Feature Adaptation for Landslide Susceptibility Assessment in “no Sample” Areas
Gondwana research(2024)
摘要
Given the time-consuming nature of compiling landslide inventories, it is increasingly important to develop transferable landslide susceptibility models that can be applied to regions without existing data. In this study, we propose a feature-based domain adaptation method to improve the transferability of landslide susceptibility models, especially in “no sample” areas. Two typical landslide-prone areas in Fujian province, southeastern China, were chosen as research cases to test the practicality of the transfer effect. Five conventional machine learning algorithms (Support vector machines (SVM), Random Forest (RF), Logistic Regression (LOG), K-nearest neighbor (KNN), and Decision tree (C4.5)) are used to model landslide susceptibility in sampled areas (source domain), and a feature transfer-based landslide susceptibility evaluation model is constructed under coupled feature transfer methods to evaluate the susceptibility of landslide in un-sampled areas (target domain). The results showed that feature transfer can effectively improve the transferability of different machine learning models for cross-regional prediction (The indicators have improved overall by 8.49%), with SVM (increased by 13.68%) and LOG (increased by 10.19%) models showing the most significant improvements. The feature-based domain adaptive method can alleviate the burden of collecting and labeling new data, and effectively improve the assessment performance of machine learning-based landslide susceptibility models in un-sampled areas. This is a new solution for landslide susceptibility assessment in completely “no sample” areas.
更多查看译文
关键词
Landslide susceptibility,Machine learning,Feature domain adaptation,Reservoir bank slope,Data scarcity
AI 理解论文
溯源树
样例
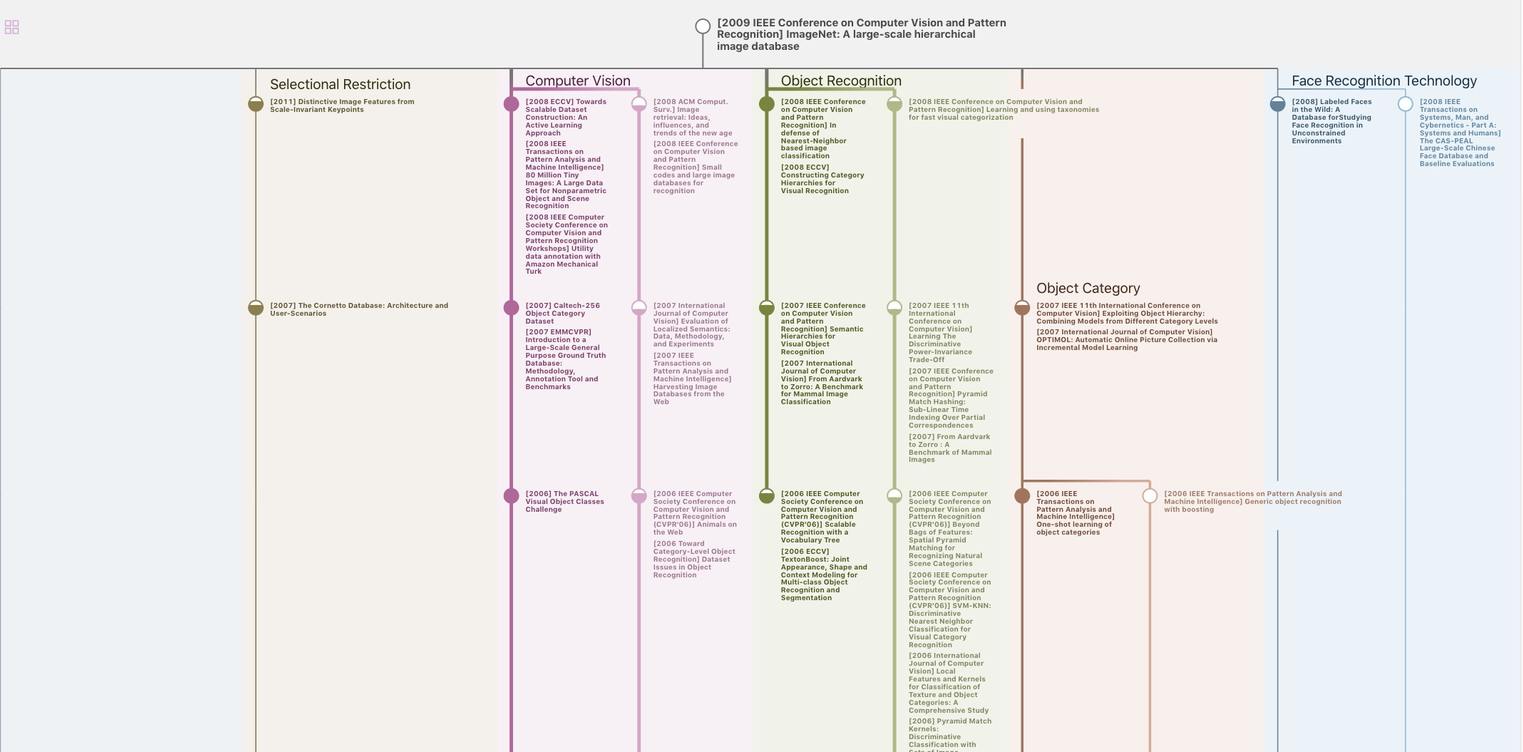
生成溯源树,研究论文发展脉络
Chat Paper
正在生成论文摘要