EQA-MX: Embodied Question Answering using Multimodal Expression
ICLR 2024(2024)
摘要
Humans predominantly use verbal utterances and nonverbal gestures (e.g., eye gaze and pointing gestures) in their natural interactions. For instance, pointing gestures and verbal information is often required to comprehend questions such as "what object is that?" Thus, this question-answering (QA) task involves complex reasoning of multimodal expressions (verbal utterances and nonverbal gestures). However, prior works have explored QA tasks in non-embodied settings, where questions solely contain verbal utterances from a single verbal and visual perspective. In this paper, we have introduced 8 novel embodied question answering (EQA) tasks to develop learning models to comprehend embodied questions with multimodal expressions. We have developed a novel large-scale dataset, EQA-MX, with over 8 million diverse embodied QA data samples involving multimodal expressions from multiple visual and verbal perspectives. To learn salient multimodal representations from discrete verbal embeddings and continuous wrapping of multiview visual representations, we propose a vector-quantization (VQ) based multimodal representation learning model, VQ-Fusion, for the EQA tasks. Our extensive experimental results suggest that VQ-Fusion can improve the performance of existing state-of-the-art visual-language models up to 13% across EQA tasks.
更多查看译文
关键词
multimodal representation learning,visual-language models,embodied question answering
AI 理解论文
溯源树
样例
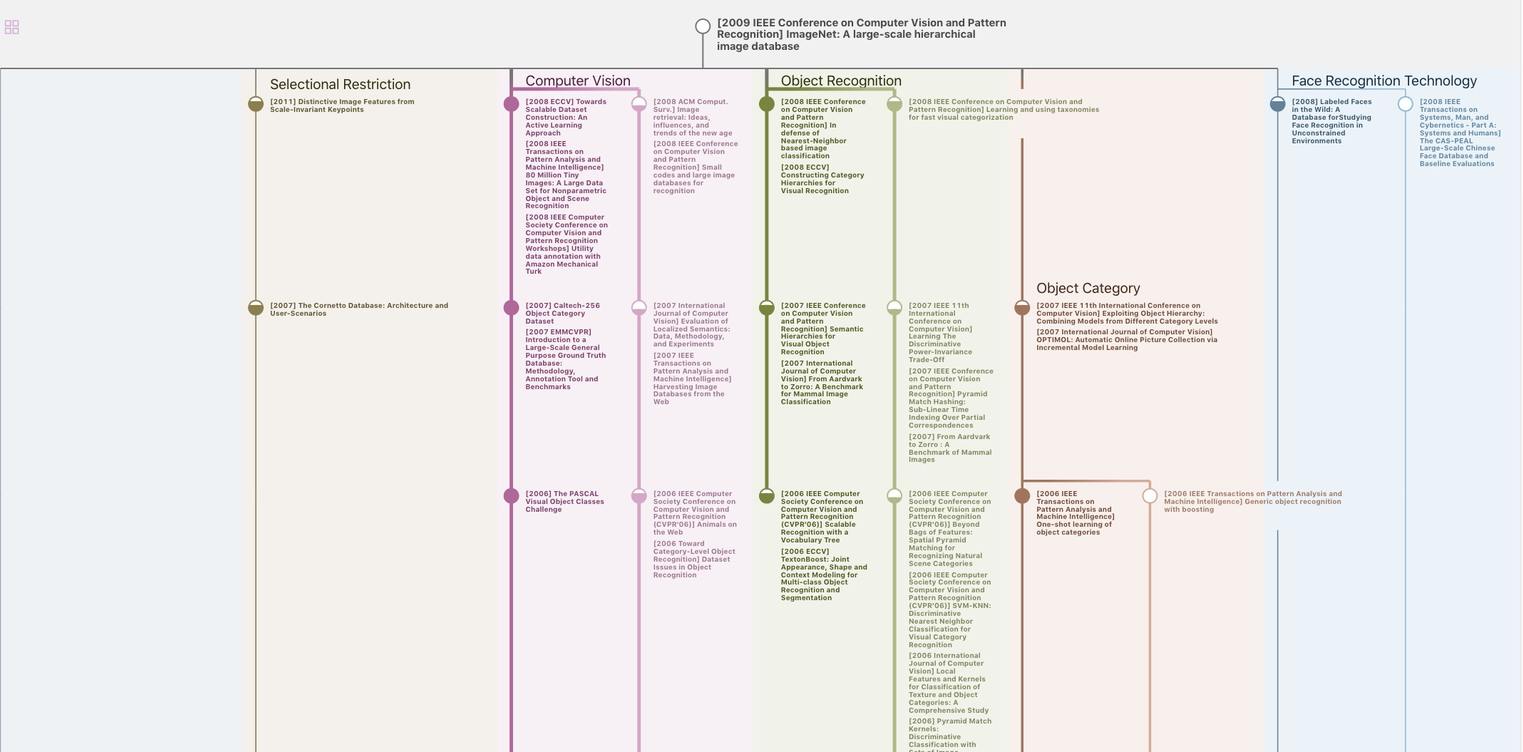
生成溯源树,研究论文发展脉络
Chat Paper
正在生成论文摘要