Toward Memristive Phase‐Change Neural Network with High‐Quality Ultra‐Effective Highly‐Self‐Adjustable Online Learning
Advanced Physics Research(2024)
Abstract
Abstract Memristive hardware with reconfigurable conductance levels are leading candidates for achieving artificial neural networks (ANNs). However, owing to difficulties in device character design and circuit combination, the ability to perform complicated online‐learning tasks on a memristive network is not well understood. Here, tandem (T) material states are harnessed in a phase‐change memory (PCM) element, i.e., the primed‐amorphous state and the partial‐crystallized state, by utilizing an impetus‐and‐consequent pair pulse through a large degree of configurational ordering, and illustrate the development of an integrated system for achieving in‐memory computing and neural networks (NNs). A correct classification of 96.1% of 10,000 separate test images from the conventional Modified‐National‐Institute‐of‐Standards‐and‐Technology (MNIST) database in the tandem neural‐network (T‐NN) model is achieved, as well as image recognition for 28×28‐pixel pictures. The T‐NN configuration exhibits an in situ learning, with 50% of the elements stuck in the low‐conductance state, and at the same time, maintains an identification accuracy of ≈90%. The structural origin of the large degree of configurational‐ordering‐enhanced improvement in the extent of the conductance uniformity in the T‐based memristive element is revealed by theoretical studies. This work opens the door for attaining a widely relevant hardware system capable of performing artificial intelligence tasks with a large power‐time efficacy.
MoreTranslated text
Key words
artificial neural network,image classification,in situ online learning,memristive devices,phase‐change‐memory materials
AI Read Science
Must-Reading Tree
Example
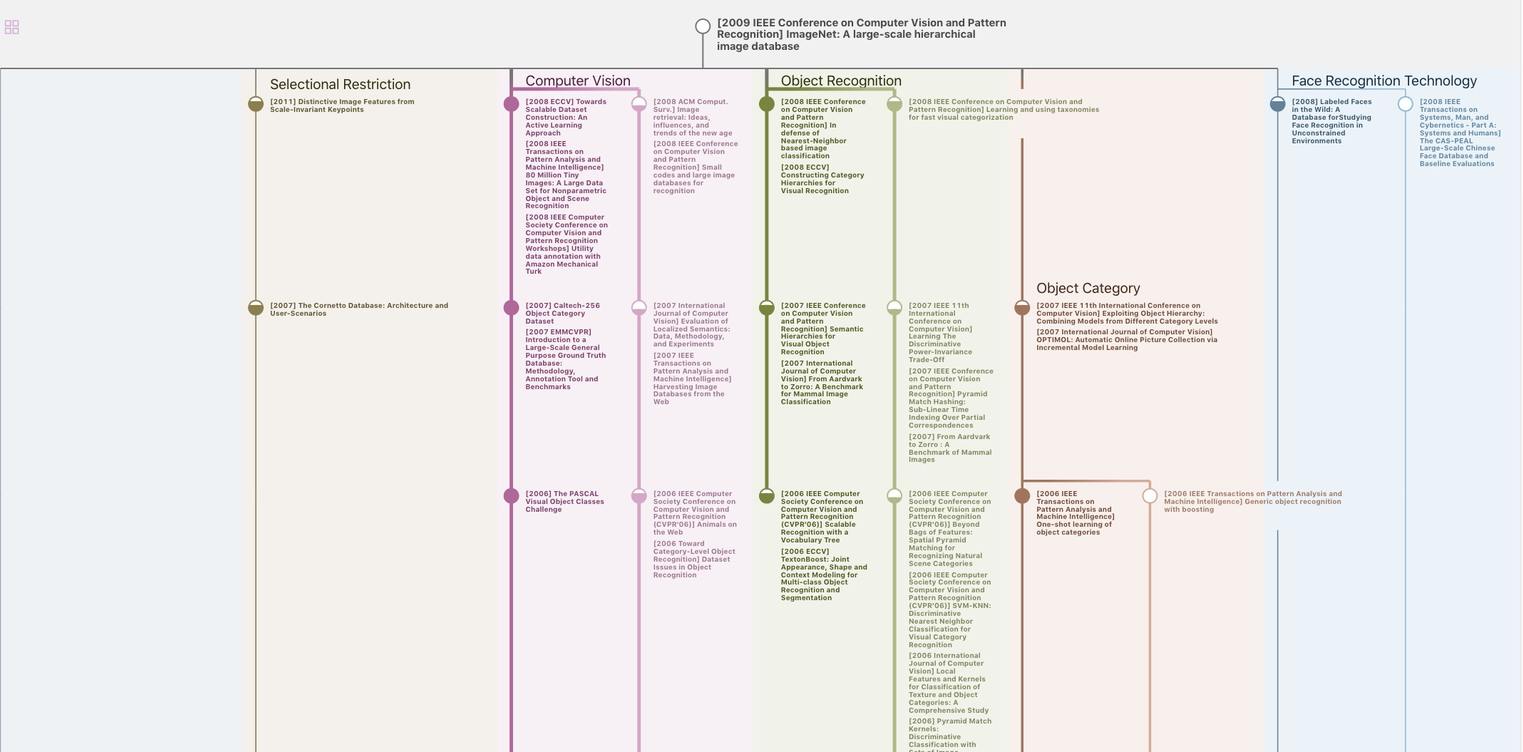
Generate MRT to find the research sequence of this paper
Chat Paper
Summary is being generated by the instructions you defined