FAM: Improving columnar vision transformer with feature attention mechanism
Computer Vision and Image Understanding(2024)
Abstract
Vision Transformer has garnered outstanding performance in visual tasks due to its capability for global modeling of image information. However, during the self-attention computation of image tokens, a common issue of attention map homogenization arises, impacting the final performance of the model as attention maps propagate through feature maps layer by layer. In this research, we propose a token-based approach to adjust the output of attention sub-layer, focusing on the feature dimensions, to address the homogenization problem. Furthermore, different network architectures exhibit variations in their approaches to modeling image features. Specifically, Vision Transformers excel at modeling long-range relationships, while convolutional neural networks possess local receptive fields. Therefore, this paper introduces a plug-and-play convolutional operator-based component, integrated into the Vision Transformer, to validate the impact of structural enhancements on model performance. Experimental results on image recognition and adversarial attack tasks respectively demonstrate the effectiveness and robustness of the two proposed methods. Additionally, the analysis of information entropy on the feature mapps of the model’s final layer indicates that the improved model exhibits higher information richness, making it more conducive to the classifier’s discriminative capabilities.
MoreTranslated text
Key words
Vision transformer,Feature adjustment,Network structure improvement
AI Read Science
Must-Reading Tree
Example
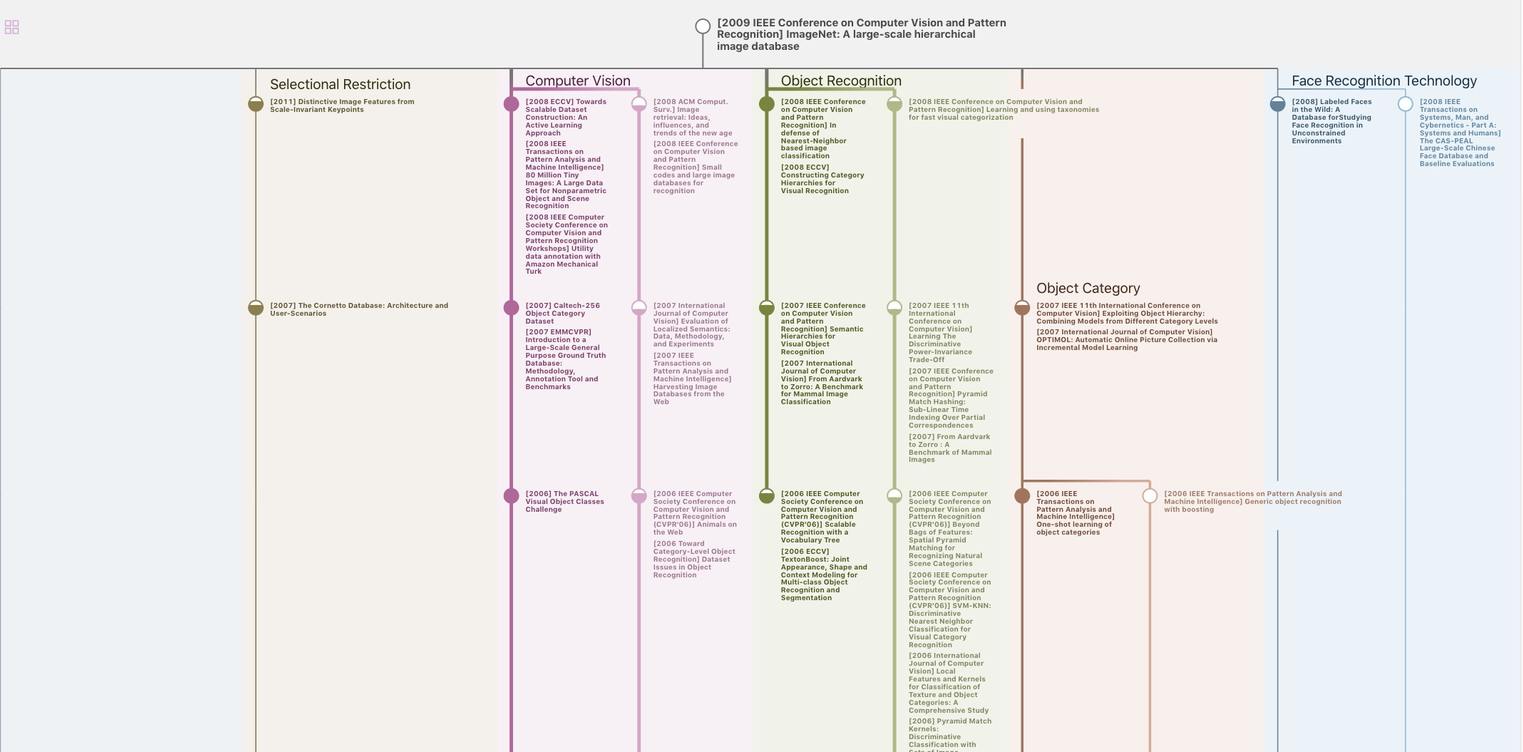
Generate MRT to find the research sequence of this paper
Chat Paper
Summary is being generated by the instructions you defined