Deep contrastive representation learning for multi-modal clustering
Neurocomputing(2024)
摘要
Benefiting from the informative expression capability of contrastive representation learning (CRL), recent multi-modal learning studies have achieved promising clustering performance. However, it should be pointed out that the existing multi-modal clustering methods based on CRL fail to simultaneously take the similarity information embedded in inter- and intra-modal levels. In this study, we mainly explore deep multi-modal contrastive representation learning, and present a multi-modal learning network, named trustworthy multi-modal contrastive clustering (TMCC), which incorporates contrastive learning and adaptively reliable sample selection with multi-modal clustering. Specifically, we are concerned with an adaptive filter to learn TMCC via progressing from ‘easy’ to ‘complex’ samples. Based on this, with the highly confident clustering labels, we present a new contrastive loss to learn modal-consensus representation, which takes into account not only the inter-modal similarity but also the intra-modal similarity. Experimental results show that these principles in TMCC consistently help promote clustering performance improvement.
更多查看译文
关键词
Multi-view representation learning,Self-supervision,Clustering
AI 理解论文
溯源树
样例
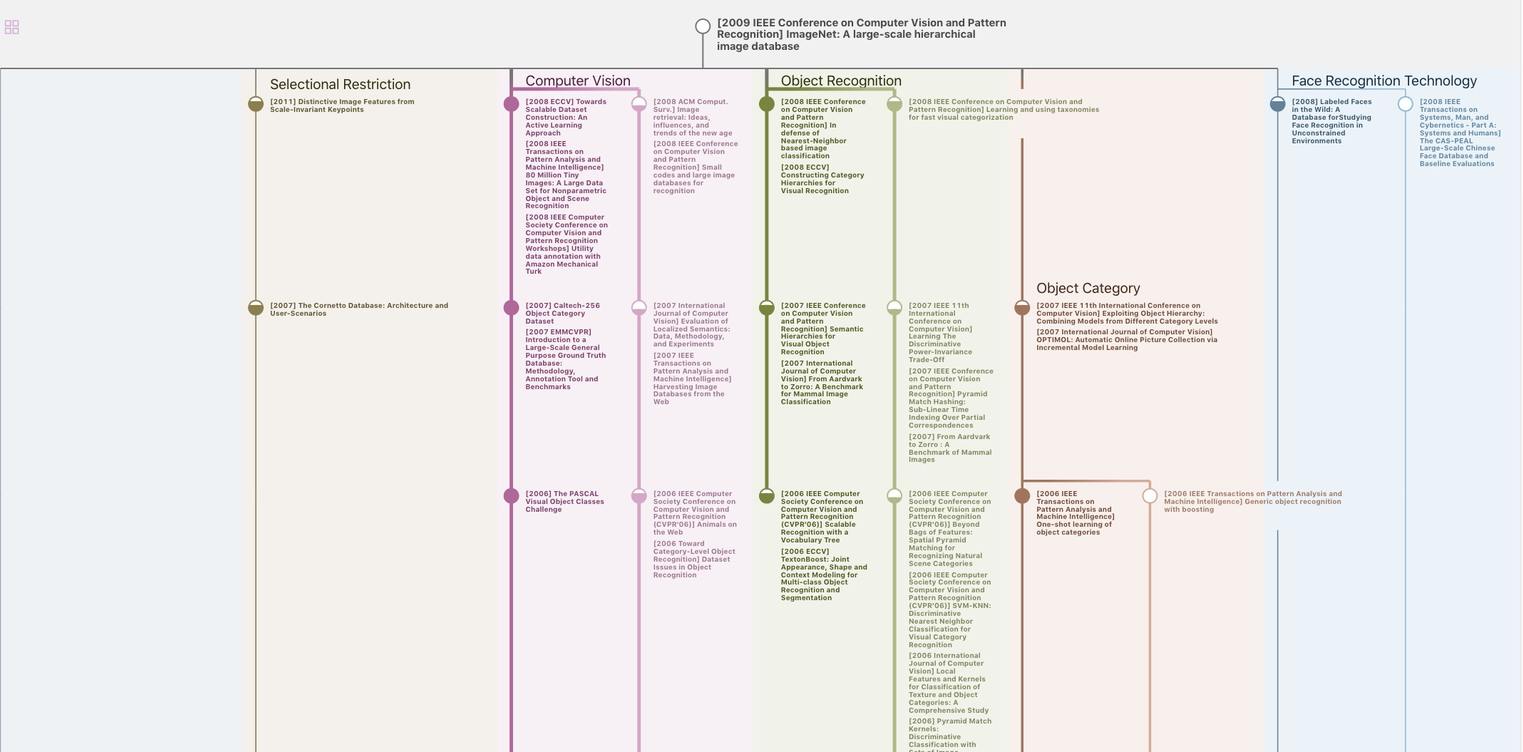
生成溯源树,研究论文发展脉络
Chat Paper
正在生成论文摘要