Debiased Collaborative Filtering with Kernel-based Causal Balancing
ICLR 2024(2024)
摘要
Collaborative filtering builds personalized models from the collected user feedback. However, the collected data is observational rather than experimental, leading to various biases in the data, which can significantly affect the learned model. To address this issue, many studies have focused on propensity-based methods to combat the selection bias by reweighting the sample loss, and demonstrate that
balancing is important for debiasing both theoretically and empirically. However, there are two questions that still need to be addressed: which function class should be balanced and how to effectively balance that function class? In this paper, we first perform theoretical analysis to show the effect of balancing finite-dimensional function classes on the bias of IPS and DR methods, and based on this, we propose a universal kernel-based balancing method to balance functions on the reproducing kernel Hilbert space. In addition, we propose a novel adaptive causal balancing method during the alternating update between unbiased evaluation and training of the prediction model. Specifically, the prediction loss of the model is projected in the kernel-based covariate function space, and the projection coefficients are used to determine which functions should be prioritized for balancing to reduce the estimation bias. We conduct extensive experiments on three real-world datasets to demonstrate the effectiveness of the proposed approach.
更多查看译文
关键词
Recommender System,Causal Inference,Bias,Debias,Balancing
AI 理解论文
溯源树
样例
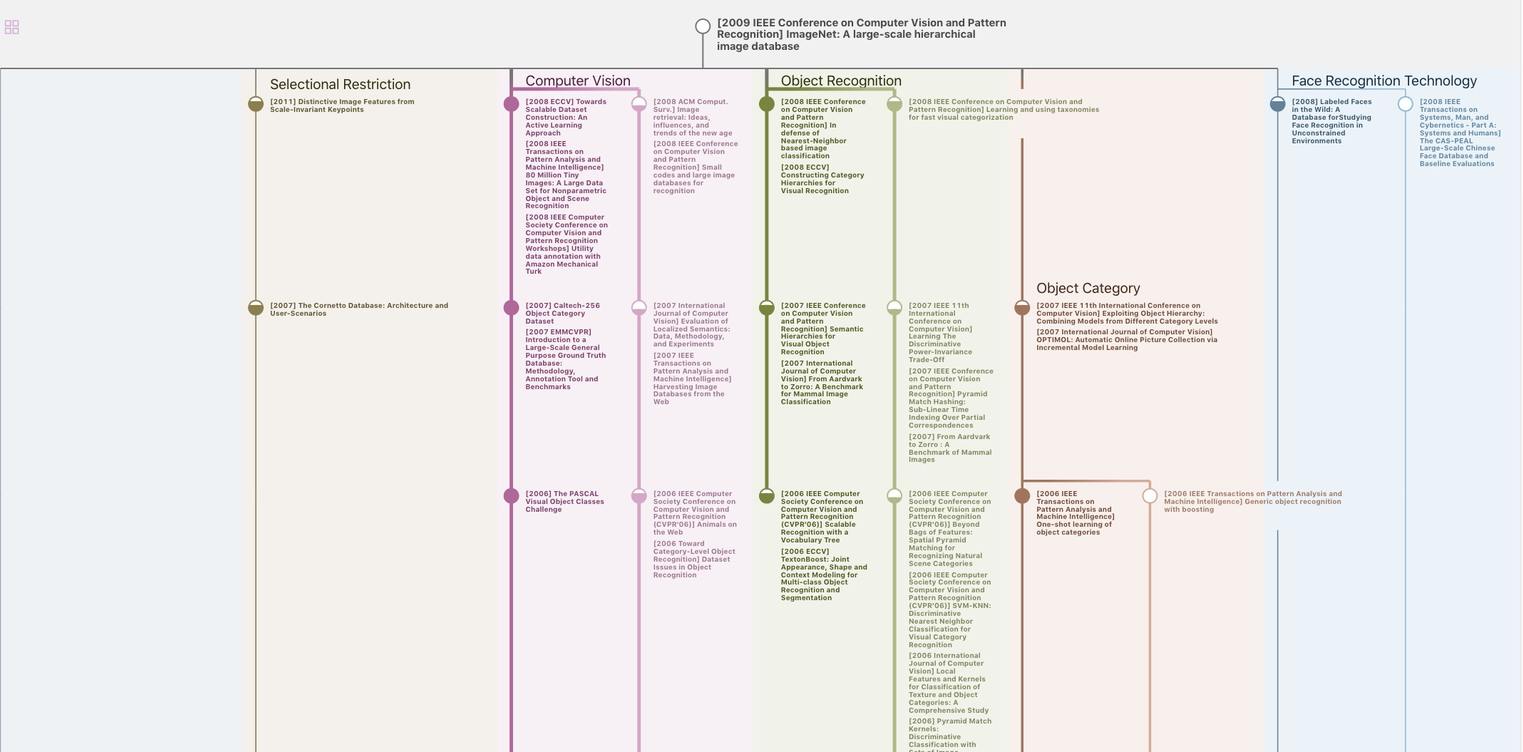
生成溯源树,研究论文发展脉络
Chat Paper
正在生成论文摘要