A Deep Learning Framework for End-to-End Control of Powered Prostheses
IEEE ROBOTICS AND AUTOMATION LETTERS(2024)
摘要
Deep learning offers a potentially powerful alternative to hand-tuned control of active lower-limb prostheses, being capable of generating continuous joint-level assistance end-to-end. This eliminates the need for conventional task classification, state machines and mid-level control equations by collapsing the entire control problem into a deep neural network. In this study, sensor data and conventional commanded torque from an open-source powered knee-ankle prosthesis (OSL) were collected across five locomotion modes: level ground, ramp incline/decline and stair ascent/descent. Reference commanded torques were generated using an expert-tuned finite state machine-based impedance controller for each mode and transfemoral amputee participant (N = 12). Stance phases of the output were then estimated using a temporal convolutional network (TCN), which produced mode- and user-independent knee and ankle torques with RMSE of 0.154 +/- 0.06 and 0.106 +/- 0.06 Nm/kg, respectively. Training the model on mode-specific data only produced significant reductions in stair descent, lowering knee and ankle RMSE by 0.06 +/- 0.028 and 0.033 +/- 0.008 Nm/kg respectively (p < 0.05). Additionally, the TCN adapted to walking speed and slope shifts in reference commanded torque. These results demonstrate that this deep learning model not only removes the need for heuristic state machines and mode classification but can also reduce or remove the need for prosthesis assistance tuning entirely.
更多查看译文
关键词
Mathematical models,Torque,Robot sensing systems,Legged locomotion,Prosthetics,Deep learning,Computer architecture,Prosthesis,end-to-end control,torque estimation,deep learning
AI 理解论文
溯源树
样例
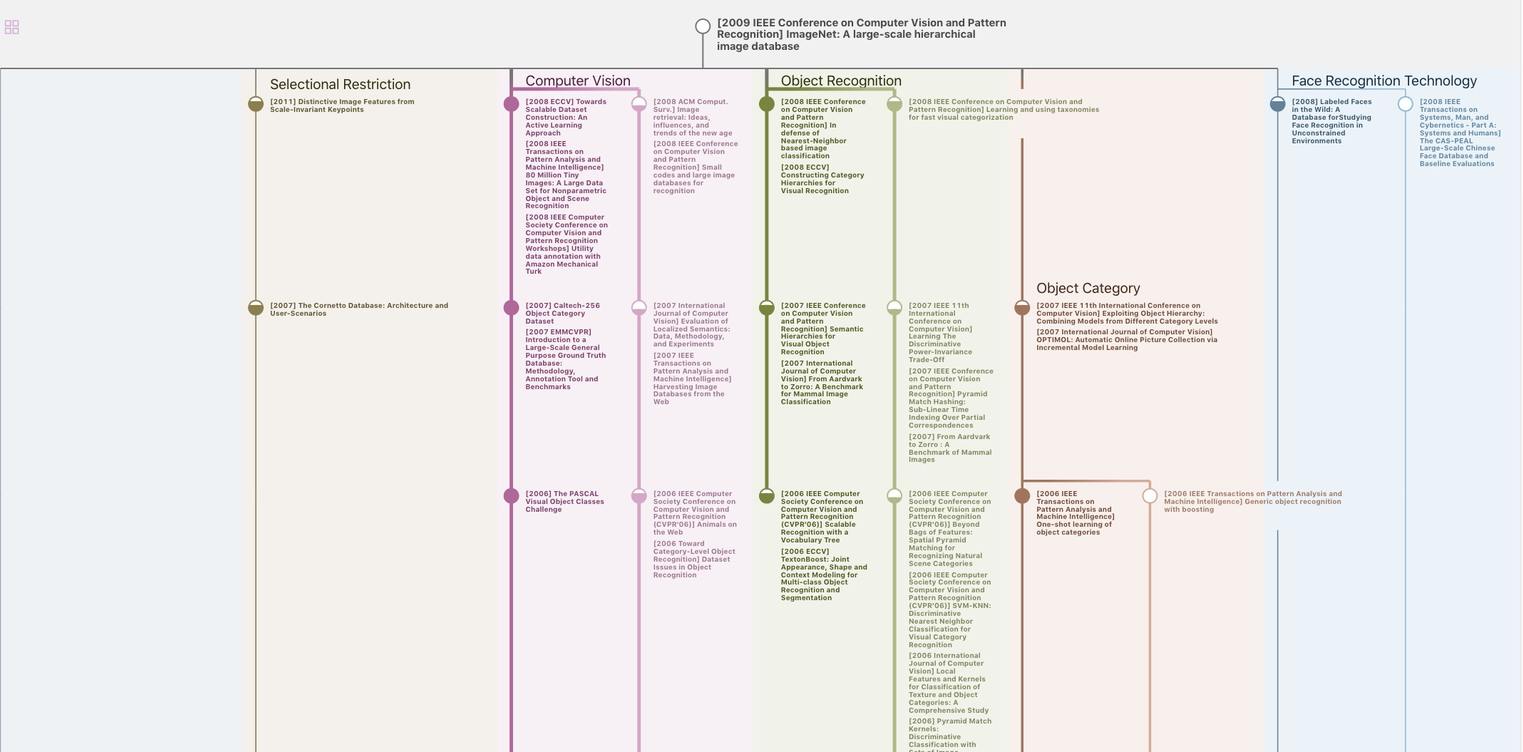
生成溯源树,研究论文发展脉络
Chat Paper
正在生成论文摘要