DCENet: Diff-Feature Contrast Enhancement Network for Semi-Supervised Hyperspectral Change Detection
IEEE Transactions on Geoscience and Remote Sensing(2024)
Abstract
Multitemporal hyperspectral images (HSIs) have wide applications in change detection (CD) of different land covers for their rich spectral features and image details. Traditional supervised learning-based HSI CD algorithms often rely on a substantial number of labeled samples. However, it requires a significant cost in sample annotation. In this article, we propose a diff-feature contrast enhancement network (DCENet) for semi-supervised HSI CD, which leverages a limited number of labeled samples to guide the training process and a large number of unlabeled samples to improve the confidence of CD. To achieve this, a differential fusion attention (DFA) subnetwork is constructed to extract temporal features from the initial input HSI patches. The dual-branch Siamese enhancement module (SEM) is utilized to enhance the generalization of differential features in the feature maps. Herein, multiscale Kullback-Leibler (KL) divergence and feature-enhanced probabilistic contrast loss are designed to constrain the SEM. The proposed method excels at detecting subtle changes in bitemporal HSIs simultaneously improving the generalization performance of networks. The visual and quantitative experimental results on four HSI datasets show that the proposed DCENet outperforms the compared state-of-the-art methods for HSI CD. Codes are available at https://github.com/Zhoutya/ChangeDetection-DCENet.
MoreTranslated text
AI Read Science
Must-Reading Tree
Example
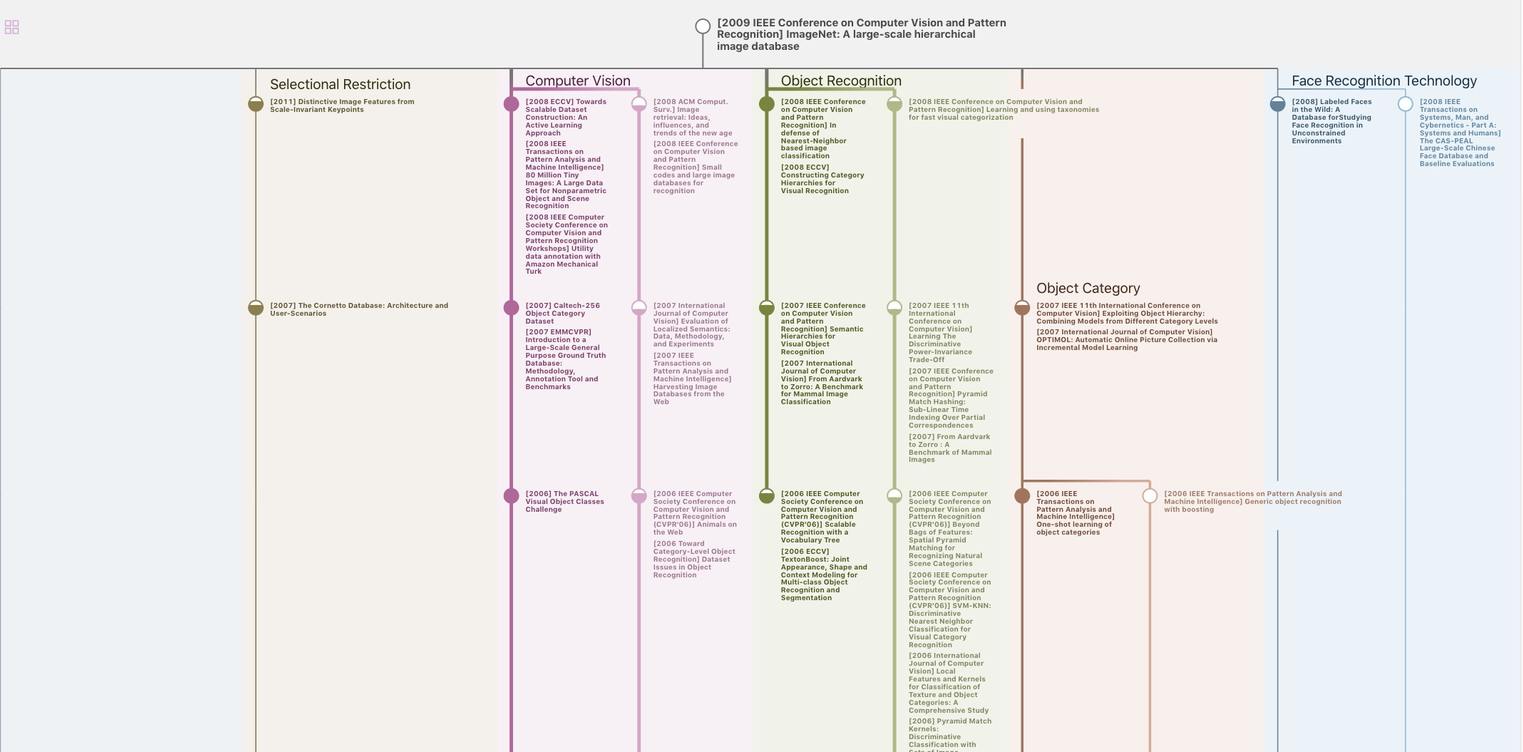
Generate MRT to find the research sequence of this paper
Chat Paper
Summary is being generated by the instructions you defined