Crafting Disability Fairness Learning in Data Science: A Student-Centric Pedagogical Approach
PROCEEDINGS OF THE 55TH ACM TECHNICAL SYMPOSIUM ON COMPUTER SCIENCE EDUCATION, SIGCSE 2024, VOL. 1(2024)
摘要
Ensuring the fairness of machine learning (ML) systems for individuals with disabilities is crucial. Proactive measures are required to identify and mitigate biases in data and models, thereby preventing potential harm or bias against people with disabilities. While previous research on ML fairness education primarily concentrated on gender and race fairness, the domain of disability fairness has received comparatively little attention. Addressing this gap, we adopted a student-centric approach to craft a disability fairness teaching intervention. A focus group of students experienced in ML and accessible computing underscored the significance of engagement and scaffolding strategies for effectively learning intricate topics. Consequently, we crafted a disability fairness hands-on programming assignment that delves into uncovering disability bias with a lens that takes intersectionality into account. The assignment was tailored for an introductory undergraduate data science (DS) course. We employed reflective questions and surveys to gauge the effectiveness of our approach. The findings indicate the success of our approach in promoting a deeper understanding of disability fairness within the context of DS education.
更多查看译文
关键词
Fairness,disability,data science,machine learning,CS education
AI 理解论文
溯源树
样例
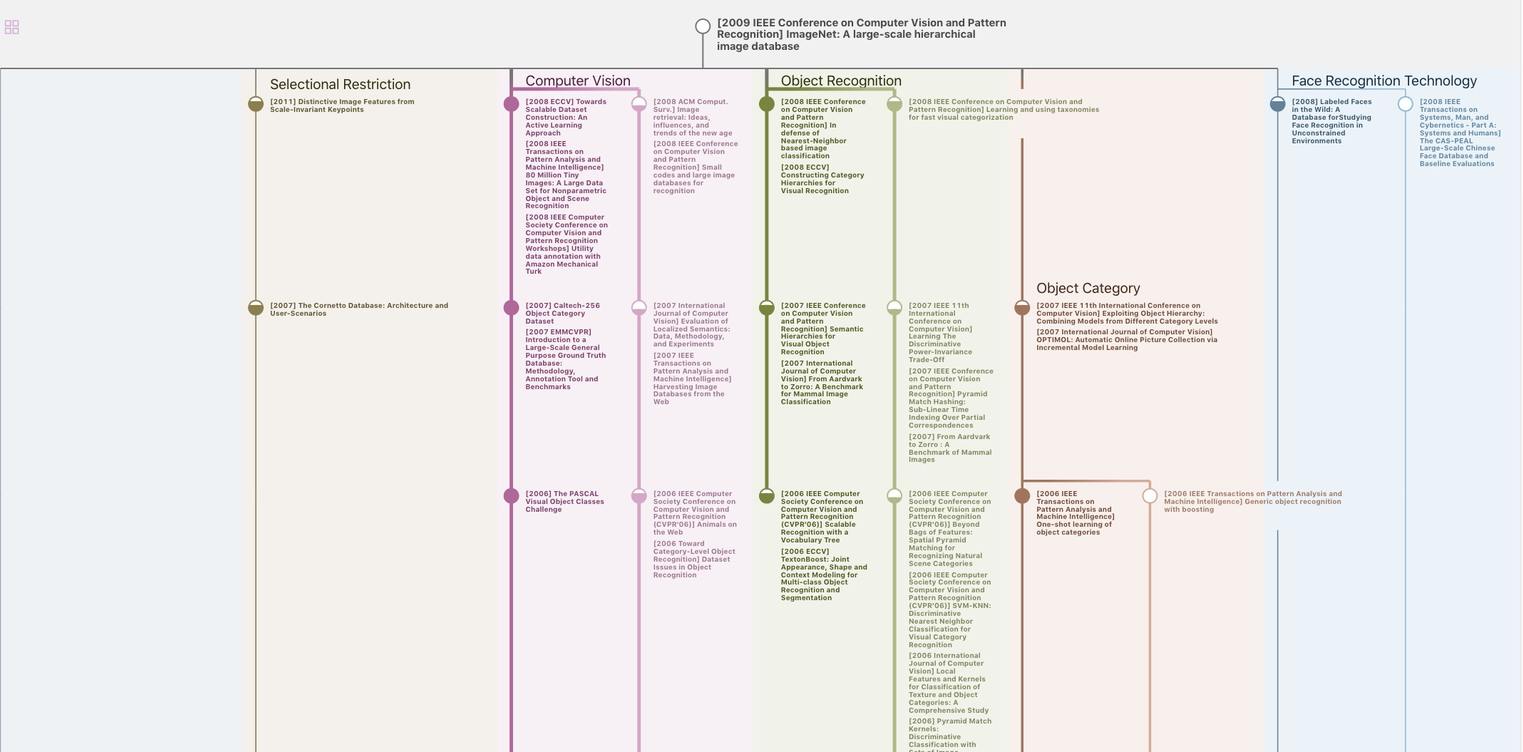
生成溯源树,研究论文发展脉络
Chat Paper
正在生成论文摘要