Color-based particle-field deep learning computed tomography with impurity-noise suppression
Optics Communications(2024)
摘要
High-quality particle image characterization based on computed tomography is always highly desired in the area of hydrodynamics and relevant applications, as the time-space particle distribution in a flow-field can reflect the field properties well. However, how to eliminate the influence of impurity-in-fluid on the particle-field computed tomography is still a challenge at present. In this paper, we propose a method to address this problem with color-based particle-field deep learning computed tomography. By utilizing color-particles and incorporating a corresponding RGB three-channel spectrum analysis algorithm in the computed tomography, the particle information can be exactly extracted from the impurity background during the image reconstruction. The minor residue of impurity noise and sparse-sampling-induced artifact noise in the tomographic reconstruction are further eliminated by adequate-depth neural network processing. The numerical analysis shows that the particle image quality can be greatly improved from 10.13 dB up to 20.22 dB in PSNR and from 79.42 down to 24.86 in RMSE accordingly. Eventually, the color restoration for the reconstructed particle images are achieved as well. It provides richer object information for high-quality particle-field image reconstruction. The experimental test approves of all theoretical predictions well.
更多查看译文
关键词
Particle-field imaging,Color filtering,Noise suppression,Computed tomography,Deep learning
AI 理解论文
溯源树
样例
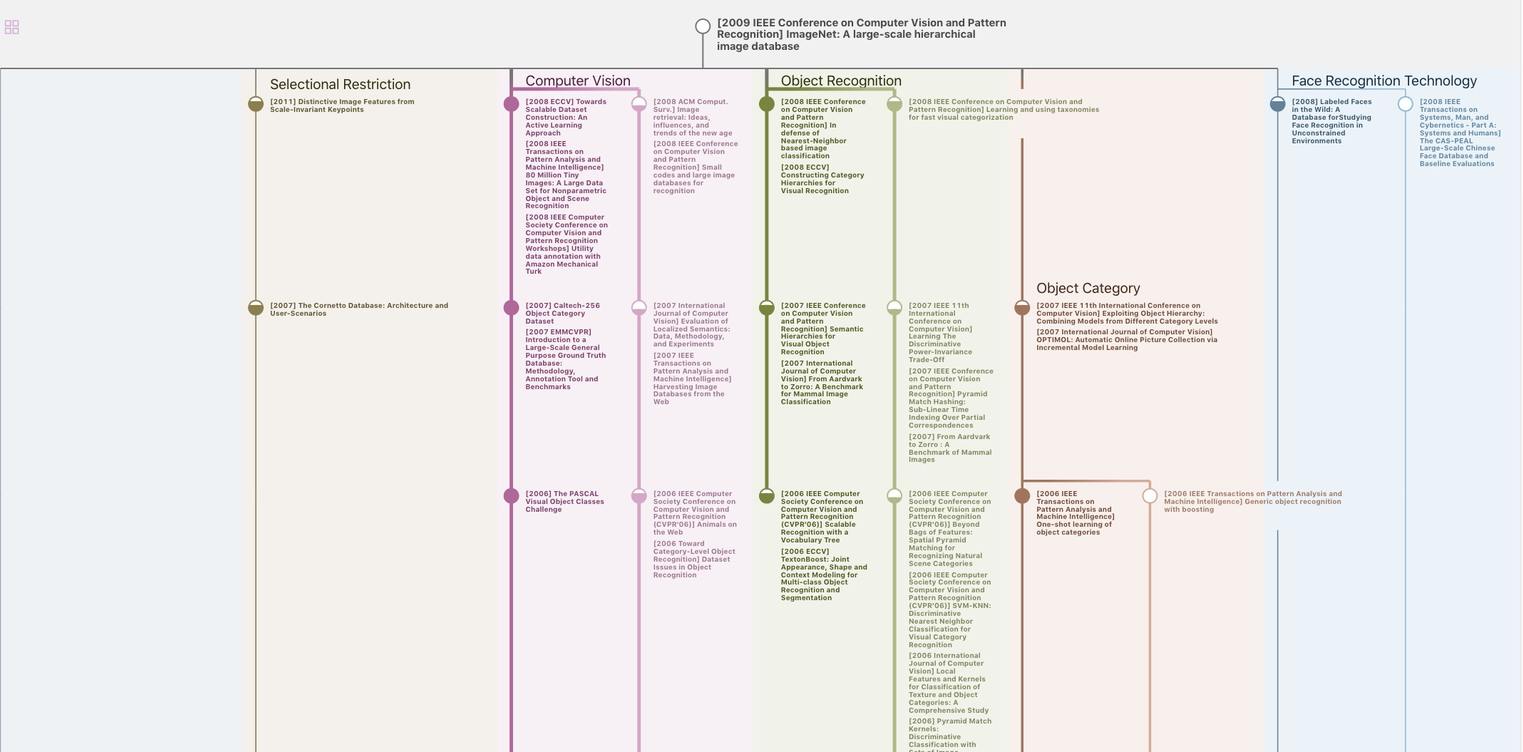
生成溯源树,研究论文发展脉络
Chat Paper
正在生成论文摘要