Correcting missingness in passively-generated mobile data with Multi-Task Gaussian Processes
Transportation Research Part C: Emerging Technologies(2024)
摘要
The prevalence of mobile devices and the ubiquity of network connectivity have generated a massive amount of temporally- and spatially-stamped data. A key characteristic of this mobile data is the prevalence of sparsity—for every recorded user, there is a significant amount of missing data. Sparsity leads to bias in the inferred mobility patterns and thus, correcting bias by imputing the missing data potentially creates opportunities for directly using the corrected mobile trajectory data for large-scale simulations in various applications. We propose a multi-task Gaussian process regression model to correct missingness in mobile data. Gaussian processes (GPs) allow for flexible modeling of diverse (i.e., non-linear, locally periodic) data patterns and quantify prediction uncertainty in an interpretable manner. We develop a methodological framework for applying GPs to mobile data. In doing so, we consider the correlations between users’ coordinates (latitudes and longitudes) through multi-task learning and adjust for individual-level differences in data characteristics through parameter initialization and optimization. We introduce and demonstrate the use of smooth (i.e., rational quadratic) and periodic kernels in modeling human mobility data. Relatedly, we analyze our model’s parameters and imputation accuracy with respect to different types of trips (e.g. slower vs faster trips). We also demonstrate our model’s performance in two experiments with real app-based data, in which it outperforms alternative imputation methods. Our implementation is open-source at https://github.com/ekinugurel/GPSImpute.
更多查看译文
关键词
Mobile data,Human mobility,Travel behavior,Gaussian processes,Multi-task learning,Missing data
AI 理解论文
溯源树
样例
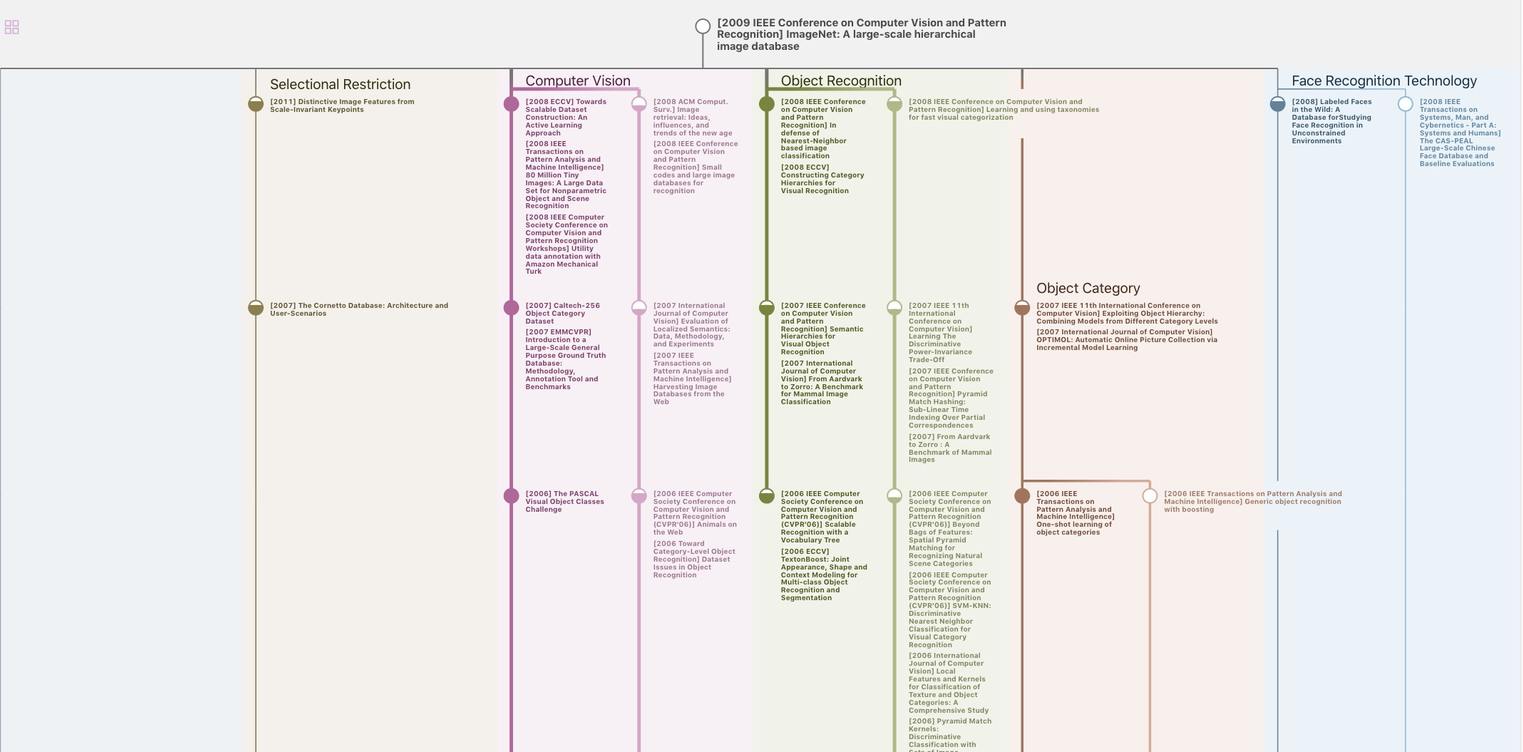
生成溯源树,研究论文发展脉络
Chat Paper
正在生成论文摘要