Change Detection in Remote-Sensing Images Using Pyramid Pooling Dynamic Sparse Attention Network With Difference Enhancement
IEEE JOURNAL OF SELECTED TOPICS IN APPLIED EARTH OBSERVATIONS AND REMOTE SENSING(2024)
摘要
Benefits from the powerful local modeling capability of deep convolutional neural networks (CNNs), remote-sensing image change detection (CD) has made significant progress. In recent years, the rise of transformers has further driven improvements in global feature extraction for bitemporal remote-sensing images. Some prior efforts have tried to integrate CNN and transformer, but they suffer from the limitation of inefficiently aggregating local features and contextual information. Besides, they struggle to refine change boundaries and exhibit inferior performance in detecting multiscale and subtle changes. To tackle these interrelated problems, we propose a difference-enhanced pyramid pooling dynamic sparse attention network (DPDANet) for CD, which integrates the potential of CNN and pyramid pooling dynamic sparse attention (PDSA) mechanism. Specifically, a pretrained EfficientNetV2-S network is first used to extract multilevel local fine-grained features. Then, a global semantic enhancement network based on well-designed PDSA mechanism is proposed to extract rich global contextual information. The proposed difference enhancement module combines long short-term memory and deformable convolution to emphasize relevant and irrelevant changes, capturing precise boundary details of the changing region. A decoder is then employed for step-by-step upsampling of encoded features, with skip connections between local multiscale features and globally enhanced features. Expensive experiments on four public CD datasets demonstrate that DPDANet outperforms state-of-the-art methods by reducing missed detections and false detections and achieving more accurate boundaries of the changing area.
更多查看译文
关键词
Feature extraction,Transformers,Semantics,Remote sensing,Computational modeling,Convolution,Data mining,Attention mechanism,change detection (CD),deep learning,remote sensing
AI 理解论文
溯源树
样例
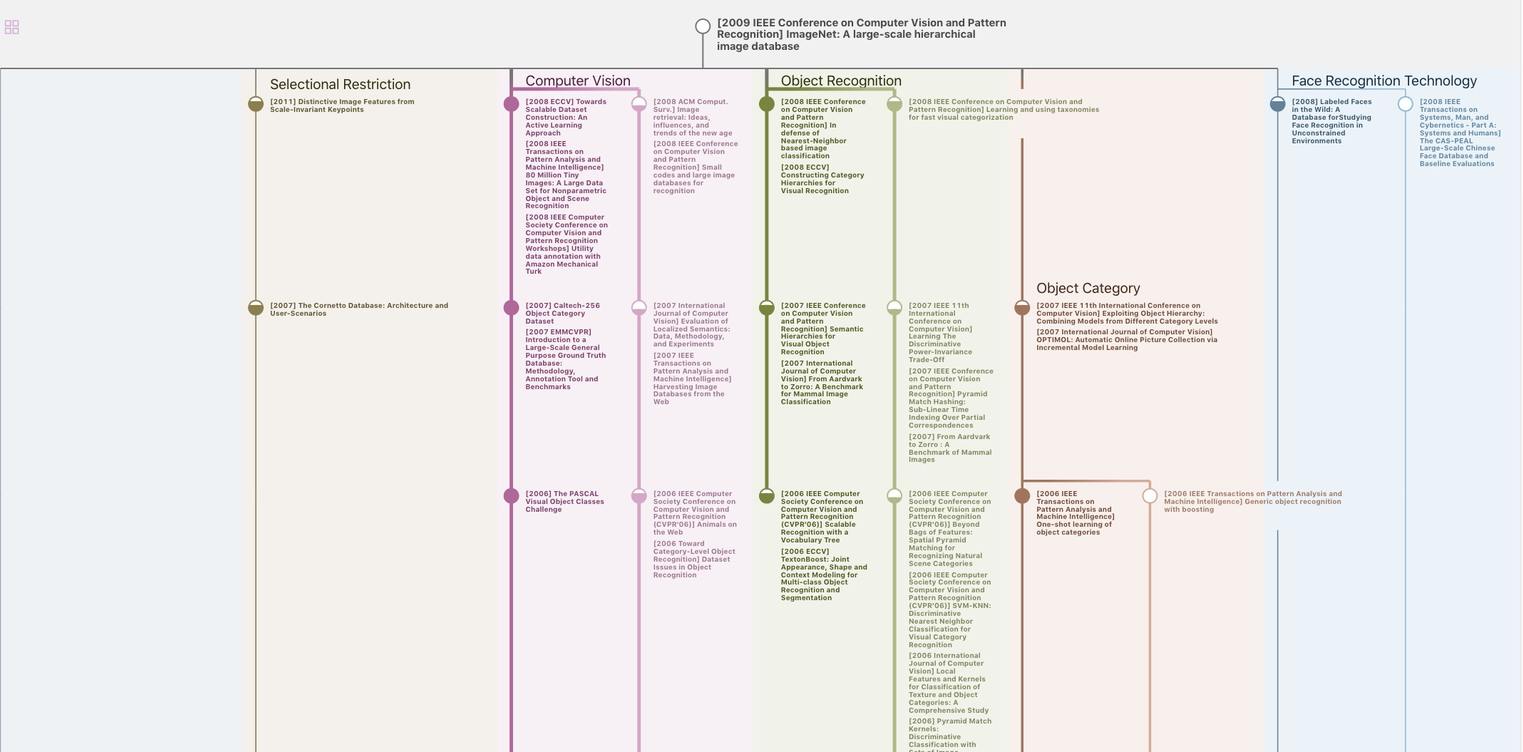
生成溯源树,研究论文发展脉络
Chat Paper
正在生成论文摘要