SCRaWl on real-world datasets and show that it outperforms other simplicial neural networks.
更多查看译文
关键词
simplicial complex,simplicial neural network,random walks
AI 理解论文
溯源树
样例
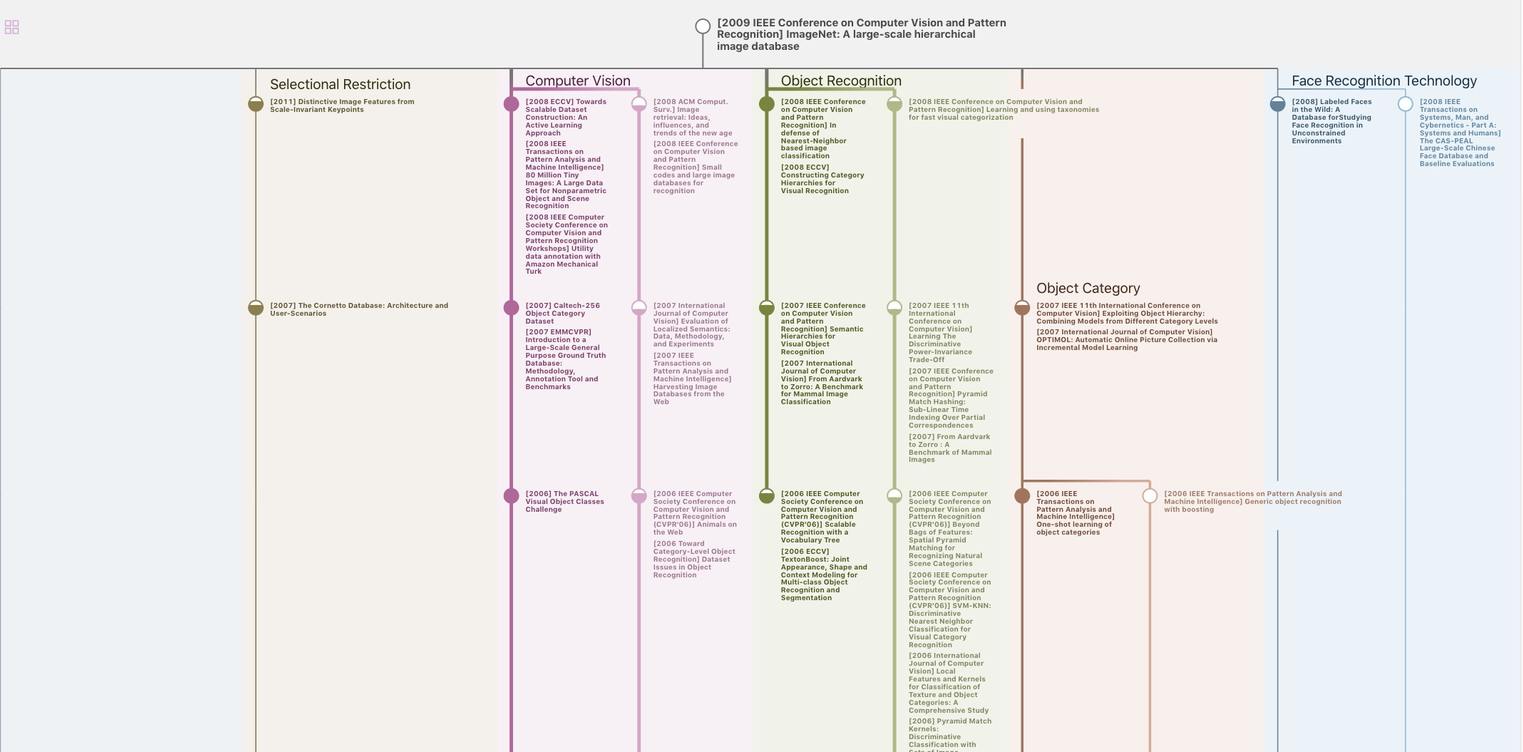
生成溯源树,研究论文发展脉络
Chat Paper
正在生成论文摘要