Multimodal Daily-Life Logging in Free-living Environment Using Non-Visual Egocentric Sensors on a Smartphone.
Proc. ACM Interact. Mob. Wearable Ubiquitous Technol.(2024)
摘要
Egocentric non-intrusive sensing of human activities of daily living (ADL) in free-living environments represents a holy grail in ubiquitous computing. Existing approaches, such as egocentric vision and wearable motion sensors, either can be intrusive or have limitations in capturing non-ambulatory actions. To address these challenges, we propose EgoADL, the first egocentric ADL sensing system that uses an in-pocket smartphone as a multi-modal sensor hub to capture body motion, interactions with the physical environment and daily objects using non-visual sensors (audio, wireless sensing, and motion sensors). We collected a 120-hour multimodal dataset and annotated 20-hour data into 221 ADL, 70 object interactions, and 91 actions. EgoADL proposes multi-modal frame-wise slow-fast encoders to learn the feature representation of multi-sensory data that characterizes the complementary advantages of different modalities and adapt a transformer-based sequence-to-sequence model to decode the time-series sensor signals into a sequence of words that represent ADL. In addition, we introduce a self-supervised learning framework that extracts intrinsic supervisory signals from the multi-modal sensing data to overcome the lack of labeling data and achieve better generalization and extensibility. Our experiments in free-living environments demonstrate that EgoADL can achieve comparable performance with video-based approaches, bringing the vision of ambient intelligence closer to reality.
更多查看译文
关键词
Daily-life logging,Egocentric non-visual sensors,Multi-modal data
AI 理解论文
溯源树
样例
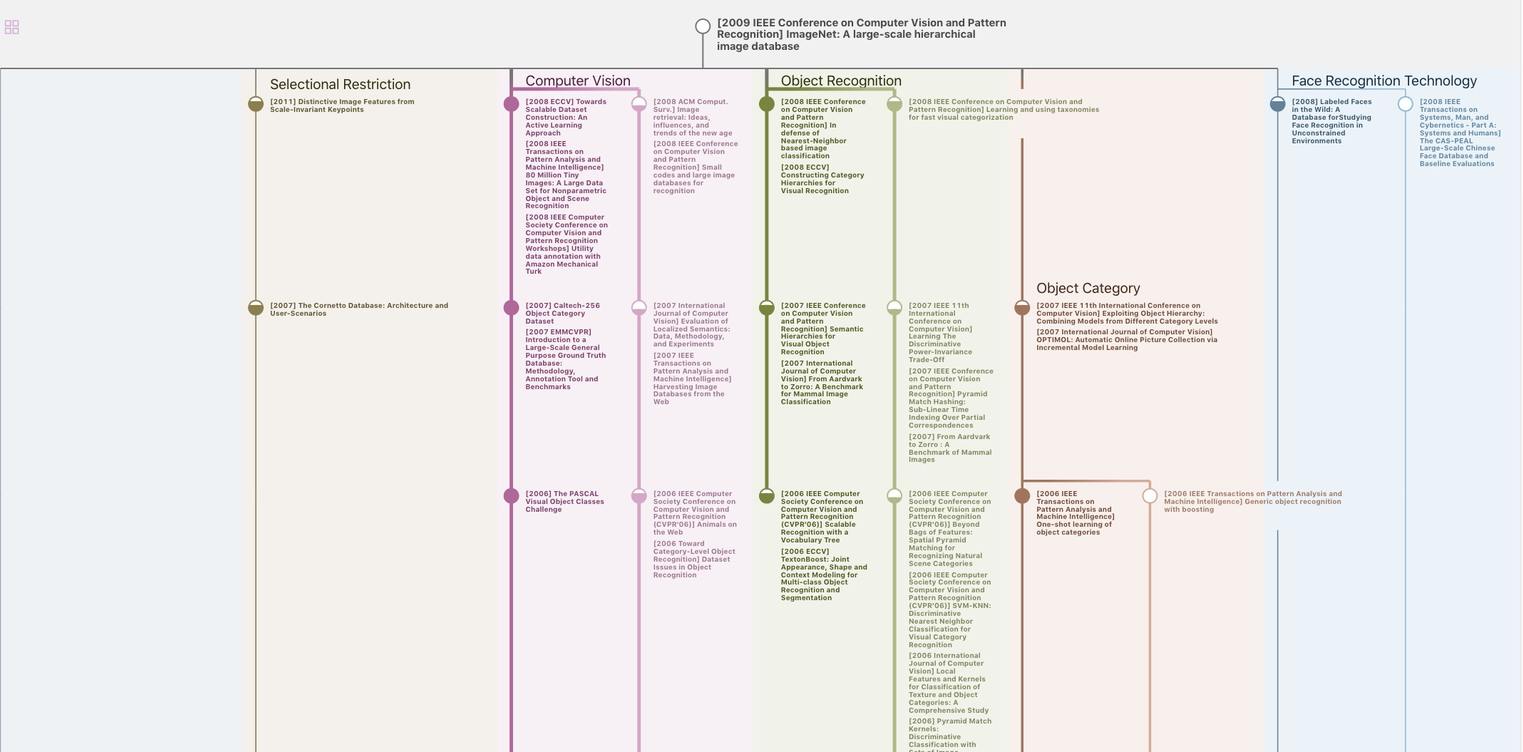
生成溯源树,研究论文发展脉络
Chat Paper
正在生成论文摘要