GPT-4 makes cardiovascular magnetic resonance reports easy to understand
Journal of Cardiovascular Magnetic Resonance(2024)
Abstract
Background
Patients are increasingly using Generative Pre-trained Transformer 4 (GPT-4) to better understand their own radiology findings.
Purpose
To evaluate the performance of GPT-4 in transforming cardiovascular magnetic resonance (CMR) reports into text comprehensible for medical laypersons.
Materials and Methods
ChatGPT with GPT-4 architecture was used to generate three different explained versions of 20 various CMR reports using the same prompt “Explain the radiology report in a language understandable to a medical layperson” (n=60). Two cardiovascular radiologists evaluated understandability, factual correctness, completeness of relevant findings, and lack of potential harm, while 13 medical laypersons evaluated the understandability of the original and the GPT-4 reports on a Likert scale (1 “strongly disagree”, 5 “strongly agree”). Readability was measured using the Automated Readability Index (ARI). Linear mixed-effects models (values given as median [interquartile range]) and intraclass correlation coefficient (ICC) were used for statistical analysis.
Results
GPT-4 reports were generated on average in 52sec ± 13. GPT-4 reports achieved a lower ARI score (10 [9-12] vs 5 [4-6]; p<.001) and were subjectively easier to understand for laypersons than original reports (1 [1-1] vs 4 [4-5]; p<.001). 18/20 (90%) standard CMR reports and 2/60 (3%) GPT-generated reports had an ARI score corresponding to the 8th grade level or higher. Radiologists’ ratings of the GPT-4 reports reached high levels for correctness (5 [4-5]), completeness (5 [5-5]), and lack of potential harm (5 [5-5]); with “strong agreement” for factual correctness in 94% (113/120) and completeness of relevant findings in 81% (97/120) of reports. Test-retest agreement for layperson understandability ratings between the three simplified reports generated from the same original report was substantial (ICC: 0.62; p<.001). Interrater agreement between radiologists was almost perfect for lack of potential harm (ICC: 0.93, p<.001) and moderate to substantial for completeness (ICC: 0.76, p<.001) and factual correctness (ICC: 0.55, p<.001).
Conclusion
GPT-4 can reliably transform complex CMR reports into more understandable, layperson-friendly language while largely maintaining factual correctness and completeness, and can thus help convey patient-relevant radiology information in an easy-to-understand manner.
MoreTranslated text
Key words
Generative pre-trained transformers,cardiovascular magnetic resonance,artificial intelligence,text simplification,large language models
AI Read Science
Must-Reading Tree
Example
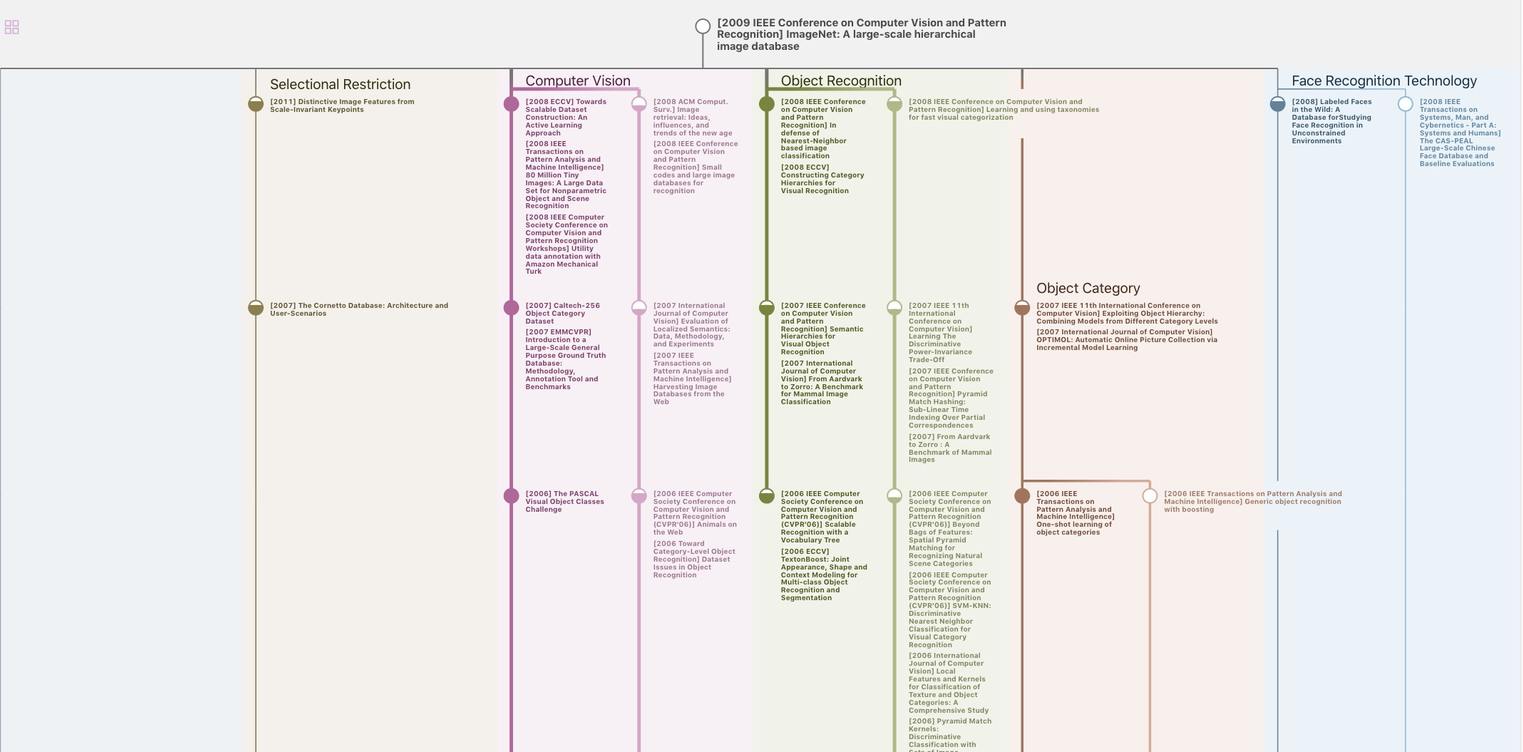
Generate MRT to find the research sequence of this paper
Chat Paper
Summary is being generated by the instructions you defined