Dimension-free bounds for sums of dependent matrices and operators with heavy-tailed distributions
ELECTRONIC JOURNAL OF STATISTICS(2024)
摘要
We prove deviation inequalities for sums of high -dimensional random matrices and operators with dependence and heavy tails. Estimation of high -dimensional matrices is a concern for numerous modern applications. However, most results are stated for independent observations. Therefore, it is critical to derive results for dependent and heavy -tailed matrices. In this paper, we derive a dimension -free upper bound on the deviation of the sums. Thus, the bound does not depend explicitly on the dimension of the matrices but rather on their effective rank. Our result generalizes several existing studies on the deviation of sums of matrices. It relies on two techniques: (i) a variational approximation of the dual of moment generating functions, and (ii) robustification through the truncation of the eigenvalues of the matrices. We reveal that our results are applicable to several problems, such as covariance matrix estimation, hidden Markov models, and overparameterized linear regression.
更多查看译文
关键词
Dependent process,heavy-tailed distribution,high-dimension,random matrix
AI 理解论文
溯源树
样例
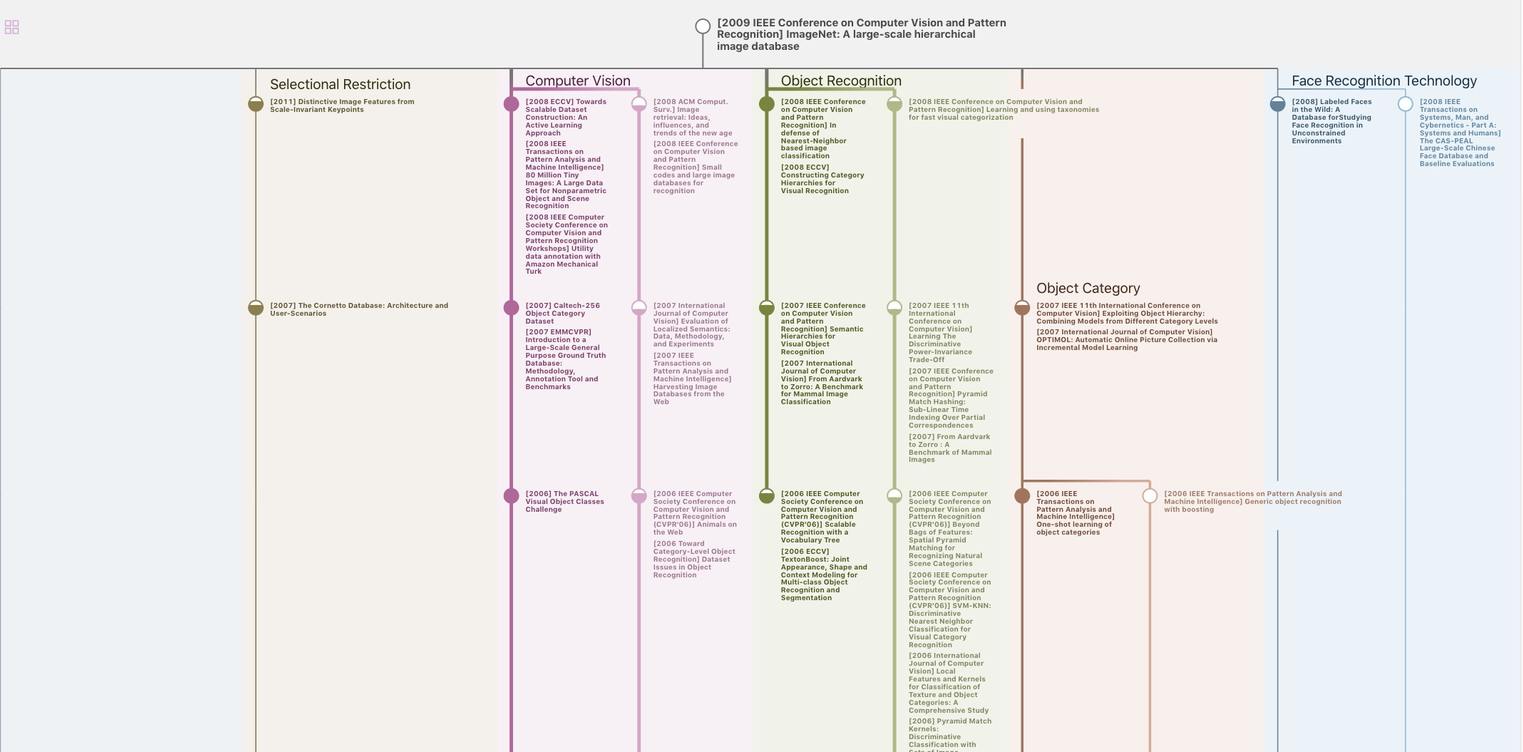
生成溯源树,研究论文发展脉络
Chat Paper
正在生成论文摘要