Learning the dynamics for unknown hyperbolic conservation laws using deep neural networks
SIAM JOURNAL ON SCIENTIFIC COMPUTING(2024)
摘要
We propose a new data -driven method to learn the dynamics of an unknown hyperbolic system of conservation laws using deep neural networks. Inspired by classical methods in numerical conservation laws, we develop a new conservative form network (CFN) in which the network learns to approximate the numerical flux function of the unknown system. Our numerical examples demonstrate that the CFN yields significantly better prediction accuracy than what is obtained using a standard nonconservative form network, even when it is enhanced with constraints to promote conservation. In particular, solutions obtained using the CFN consistently capture the correct shock propagation speed without introducing nonphysical oscillations into the solution. They are furthermore robust to noisy and sparse observation environments.
更多查看译文
关键词
conservation laws,data-driven method,neural networks,conservative form network
AI 理解论文
溯源树
样例
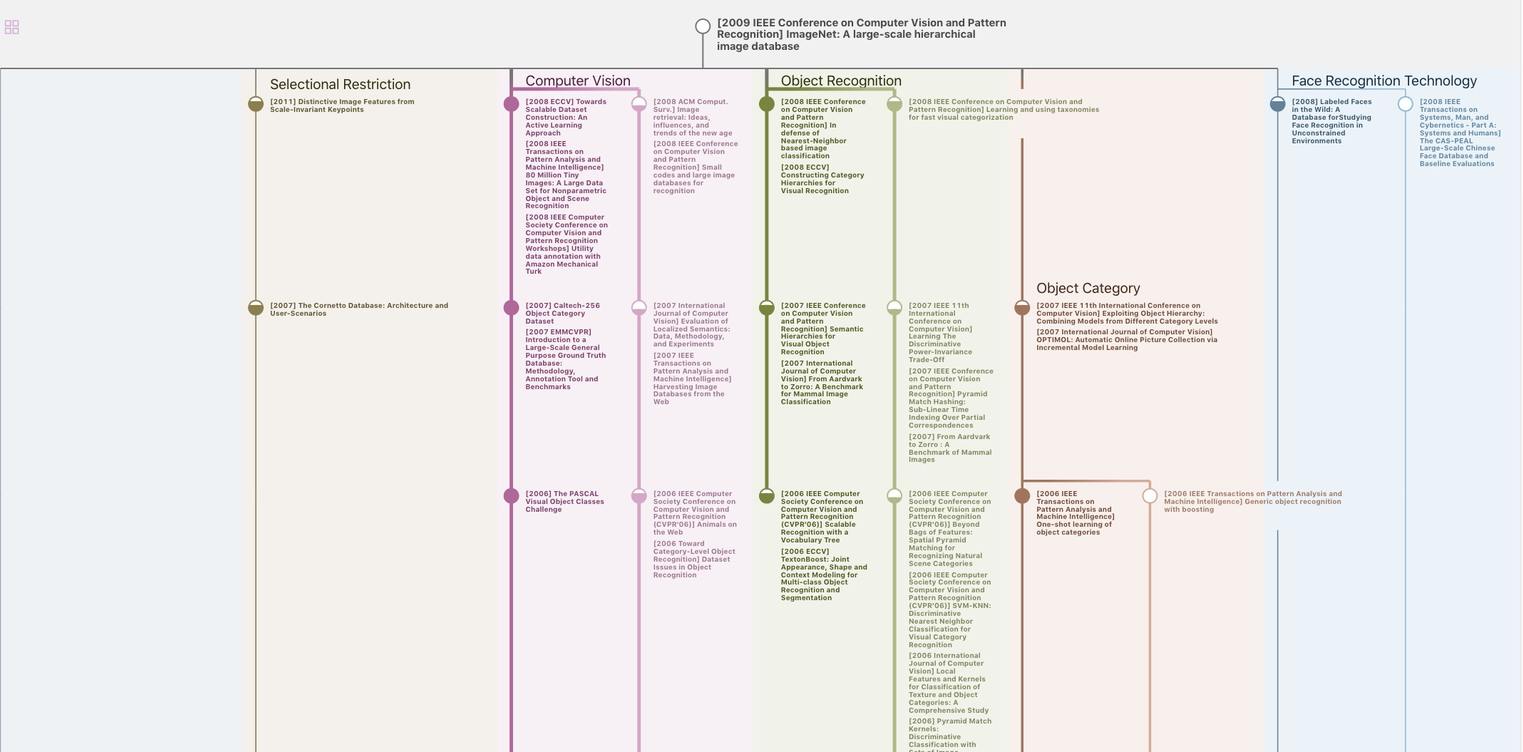
生成溯源树,研究论文发展脉络
Chat Paper
正在生成论文摘要