GAM: General affordance-based manipulation for contact-rich object disentangling tasks
NEUROCOMPUTING(2024)
摘要
Picking up an entangled object is a difficult manipulation task due to its rich contact dynamics. Most existing solutions fail to produce grasp poses to enable reliable manipulation due to the dependence on simplified assumptions for the motion policies. Grasps generated by these methods tend to drop objects or cause undesired movements of non-grasped objects. To improve such object-disentangling tasks, we propose to extend the concept of reinforcement learning (RL)-based affordance to include arbitrary action consequences and implement a general affordance-based manipulation (GAM) framework. In the GAM, we train an RL agent that uses more fine-grained actions and outperforms previous methods with a smaller chance of dropping objects and making contact with non-grasped hooks. Then, a manipulation affordance prediction (MAP) model is trained to estimate the performances of the RL agent. Finally, the manipulation affordance-based grasp filter (MAGF) selects grasp poses that afford the desired manipulation performances, showing substantial improvements in five challenging hook disentangling tasks in simulation. The experiments show (1) the limitation of TAG generators, (2) the effectiveness of filtering TAGs with predicted manipulation performances based on the general affordance theory, and (3) the importance of avoiding contact with non-grasped objects in contact-rich manipulation.
更多查看译文
关键词
Contact-rich manipulation,Affordance theory,Reinforcement learning,Task-oriented grasping,Grasp filter
AI 理解论文
溯源树
样例
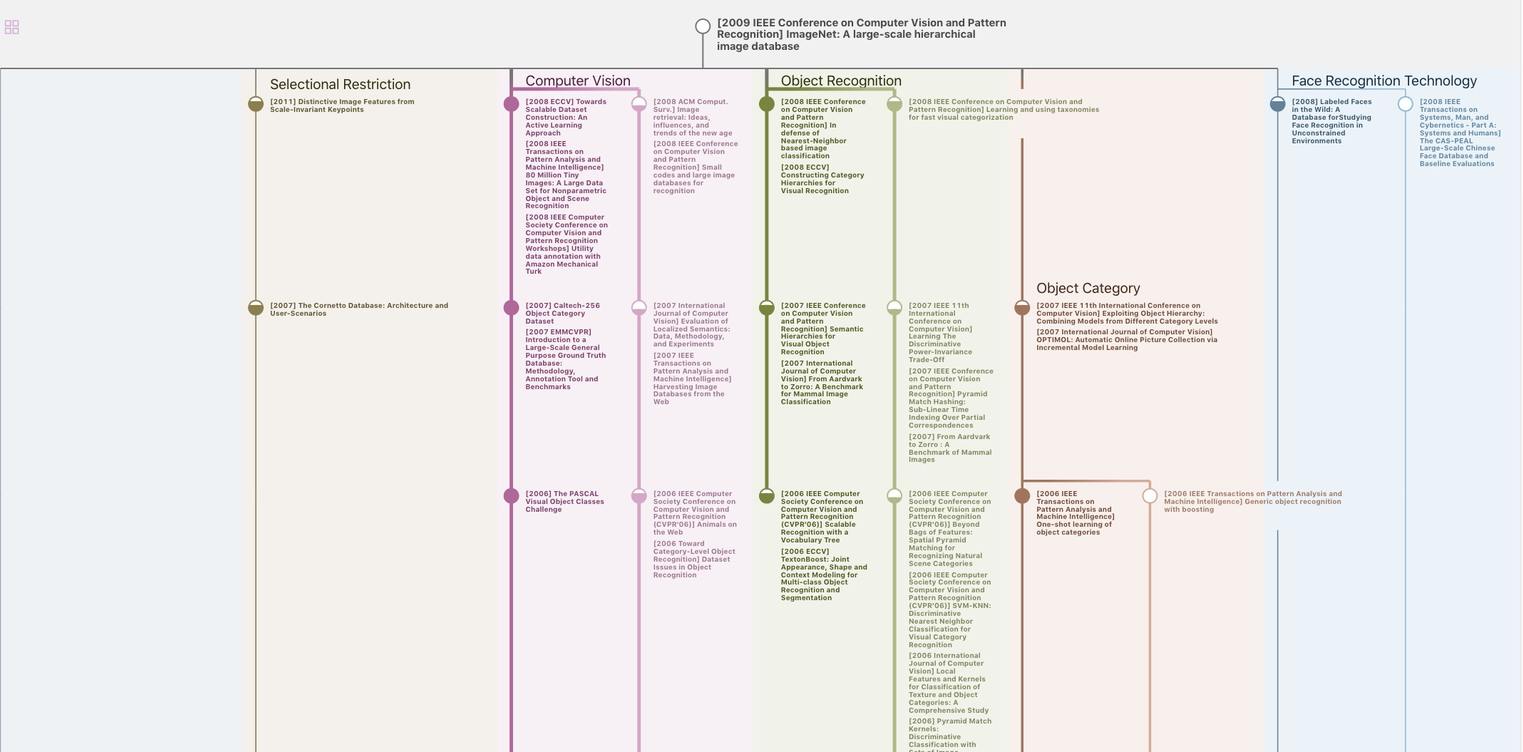
生成溯源树,研究论文发展脉络
Chat Paper
正在生成论文摘要