Discriminative Sample-Guided and Parameter-Efficient Feature Space Adaptation for Cross-Domain Few-Shot Learning
CoRR(2024)
摘要
In this paper, we look at cross-domain few-shot classification which presents
the challenging task of learning new classes in unseen domains with few
labelled examples. Existing methods, though somewhat effective, encounter
several limitations, which we address in this work through two significant
improvements. First, to address overfitting associated with fine-tuning a large
number of parameters on small datasets, we introduce a lightweight
parameter-efficient adaptation strategy. This strategy employs a linear
transformation of pre-trained features, significantly reducing the trainable
parameter count. Second, we replace the traditional nearest centroid classifier
with a variance-aware loss function, enhancing the model's sensitivity to the
inter- and intra-class variances within the training set for improved
clustering in feature space. Empirical evaluations on the Meta-Dataset
benchmark showcase that our approach not only improves accuracy up to 7.7
5.3
performance while being at least 3x more parameter-efficient than existing
methods, establishing a new state-of-the-art in cross-domain few-shot learning.
Our code can be found at https://github.com/rashindrie/DIPA.
更多查看译文
AI 理解论文
溯源树
样例
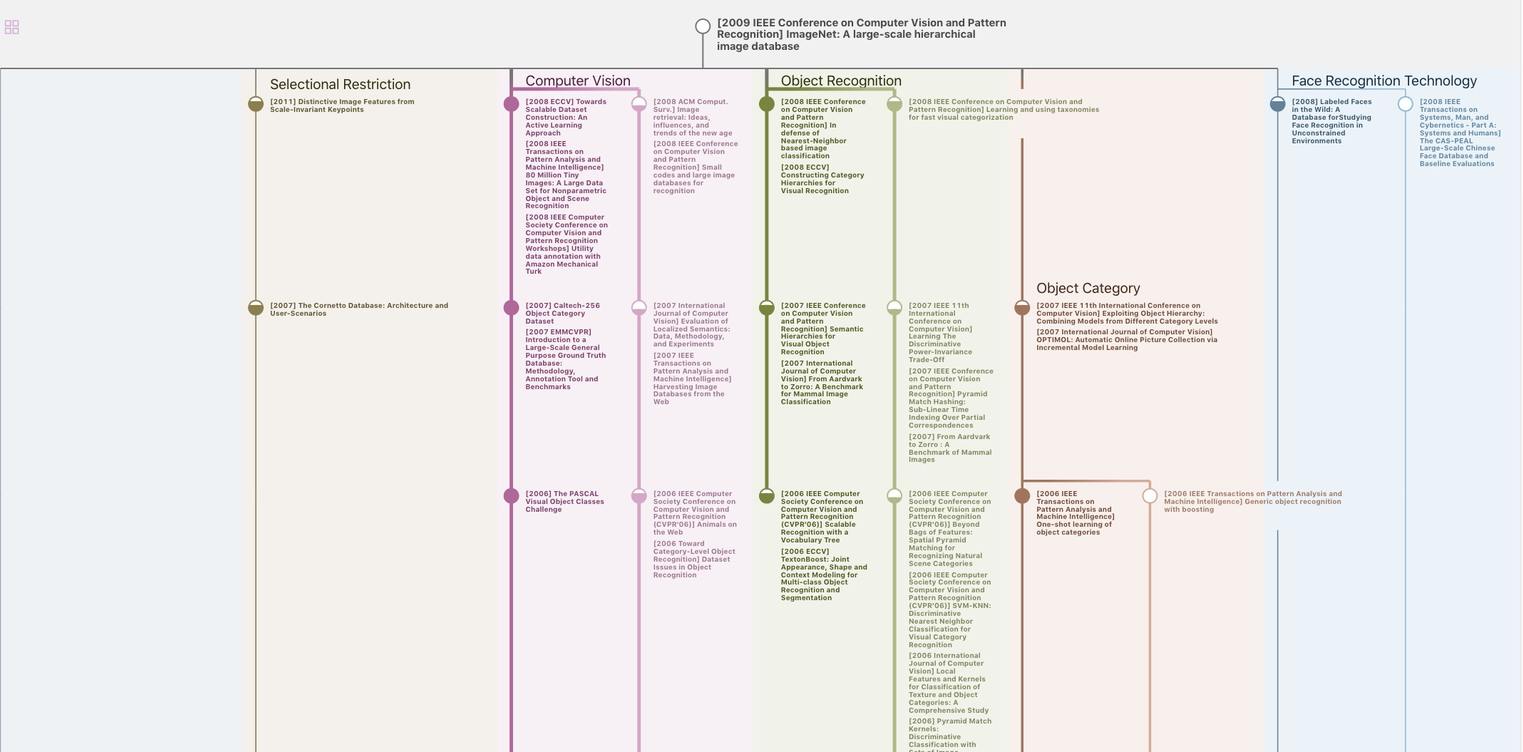
生成溯源树,研究论文发展脉络
Chat Paper
正在生成论文摘要