ASTKD-PCB-LDD:A Lightweight High Performance PCB Defect Detection Algorithm
crossref(2024)
Abstract
Abstract The YOLOv5 algorithm, widely utilized in printed circuit board (PCB) defect detection, undergoes a reduction in speed when deployed on compact industrial computing platforms due to its substantial parameter count. Consequently, this study proposes a lightweight and high-performance PCB defect detection model based on YOLOv5, named ASTKD-PCB-LDD.Employing the k-means++ algorithm for optimal anchor boxes and the SCYLLA-IoU(SIoU) loss function over the Complete IoU(CIoU) loss function, the model enhances accuracy in locating and detecting small-target defects.It designs the Faster-Ghost lightweight backbone network to replace the YOLOv5 backbone network, and employs a slim-neck neck architecture to supersede the YOLOv5 neck network, which significantly reduces computational requirements and enhances inference speed, yielding a more efficient PCB-LDD network. The align soft-target knowledge distillation(ASTKD) approach is crafted to employ the PCB-LDD model as the teacher and the pruned model (via the Layer-Adaptive Magnitude-based Pruning(LAMP)) as the student, aiming to preserve detection accuracy while reducing parameters. Experimental results demonstrate that the model size has been reduced from 14.5MB to 4MB, achieving a compression rate of 27.6%; the mean average precision (mAP) achieves 98%, and the detection speed is enhanced from 73.2 frames/s to 112.3 frames/s, resulting in a speedup of 153.4%. This approach successfully achieves the goal of combining high performance with lightweight design, significantly enhancing the accuracy and efficiency of PCB defect detection.
MoreTranslated text
AI Read Science
Must-Reading Tree
Example
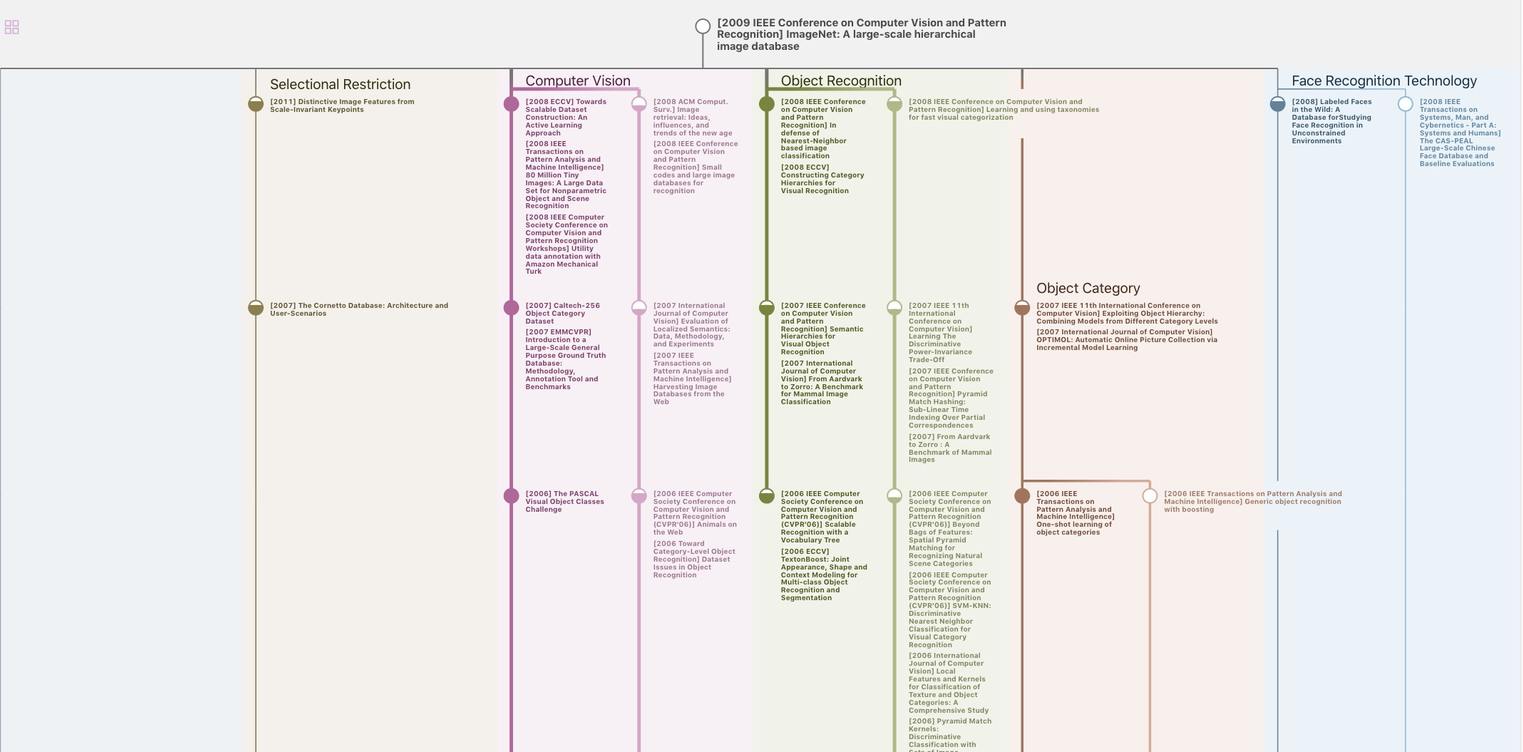
Generate MRT to find the research sequence of this paper
Chat Paper
Summary is being generated by the instructions you defined