Variable Correlation Analysis-Based Convolutional Neural Network for Far Topological Feature Extraction and Industrial Predictive Modeling.
IEEE Trans. Instrum. Meas.(2024)
摘要
In process industries, accurate prediction of critical quality variables is particularly important for process control and optimization. Usually, soft sensors have been developed to estimate the quality variables via process variables. However, there are often process variables that are far apart in topology but have high correlations, presenting challenges for such feature learning in soft sensor. To solve these difficulties, a variable correlation analysis based convolutional neural network (VCA-CNN) is proposed in this paper for far topological feature extraction, which generates three-channel data samples containing natural topology features, linear topology features, and nonlinear topology features through Pearson and Spearman correlation to adequately focus on far-topology features. In the feature extraction stage, the VCA-CNN employs a special row convolution kernel to extract far-topological features. Then, normal convolutional and pooling operations are alternately processed on the output feature maps. This approach overcomes the limitation that traditional convolutional operations mainly extract local correlations of process variables. The effectiveness and superiority of the VCA-CNN are validated in the hydrocracking process and debutanizer column.
更多查看译文
关键词
Soft sensor,Quality prediction,Convolutional neural network,Variable correlation analysis
AI 理解论文
溯源树
样例
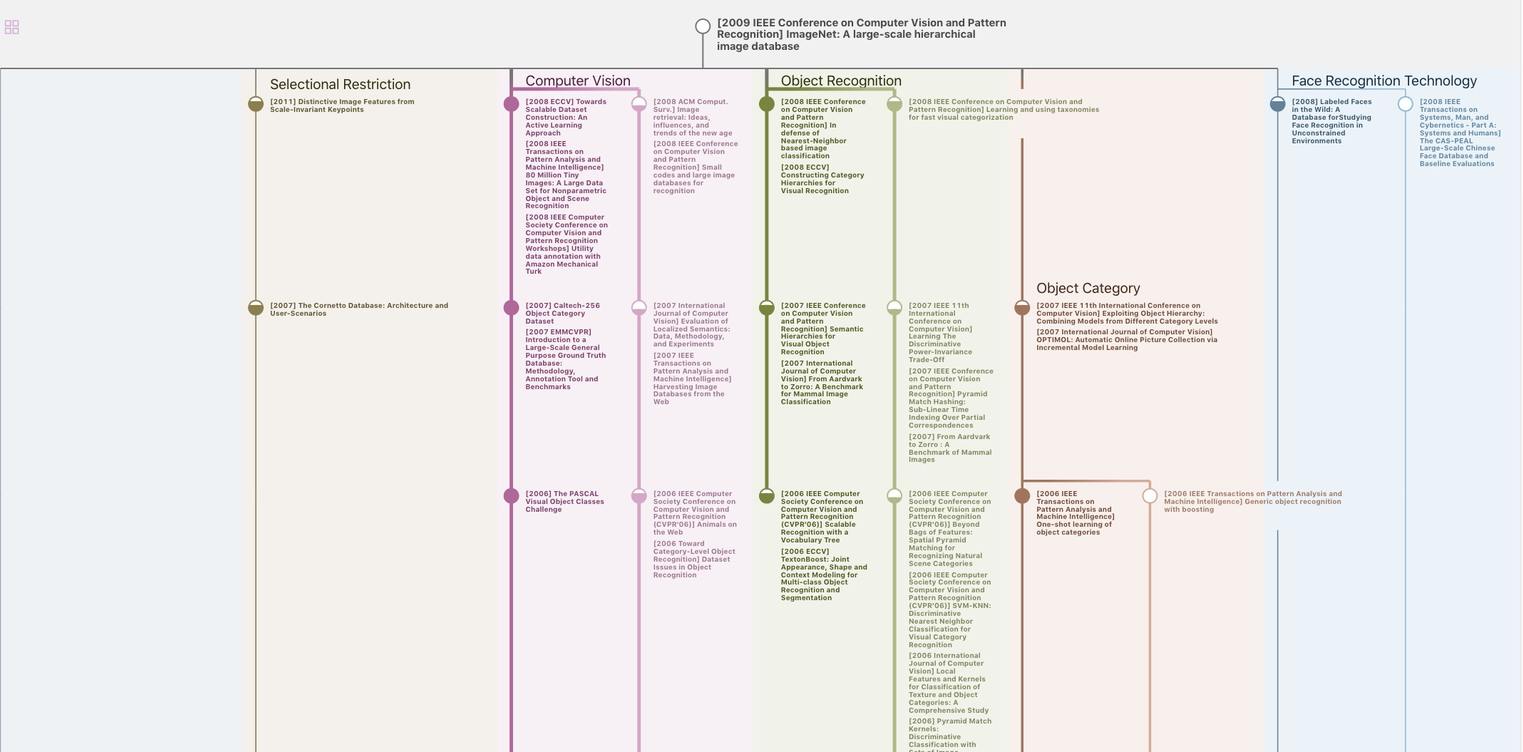
生成溯源树,研究论文发展脉络
Chat Paper
正在生成论文摘要