Variational Bayesian Last Layers
ICLR 2024(2024)
摘要
We introduce a deterministic variational formulation for training Bayesian last layer neural networks. This yields a sampling-free, single-pass model and loss that effectively improves uncertainty estimation. Our variational Bayesian last layer (VBLL) can be trained and evaluated with only quadratic complexity in last layer width, and is thus (nearly) computationally free to add to standard architectures. We experimentally investigate VBLLs, and show that they improve predictive accuracy, calibration, and out of distribution detection over baselines across both regression and classification. Finally, we investigate combining VBLL layers with variational Bayesian feature learning, yielding a lower variance collapsed variational inference method for Bayesian neural networks.
更多查看译文
关键词
bayesian deep learning,variational methods,bayesian last layers,neural linear models
AI 理解论文
溯源树
样例
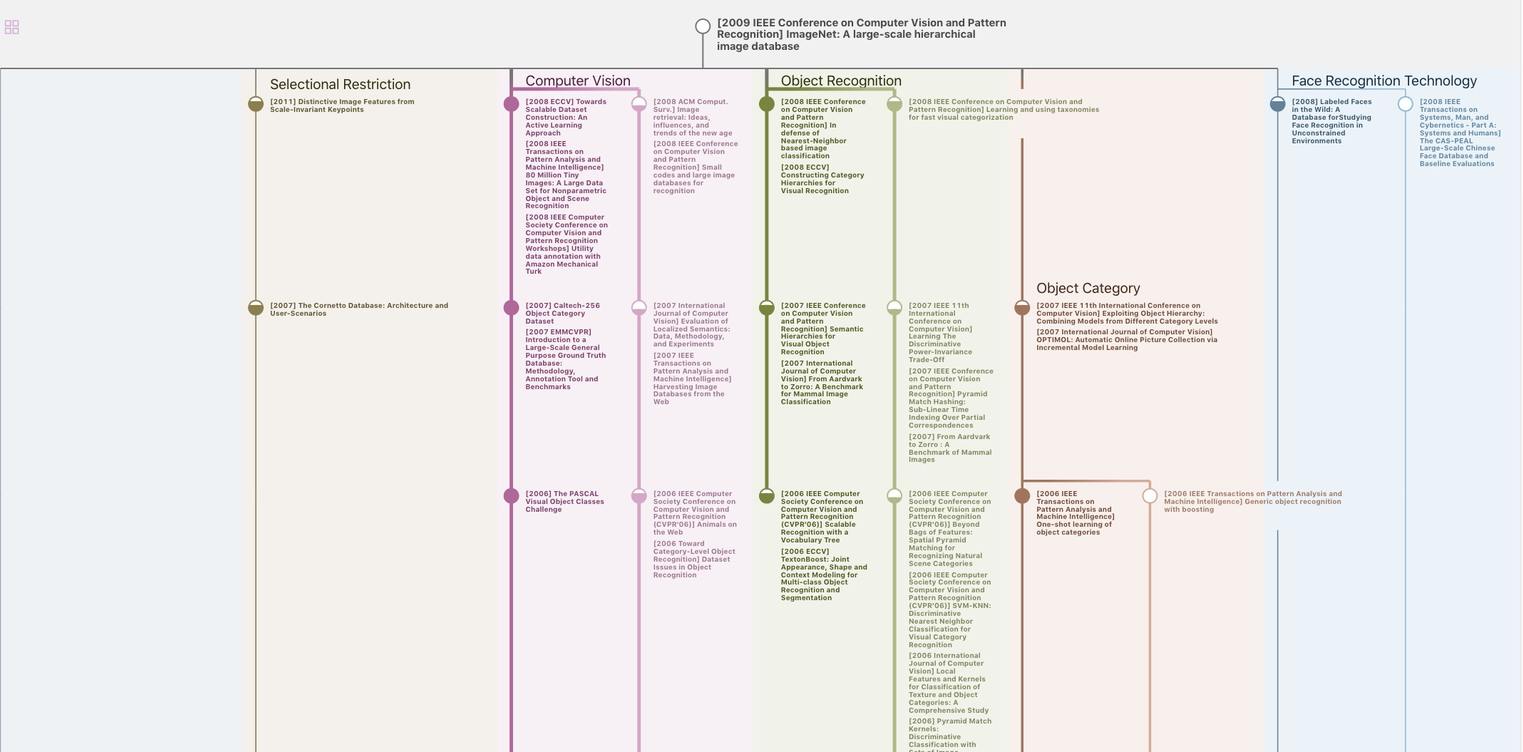
生成溯源树,研究论文发展脉络
Chat Paper
正在生成论文摘要