Interpretable Siamese dual attention enhancement transfer compound diagnostic model for unbalanced samples
STRUCTURAL HEALTH MONITORING-AN INTERNATIONAL JOURNAL(2024)
Abstract
The intelligent transfer diagnosis model is used to address the issue of feature drift caused by the changing working conditions of rotating parts in engineering. However, few models can perform transfer diagnosis on multiple unbalanced samples of rotating parts simultaneously, and even fewer models can visually enhance the domain-invariant features, making them more interpretable. To address these issues, we propose a novel interpretable Siamese dual attention enhancement transfer compound diagnosis model for unbalanced samples. The model can diagnose multiple rotating parts simultaneously and consists of a channel feature attention enhancement (CFAE) network, a fragment feature attention enhancement (FFAE) network, and a Siamese feature fusion (SFF) network. The CFAE network enhances features of different convolutional channels, the FFAE network improves segment features in various frequency domains, and the SFF network extracts domain-invariant features of diverse rotating components under varying working conditions. The model is validated using bearing fault data collected under different loads and planetary gear fault data obtained at varying speeds. Its diagnostic accuracy remains above 96.4%, and the diagnostic variance is controlled within 1.0%. The model has good interpretability for imbalanced sample domain-invariant features, providing an effective tool for interpretable transfer diagnosis in this compound engineering situation.
MoreTranslated text
Key words
Transfer diagnosis,unbalanced samples,interpretable,Siamese,attention enhancement,visualization
AI Read Science
Must-Reading Tree
Example
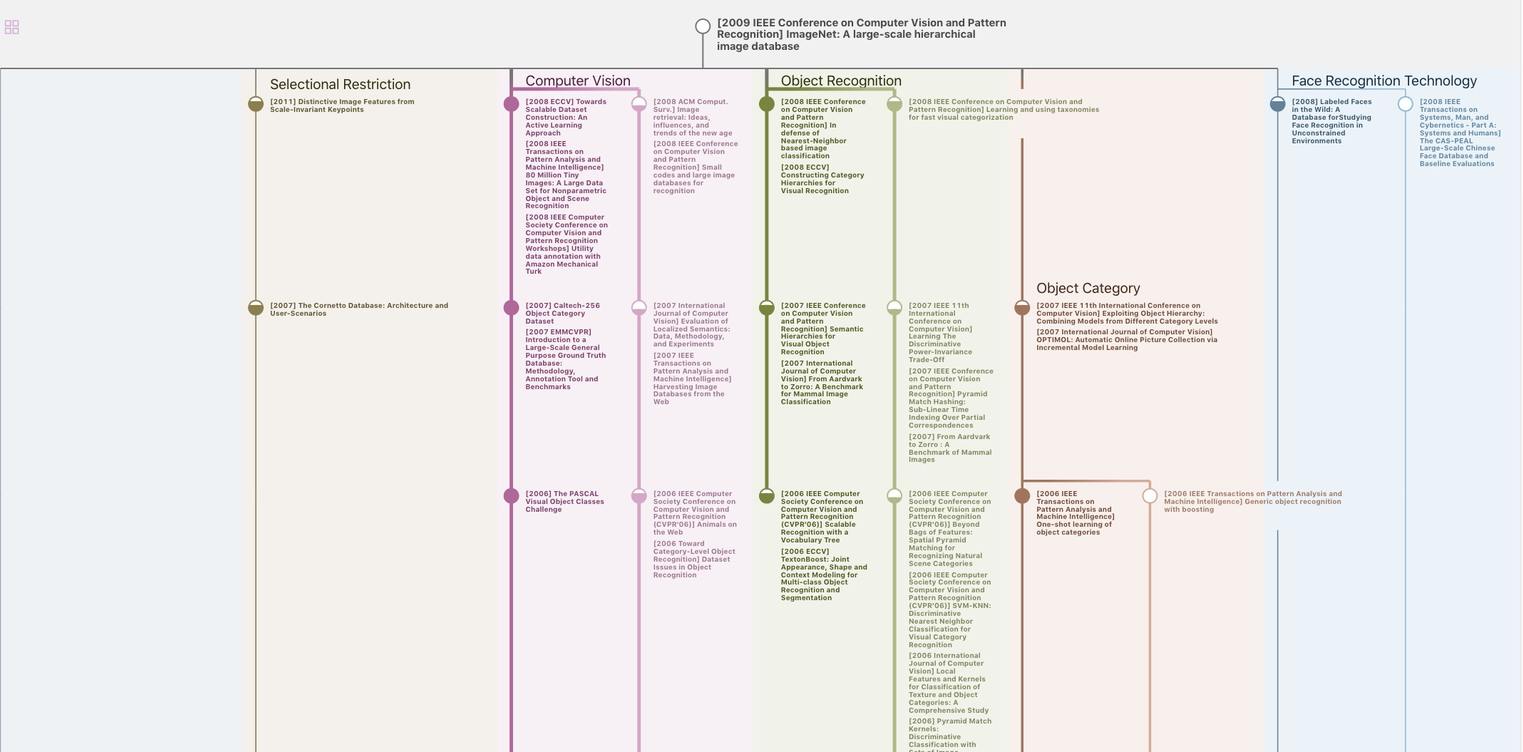
Generate MRT to find the research sequence of this paper
Chat Paper
Summary is being generated by the instructions you defined