Gabor-Filtered Fourier Neural Operator for solving Partial Differential Equations
COMPUTERS & FLUIDS(2024)
摘要
The Fourier Neural Operator (FNO) solves a family of Partial Differential Equations (PDEs) by learning the nonlinear mapping from the parameter space to the solution space. In this study, we enhance FNO by integrating a learnable Gabor -Filtered module, leading to the Gabor -Filtered Fourier Neural Operator (GFNO). The frequency transformation matrix is pivotal to the expressiveness of FNO. In the proposed Gabor -Filtered module, the parametric Gabor filters provide regularization to the elements of the frequency transformation matrix, and it assigns the higher weights to the key frequencies in the frequency domain. This enables the frequency transformation matrix to reduce redundancies and emphasize the key frequency bands. Our evaluation, covering five different PDEs and a Climate modeling challenge, shows that GFNO outperforms the original FNO. Compared with FNO, GFNO has average error reductions of 8.3%, 26.3%, and 28.7% on solving 1-d Burgers' equation, 2-d Darcy Flow equation, and 3-d (2-d + time) Navier-Stokes equation, respectively. As a version of GFNO with fewer number of feature channels, GFNO-small uses merely 19.5%, 39.1%, and 64.1% of the number of parameters of FNO. Despite having fewer parameters, GFNO-small reduces errors by 24.9%, 21.3%, and 16.3% compared to FNO in solving the three mentioned equations, respectively. The code and datasets are available at https://github.com/Kai-Qi/Gabor-Filtered-Fourier-Neural-Operator.
更多查看译文
关键词
Gabor filters,Regularization in frequency domain,Neural operator,Partial differential equations
AI 理解论文
溯源树
样例
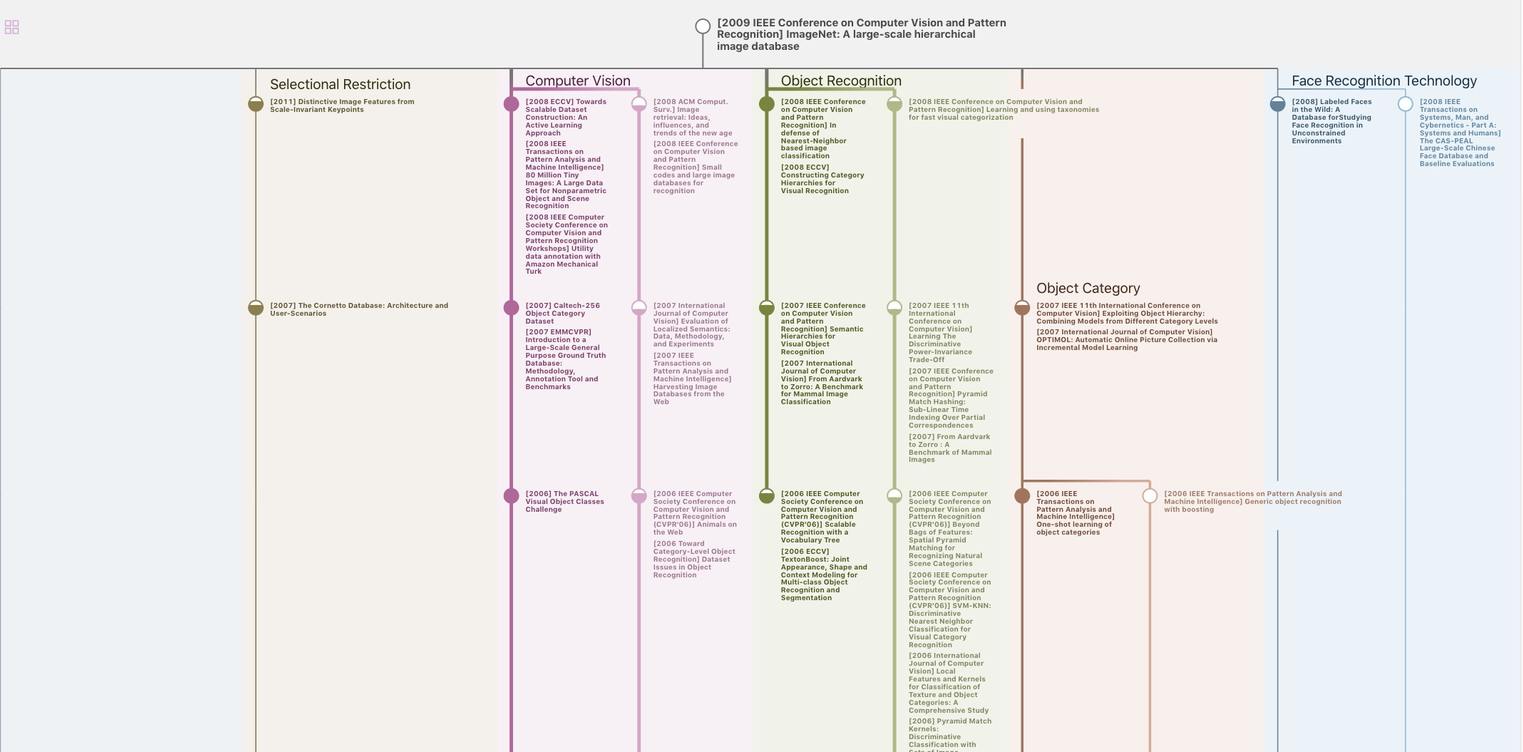
生成溯源树,研究论文发展脉络
Chat Paper
正在生成论文摘要